Beware of Urban "chronic Diseases": Professor Liu Jianxin's Team From Central South University Uses AI to Predict the Risk of Land Subsidence in the Next 40 Years
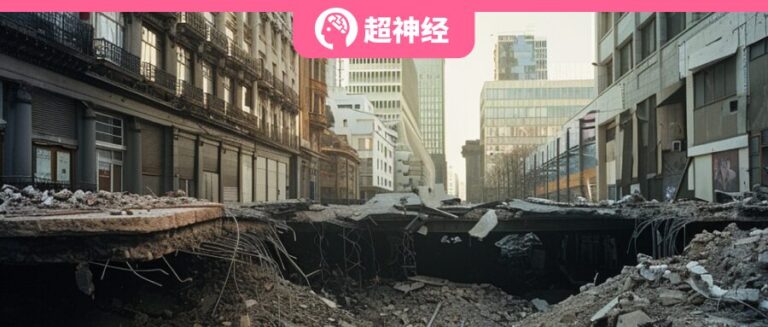
In May 2023, the owners of Fengjin Courtyard in Balitai Town, Jinnan District, Tianjin were "terrified" because, from a distance, their 25-story building "seemed to be crooked." What made the owners even more panic was that not only did the grass and roads around the building begin to sink and crack, but even the foundation of the building had dozens of centimeters of settlement and cracks, and some underground parking lots also had cracks and water seepage in the walls, causing thousands of owners to be unable to return home.
In fact, land subsidence (LS) is not uncommon. As early as 2012, the first National Land Subsidence Prevention and Control Plan (2011-2020) was jointly compiled by the Ministry of Land and Resources, the Ministry of Water Resources, the National Development and Reform Commission, the Ministry of Finance and other ten ministries and commissions. It pointed out that there are more than 50 cities in the country suffering from land subsidence disasters, distributed in 20 provinces, autonomous regions and municipalities including Beijing, Tianjin, Hebei, Shanxi and Inner Mongolia.
In addition, a study published in Science in 2021 by the UNESCO Land Subsidence Working Group also warned that by 2040, land subsidence will threaten nearly 1/5 of the world's population. It can be seen that how to achieve rapid monitoring and accurate simulation of land subsidence has become the primary problem in current urban development.
In order to solve this problem, the team of Professor Liu Jianxin from the School of Earth Sciences and Information Physics of Central South University, in collaboration with the Guangdong Provincial Geological Environment Monitoring Station, the Fourth Geological Brigade of Guangdong Province, and the University of Boigny in Côte d'Ivoire, published the research results of intelligent prediction of urban land subsidence in the international academic journal of environmental science and ecology, Journal of Environmental Management.The first to use extreme gradient boosting regressor (XGBR) and long short-term memory (LSTM) network for land subsidence modeling in urban areas,It not only solves the inherent limitations of single model frameworks in dealing with complex scenarios, but also provides effective prediction methods for disaster prevention, mitigation and land use strategies through data-driven strategies.
Research highlights
* Using Taylor plots, we demonstrate that the XGBR and LSTM models have strong correlation with the sedimentation data, as well as prediction accuracy
* Use InSAR, geological and borehole data to estimate the rate of change of surface activity, thereby revealing the key variables that affect surface activity
* Promote proactive land use management through data-driven strategies, laying the foundation for sustainable urban development and resource management practices

Paper address:
https://doi.org/10.1016/j.jenvman.2024.120078
Follow the official account and reply "ground subsidence" to get the complete PDF
Research scope: Focus on Nansha District and explore the land subsidence risks in the Pearl River Delta region
The subsidence area in the Pearl River Delta region has reached 11,397 square kilometers, and the subsidence amplitude generally exceeds 500 mm, and the maximum can reach more than 1,000 mm. Among them, Nansha District is located at the southernmost tip of Guangzhou City, which is the intersection of Xijiang River, Beijiang River and Dongjiang River, with a total area of about 803 square kilometers. From the perspective of lithology, Nansha District is mainly distributed with soft soil deposits such as brown-red clay, light yellow gravel, medium-coarse sand, porphyritic clay, gray-white, and brown-red gravel. From the perspective of hydrogeological conditions, the groundwater in Nansha District can be divided into two major aquifers: unconfined and confined.
With the rapid development of the Pearl River Delta region, Nansha District has not only been limited to surface development during the rapid urban development, but has also increased the collection of groundwater, which has led to a drop in groundwater levels. At the same time, the increase in high-rise buildings and industrial facilities has further increased the pressure on the surface, exacerbating the occurrence of ground subsidence.
Geotechnical surveys, including engineering and hydrogeological drilling, show that ground subsidence in Nansha District has gone through four different stages: the start-up stage (2015-2016, -125mm), the development stage (2016-2018, -170mm), the expansion stage (2018-2020, -222mm), and the rapid development stage (2020-2022, -320mm), which are basically consistent with urban development and infrastructure construction.

Dataset: SAR and geological data are combined to fully restore local geological information
The SAR (Synthetic Aperture Radar) image data for this study was acquired from the Sentinel-1 satellite of the European Space Agency's Copernicus mission (GMES).
This is an active microwave remote sensing satellite, consisting of two satellites, Sentinel-1A and Sentinel-1B, which carry sensors that can provide continuous images during the day and night and in all weather conditions. From June 15, 2015 to December 23, 2022, Sentinel-1 carried a C-band synthetic aperture radar and captured a total of 213 scenes. The researchers cross-checked the SAR data with actual data collected through control points every year.

In order to better understand the comprehensive geological and drilling data of Nansha District, the study also analyzed the local soil characteristics, groundwater levels, etc.:
* Geological information:By collecting geological system information from the Guangdong Provincial Geological Bureau, the Nansha area can be divided into eight geological periods: Tertiary, Cretaceous, Jurassic, Triassic, Permian, Carboniferous, Devonian and Silurian.
* Fill thickness (FSt) and sand layer distribution (SLd):Different soils will lead to different settlements. This study effectively evaluates the underground ground conditions in the Nansha area by carefully collecting data on fill thickness (FSt) and sand layer distribution (SLd) from engineering boreholes at different times.
* Building density (BC):The building density (BC) of Nansha District is derived by analyzing large buildings from the Guangdong Provincial Geological Bureau from 2015 to 2022 and based on comprehensive civil engineering data of building structures, thus deeply exploring the relationship between urban infrastructure density and ground subsidence in this densely populated area.
* Groundwater Level (GWL):The study also obtained data on the presence, depth, quantity and quality of groundwater in Nansha District through hydrogeological drilling, based on a groundwater level map compiled from the combined results of all drillings in Guangdong Province.

Research method: Integrating InSAR and machine learning, using the Δr strategy to fit variables
The study is structured as a brief overview of SAR data processing steps and integration techniques based on machine learning.
Due to the influence of atmospheric delay, orbital residual and decorrelation noise, the Interferometric Synthetic Aperture Radar (InSAR) technology can only measure surface deformation within two imaging cycles and cannot measure deformation that changes over time. In order to reduce the influence of atmospheric delay, the current mainstream time series InSAR methods can be divided into permanent scatterer interferometry (PS-InSAR) and small baseline subset method (SBAS-InSAR). PS-InSAR technology has largely overcome the influence of factors such as decorrelation noise. SBAS-InSAR technology can maximize the use of existing SAR data for high-speed and accurate surface deformation inversion.
The machine learning model of this study is composed of extreme gradient boosting regression (XGBR) and long short-term memory network (LSTM).
Among them, XGBR is a variant of the gradient boosting algorithm for regression tasks, which can generate diverse training data sets through a random sampling process; the LSTM architecture is designed to address the limitations of traditional recurrent neural networks in capturing and learning long-term relationships, and can effectively manage and retain information over the entire time interval.
Based on the above method,The study first obtained the main dataset by analyzing SAR imagery.From June 2015 to December 2022, the Sentinel-1 satellite collected a total of 225 scenes as SAR images. Secondly, the researchers used the PS-InSAR technology to process 144 images obtained between June 2015 and November 2019, of which the images taken in December 2018 were used as the main images; then, the researchers used the SBAS-InSAR technology to process 66 images captured between January 2019 and December 2022. Finally, using the PS-InSAR and SBAS-InSAR monitoring results, the cumulative settlement data from June 2015 to November 2019 were used as the deformation sequence benchmark, and the cumulative deformation variables from June 2015 to December 2022 were time series fused.
Next,This study focuses on extracting groundwater level (GWL) data.This is a key step in understanding the impact of groundwater dynamics on surface morphology. The study mapped the aquifers based on hydrogeological drilling conducted throughout Guangdong Province from June 2015 to December 2018. The map was drawn based on information collected from each borehole in both aquifers. The hydrogeological data was then analyzed using Golden Software Surfer 25.1 and rasterized using ArcGIS Pro 1.2.
at last,The study explored in depth the delta rate (Δr) strategy using advanced machine learning algorithms to interpret and further analyze the data.In order to fit all variables on the same time scale, the study used delta rate (Δr) calculation and applied it to all data except the classification feature Lith. Two different techniques were applied to LSTM and XGBR to solve these problems. In LSTM, the study directly implemented Lasso regression (L1 regularization) in the LSTM network, adding a penalty term equivalent to the absolute value of the coefficient to the loss function Las to reduce the influence of specific features. In XGBR, the study can indirectly affect the importance of features by limiting the complexity of the model by controlling the depth of the tree.
Research results: XGBR is better than LSTM model
By analyzing the spatial and temporal patterns of Nansha District from 2015 to 2022, this study calculated the ground subsidence or deformation in Nansha District in different time periods.
* June - December 2015:There is no deformation signal in Nansha District, the maximum accumulated settlement is -21 mm, and the land and buildings are very stable.
* 2015-2016:Large deformations occurred around many streets in Nansha District, with the maximum cumulative settlement reaching -64 mm.
* 2015-2017:The deformation in the southeast of Nansha District is the most serious, with the maximum cumulative settlement of -128 mm.
* 2015-2018:The maximum cumulative settlement in Nansha District is -176 mm.
* 2015-2019:The maximum cumulative settlement in Nansha District is -225 mm.
* 2015 - 2020:The western side of Nansha District has undergone significant deformation, with the maximum total settlement being -263 mm.
* 2015 - 2021:Many communities have experienced widespread deformation signals, with the highest cumulative settlement reaching -320 mm.
* 2015 - 2022:The maximum accumulated settlement is -364 mm.

This study evaluated the importance of different features by using XGBR and Random Forest (RF). Both models showed thatGroundwater level and building density are the factors that have the greatest impact on land subsidence, and their cumulative importance is around 51%.Next, the study established a land subsidence prediction model using the average Δr and attempted to solve the subsidence problem by reducing the groundwater level and building density by 80%.
The results show thatLowering the groundwater level and building density can significantly reduce the land subsidence rate.In addition, there may be subtle differences in the prediction results of XGBR and LSTM models. In fact, XGBR can better capture sudden changes, while LSTM can better capture long-term dependencies.

The study further used XGBR and LSTM to simulate and predict subsidence data until 2060. The results showed that:
* By 2024,The XGBR predicted settlement was relatively small, with most areas less than −250 mm;
* By 2030,The accumulation of land subsidence became more obvious and gradually worsened thereafter;
* By 2060,The settlement data in some areas will exceed -450mm.
Overall, the prediction trend of LSTM is consistent with that of XGBR, but the severity is smaller overall.

LS simulations for 2024, 2030, 2040 and 2060
In the testing phase of this study, RMSE, MAE, and R² were used to evaluate the performance of the model based on the normalized groundwater level.
The results show that the predicted values of XGBR are very close to the actual values and can capture more variability. In addition, the average prediction error of LSTM is larger than that of XGBR, and the average prediction accuracy is lower. At the same time, the Taylor diagram also shows thatBoth models have high correlation coefficients with the observed data, but the XGBR model's correlation and standard deviation with land subsidence are closer to agreement.

In addition, the study also shows that as the risk of ground subsidence caused by groundwater levels and building density is effectively reduced, the degree of future surface subsidence can be effectively controlled.If no action is taken, the risk of land subsidence by 2040 may be more serious than predicted by the study.
Land subsidence: a geological disaster phenomenon that occurs all over the country
Although ground subsidence has received high attention from researchers including Professor Liu Jianxin, research on artificial intelligence in related fields is still in its early stages and urgently needs more efforts.
The "Comprehensive Study on Land Subsidence Investigation and Monitoring in the North China Plain" and "China Groundwater Resources and Environmental Survey" published by the China Geological Survey show that the subsidence centers in different areas of the North China Plain have a tendency to be connected; the area of the Yangtze River region with a cumulative subsidence of more than 200 mm in the past 30 years is nearly 10,000 square kilometers, accounting for 1/3 of the total area of the region. Among them, Shanghai, Suzhou, Wuxi and Changzhou in Jiangsu Province have begun to experience geological disasters such as ground fissures. Not only that, more places including Northwest China and South China are in the long-term trouble of slow subsidence.
Groundwater overdraft can have a variety of adverse effects, including land subsidence and permanent groundwater storage loss. In October 2023, the latest research published in the journal Nature Communications showed that about 17 cubic kilometers of groundwater storage capacity disappears every year worldwide, leading to land subsidence and permanent reduction of groundwater reserves. Researchers predict that the United States, China, and Iran are the countries with the most serious groundwater reserve losses and will also be the "hardest hit areas" of future ground subsidence.
Today, by leveraging machine learning and comprehensive data sets, as well as machine learning methods based on random forest algorithms, researchers are able to gain a deeper understanding of the environmental challenges posed by over-extraction of groundwater, and emphasize the need to take proactive measures to protect water resources and mitigate the impact of land subsidence. However, as in this study, artificial intelligence predictions only provide a guiding direction. Whether the groundwater crisis and ground building density in the region can be truly alleviated is the fundamental solution.