A Research Team From Beijing Normal University Established the ECA-Net Model to Predict China's Wind Energy Utilization Potential in the Next 70 Years
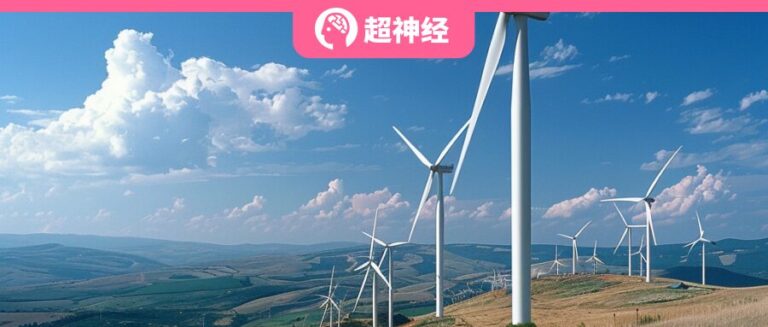
From the increased frequency of extreme climate events such as heavy rains, floods, droughts, hurricanes, and forest fires, to the rising sea levels caused by the melting of polar glaciers, to the loss of biodiversity and the increased pressure on water resources, global warming has truly affected everyone on the planet.
Expanding the deployment of renewable energy is an effective way to mitigate global warming. China has pledged to achieve "carbon peak" in 2030 and "carbon neutrality" in 2060. To achieve this goal, my country plans to vigorously promote the adoption and development of renewable energy, and clean and sustainable wind energy is one of the most valued resources. However, one disadvantage of wind energy resources is that it relies on meteorological drive and is intermittent. Especially in the context of climate change, this disadvantage may be further amplified.
In response to this, many studies have begun to explore how to detect the impact of climate change on regional wind energy. Under the same climate scenario, different models may produce different wind speed change predictions, and the same model may also produce different wind speed change predictions under different scenarios.This inter-model uncertainty and scenario uncertainty are major challenges in assessing changes in wind power generation potential under climate change.
Recently, a research team from the School of Environment at Beijing Normal University published a new paper titled "Assessing Climate Change Impacts on Wind Energy Resources over China Based on CMIP6 Multimodel Ensemble" in ACS Publications. The study assessed how wind energy potential will change under the background of global warming.
Compared with most previous studies that used only one or a few climate models to predict regional wind energy potential,The study used 22 CMIP6 Global Climate Models (GCMs) as output to provide a reliable assessment of uncertainties between models.In addition, improving wind speed simulation can effectively reduce inter-model uncertainty, so this study also uses a deep convolutional neural network (CNN) combined with an efficient channel attention (ECA) module (ECA-Net) to generate reliable prediction results. The relevant evaluation results are of great significance for the medium- and long-term planning of wind power generation in my country and the formulation of policies related to carbon emission reduction.
Research highlights:
* The study compared my country’s wind power generation potential under low, medium and high warming scenarios to better account for environmental uncertainties
* This study used 22 large-scale predictors from CMIP6 GCM outputs to generate 22 sets of simulation data to provide a robust assessment of inter-model uncertainty
* The findings of this study are of great significance to my country's medium- and long-term planning of wind power generation and the formulation or implementation of policies related to carbon emission reduction.

Paper address:
https://pubs.acs.org/doi/abs/10.1021/acs.estlett.3c00829
Follow the official account and reply "wind energy" to get the complete PDF
Dataset: Multiple evaluations based on historical data
This study established the ECA-Net downscaling model using monthly large-scale prediction factors from the European Centre for Medium-Range Weather Forecasts (ECMWF) global climate reanalysis dataset (ERA5) and monthly wind speed data observed from 1981 to 2005.
Among them, large-scale prediction factors include eastward near-surface wind speed (m/s), northward near-surface wind speed (m/s), near-surface wind speed (m/s), near-surface temperature (K), sea level pressure (Pa), surface downward shortwave radiation (W/m²), surface upward sensible heat flux (W/m²) and 200 hPa air temperature (K). Monthly wind speed data are from the China National Station Basic Element Dataset V3.0 of the National Meteorological Information Center of the China Meteorological Administration.
Dataset address: http://data.cma.cn/en
The following table lists the details of the dataset used in this study:

Model architecture: forecasts based on three emission scenarios
This study established the ECA-Net downscaling model based on the ECA-Net method and validated the established model using data from 2006 to 2010. Then, using the validated model, the future wind speed under various scenarios was projected through the monthly large-scale prediction factors provided by GCM, and the wind energy potential was calculated based on the wind energy density function.

In the figure above, the light pink box represents the training data, the light green box represents the validation data, the yellow box represents the GCM data, the light blue box represents the ECA-Net downscaling model, the orange box represents the downscaled wind speed, the gray box represents the wind energy potential calculation, and the green box represents the analysis of the change of wind energy potential under climate change.
Future climate projections involve emission scenarios under three warming patterns, namely SSP1-2.6, SSP2-4.5 and SSP5-8.5. SSP1-2.6 represents lower greenhouse gas emission levels and relatively small climate change, indicating that the temperature rise will not exceed 2°C by 2100, SSP2-4.5 refers to moderate greenhouse gas emission scenarios, and SSP5-8.5 refers to high and very high greenhouse gas emission scenarios.
In order to construct the ensemble wind speed projection of GCM data, this study first input the large-scale prediction factors output by each CMIP6 GCM into the ECA-Net model to generate a total of 22 sets of wind speed simulation data.Then, the ensemble mean is calculated based on the obtained simulation data.Finally, spatial and temporal variability analysis, K-means cluster analysis, and hotspot analysis are performed on the predicted wind energy potential in my country.
For comparative analysis, my country was divided into seven regions according to typical geographical and climatic conditions, namely, Northeast China (NEC), North China (NC), East China (EC), South China (SC), Central China (CC), Northwest China (NWC), and Southwest China (SWC), and the next 30 years were divided into two periods (2031-2060 and 2071-2100), and the changes were evaluated compared with the base period of 1985-2014.

Research results: my country's wind energy development faces challenges from climate change
The validation results show that the ECA-Net downscaling model performs well in my country and its sub-regions.
Specifically, as shown in the figure below, under the three carbon emission scenarios of high, medium and low, the researchers calculated the spatiotemporal variation trends of wind energy density in each sub-region. The results showed that it was statistically significant at an α level of 0.05 (except for the central region under the SSP5-8.5 scenario).my country’s overall wind energy density is expected to show a slight downward trend during this century, with the magnitude of the trend depending on the emissions scenario.Except for East China, Central China and South China, the relative changes of wind energy density in other sub-regions show a large downward trend, especially under the SSP5-8.5 scenario. There are obvious regional differences in the changes of wind energy density in different regions.
The change in wind energy density in southern my country (East China, Central China and South China) is relatively low. Among all sub-regions, North China is likely to experience the largest reduction in wind energy density, which may reach 22.79% by 2100 under the SSP5-8.5 scenario.

Increases in wind intensity are projected to occur in relatively cooler months and to be larger under the higher emissions scenario.Except for the Middle Ages (2031–2060) under the SSP2–4.5 scenario, wind energy resources in most parts of my country will experience a reduction.
Under the three scenarios, the wind energy potential decreases more at the end of the century than at the middle of the century. The ensemble projection shows that by the end of the century (2071-2100), the average annual wind energy resources in Chongqing, Hunan and parts of Hainan will increase under the SSP2-4.5 and SSP5-8.5 scenarios.
Changes in wind energy resources show obvious seasonality. The change in wind energy resources in most regions is greater in winter than in summer. In 2031-2060, under the SSP2-4.5 and SSP5-8.5 scenarios, the wind energy potential in most regions in winter shows an increasing trend, while the opposite is true in summer - this obvious seasonal feature may be attributed to the seasonal changes in monsoons and ocean currents.
The stations in the north and west of my country identified by K-means clustering showed a clear decreasing trend, which indicates that wind energy resources in some parts of my country may decrease and become more unstable both within the year and between years due to climate change.

Prediction of interannual variation of wind energy density in the two future periods 2031-2060 and 2071-2100 (unit: %)

Predicted changes in annual variation of wind power density in the two future periods 2031-2060 and 2071-2100 (unit: %)
These findings suggest that the sustainability of wind energy development in my country may be challenged by climate change.
Wind energy resources have great prospects but still face challenges
In order to mitigate the greenhouse effect caused by carbon dioxide emissions from the combustion of fossil energy, the traditional energy structure must be reformed and upgraded to promote the development of clean and low-carbon energy. Wind energy, as a clean and renewable energy source, is inexhaustible and very suitable for coastal islands, grassland pastoral areas, mountainous areas and plateau areas that are short of water, fuel and have inconvenient transportation. Therefore, wind power generation is increasingly valued by countries around the world and is forming a global craze.
Wind power generation has huge potential, with global wind energy of about 2.74×109 MW, of which 2×107 MW, which is 10 times greater than the total amount of hydropower that can be exploited on Earth. According to statistics from the International Renewable Energy Agency (IRENA), the global wind power industry has developed rapidly in recent years and has broad prospects. In 2017, global wind power generation exceeded 100 billion kWh, reaching 114.15 billion kWh, a year-on-year increase of 18.65%; in 2022, global wind power generation broke a new record, reaching 210.48 billion kWh, an increase of 13.52% from 2020.

(Source: GWEC, Zhiyan Consulting)
In my country, the development of wind power generation is conducive to balancing the contradiction between energy supply and demand and achieving sustainable development, and it is of great practical significance for achieving the goal of "carbon neutrality". Data released by the National Energy Administration show that as of November 2021, my country's wind power grid-connected installed capacity reached 300.15 million kilowatts, breaking the 300 million kilowatt mark, which is 1.4 times the total installed capacity of wind power in the European Union at the end of 2020 and 2.6 times that of the United States. It has ranked first in the world for 12 consecutive years.
However, compared with conventional energy, the intermittent and variable characteristics of wind power result in relatively strong volatility and randomness. Wind power grid connection will inevitably bring huge impact to the power system and affect the quality of power. In addition,The long-term changes in wind speed also bring certain challenges to the planning and layout of the wind energy industry, which puts forward an urgent need to predict future changes in wind speed and wind power.
Observations show that China's surface wind speed has continued to decrease in recent years, with large regional and seasonal differences. There is still a long way to go to effectively control carbon emissions, develop wind energy in a regional, seasonal and all-round manner according to local conditions, and ensure the dispatch and utilization of clean energy in China in the future.
References:
1.https://pubs.acs.org/doi/10.1021/acs.estlett.3c00829
2.https://pubs.acs.org/doi/suppl/10.1021/acs.estlett.3c00829/suppl_file/ez3c00829_si_001.pdf
3.http://dqkxxb.cnjournals.org/dqkxxb/article/html/20230308
4.https://www.chyxx.com/industry/1154543.html