Argonne National Laboratory Releases Generative AI Framework to Accelerate MOFs Innovation
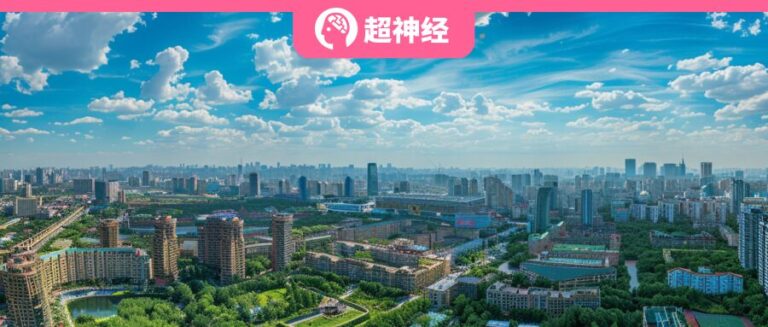
Under the background of rapid industrial development, the earth is facing a serious and urgent problem: excessive carbon dioxide emissions. Carbon dioxide is like an invisible thick cocoon that wraps the homeland on which human beings depend for survival, quietly changing the global climate, and also bringing about a series of chain reactions such as frequent extreme weather, severe damage to the ecosystem, obstruction of agricultural production and public health challenges.
As a cutting-edge means to solve the high carbon dioxide emissions, the innovation and development of carbon capture technology is particularly important and has received more extensive attention. This technology uses physical or chemical methods to extract carbon dioxide from large emission sources and process it to prevent carbon dioxide from directly entering the atmosphere, thereby achieving the purpose of emission reduction.
In recent years, a new type of porous crystalline material called Metal-Organic Frameworks (MOFs) has attracted widespread attention from many researchers due to its unique structure and performance. MOFs are self-assembled by metal ions and organic ligands through coordination bonds. Compared with traditional solid adsorbents such as activated carbon and molecular sieves, they are more environmentally friendly, have lower energy consumption, and show better performance in carbon dioxide adsorption.
However, the current production design of MOFs usually relies on a large amount of experimental data and computational work, which is both expensive and time-consuming.A research team from Argonne National Laboratory in the United States proposed a generative AI framework GHP-MOFsassemble.The framework can randomly generate and assemble new MOFs structures, screen highly stable MOFs structures through molecular dynamics simulations, and use Crystal Graph Convolutional Neural Network (CGCNN) and Grand Canonical Monte Carlo simulations to test the adsorption capacity of MOFs for carbon dioxide. The results show that this method can quickly assemble 120,000 new candidate MOFs within 33 minutes, greatly promoting the future development of MOFs design.
Research highlights:
* Generative AI framework GHP-MOFsassemble rapidly assembles 120,000 new MOF candidates in 33 minutes
* This approach can reduce the high cost of building large databases

Paper address:
https://www.nature.com/articles/s42004-023-01090-2
Download the dataset with one click:
https://bit.ly/3IlrvQl
Follow the official account and reply "MOFs" to get the full PDF
Three major structures form the high-throughput computing framework
The GHP-MOFsassemble method proposed by the researchers is a new type of high-throughput computing framework, which mainly consists of three components: decomposition (Decompose), generation (Generate), screening and prediction (Screen and Predict).
Decompose: Decomposing hMOFs datasets using MMPA
The hMOFs selected for the study is a high-performance metal-organic framework (HMF) related data set, which contains the structural information of high-performance MOFs that have been screened or experimentally verified.

Carbon is grey, oxygen is red, nitrogen is blue, and hydrogen is white
In the hMOFs dataset, three types of topological structures frequently appear: Cu PW-pcu, Zn PW-pcu and Zn TM-pcu. These three types of structures account for 74% of the total dataset, a total of 102,117 hMOF structures. The researchers selected 78,238 MOF structural data with correctly resolved MOFids and valid SMILES structures.
SMILES: Simplified molecular input line entry system, simplified molecular linear input specification

The researchers extracted 540 molecular fragments from high-performance hMOF structures (the last column in the table above), decomposed the 540 unique structures using the matching molecular pair algorithm (MMPA), and extracted their molecular fragments. New MOFs connectors were generated using DiffLinker.
Generate: Diffusion model to generate new MOFs linkers

Carbon is grey, oxygen is red, nitrogen is blue, and hydrogen is white
For the generation part of the GHP-MOFassemble framework, the researchers first extracted the molecular fragments of the above 540 unique structures.New MOFs linkers were generated using the diffusion model DiffLinker.
In this process, the researchers used openbabel to add hydrogen atoms and delete connectors with incorrect hydrogen assignments, perform a virtual atom recognition process, generate information that can be assembled with metal nodes, and then process it through component filters, ultimately obtaining 12,305 connectors.Three linkers generated by DiffLinker and a metal node that frequently appears in the hMOFs dataset were randomly selected for assembly.
It is reported that researchers generated 120,000 new MOFs with PCU topology in 33 minutes on the Theta supercomputer at Argonne National Laboratory (ALCF).
Screen and Predict: CGCNN model tests carbon dioxide adsorption capacity
The researchers performed a geometric structure check on the new MOFs structures and identified 78,796 MOFs with effective bond lengths within 40 minutes. A pre-simulation check was then performed, and 18,770 MOFs were successfully generated for LAMMPS input files in less than 4 hours (205 minutes). In order to reduce the number of LAMMPS simulations, the researchers trained a CGCNN model to screen the adsorption properties of MOFs.
LAMMPS: Large-scale Atomic/Molecular Massively Parallel Simulator
CGCNN model training process: The MOFs structures in the hMOF dataset and their carbon dioxide adsorption at 0.1 bar were used as input data. The hMOF dataset was divided into three independent datasets: 80% for training, 10% for validation, and 10% for testing. With this data split, three CGCNN models were trained using the random weight initialization method.
By training based on the CGCNN model on the NVIDIA A40 GPU, the researchers predicted the carbon dioxide adsorption capacity of the 18,770 screened MOFs structures mentioned above, and performed molecular dynamics simulation on 364 MOFs structures with high adsorption performance. The entire AI reasoning process was completed within 50 minutes.
In summary, based on the GHP-MOFsassemble framework, the researchers completed the entire process of generating new MOFs structures and screening high-performance structures within 5 hours and 7 minutes.

Carbon dioxide adsorption capacity of 6 MOFs at 0.1 bar and 300 K
Finally, the researchers identified 102 stable and high-performance MOFs structures and performed Grand Canonical Monte Carlo (GCMC) simulations on these 102 MOFs.By calculating their carbon dioxide adsorption capacity at 0.1 bar and 300K, six MOFs with carbon dioxide adsorption capacity higher than 2 m mol g−1 were found. The crystal structures of these six MOFs are as follows:

Carbon is grey, nitrogen is dark blue, fluorine is cyan, zinc is purple, hydrogen is white, and lithium is green
Experimental conclusion: AI framework can generate effective new MOFs

The researchers conducted a similarity analysis between the ligands generated based on the AI framework and the hMOFs ligands and found that the similarity between the ligands generated by AI and the ligands in hMOFs was only 30%-40%.This shows that the AI framework is able to generate new ligands.
The high similarity peak at 0.4 also indicates that AI can generate ligands similar to those already present in hMOFs.This shows that the MOFs generated under this AI framework are practically effective.
It is worth mentioning that although GHP-MOFassemble can successfully discover and verify metal-organic framework structures with excellent properties, it is not perfect.The screening rules, simulation conditions and model accuracy of the experimental process will affect the accuracy of the results. The performance of MOFs screened by this AI framework also needs to be further verified in experiments.
AI goes deep into carbon capture, Nvidia's simulation speed increases 700,000 times
Today, addressing climate change has become a global challenge that concerns the common future of all mankind. The Chinese government attaches great importance to this and has set a clear emission reduction path: it plans to peak carbon dioxide emissions by 2030 and strive to achieve carbon neutrality by 2060. When the visions of "carbon peak" and "carbon neutrality" collide with AI digital technology, a series of future changes will be born.In fact, artificial intelligence has been looking for solutions for low carbon.

Source: Baidu Smart Cloud
Microsoft has stated thatAI technology can use large amounts of data from different sources to solve complex problems, thereby helping to improve global productivity and play a role in reducing carbon dioxide and other greenhouse gas emissions. In addition, Jin Xuan, a professor at the University of Surrey, has also shown in his research that with the help of AI models, it is possible to reduce the energy consumption of carbon capture facilities in coal-fired power plants by more than one-third and increase the carbon dioxide capture efficiency by at least 16.7%.
In addition, NVIDIA also released Earth-2, a full-stack open platform that can accelerate climate and weather forecasts through interactive high-resolution simulations. At the same time, NVIDIA uses the Riebel neural operator and neural network framework NVIDIA Modulus to increase the simulation analysis speed of carbon capture and storage by 700,000 times, completing the reliability assessment of carbon dioxide plumes and pressure accumulation in 2.8 seconds. This improvement undoubtedly provides technical support for subsequent carbon capture research.
Faced with increasingly severe environmental pressures and ecological warnings, promoting breakthroughs and applications of carbon capture technology is no longer just a scientific proposition, but also an issue of the times concerning the sustainable development of mankind.Integrating AI technology into the carbon capture process, especially in the design of new materials such as metal organic frameworks (MOFs), is expected to bring about a new round of scientific and technological breakthroughs and help promote the transformation of the global economic and social system towards a greener and low-carbon direction.
References:
1. https: www.ambchina.com
2.https:www.nvidia.cn/high-performance-computing/earth-2/
3.http://www.tanpaifang.com/CCUS/202307/1198593.html
4.http://m.xinhuanet.com/tech/2021-04/01/c_1127280796.htm