Southeast University Proposes a Photovoltaic Cell Defect Detection Model With an Accuracy Rate of 91.74%, Introducing Neural Structure Search for the First Time
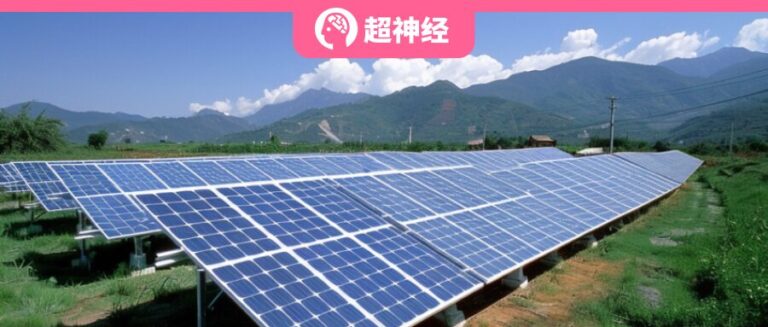
Riding on the global trend of "green development and low-carbon transformation", the photovoltaic (PV) industry has been developing rapidly since the 21st century at an amazing speed. In my country, photovoltaic power generation has shown unprecedented vitality. According to the national power industry statistics for January to March 2023 released by the National Energy Administration in April 2023, by the end of March of that year, my country's cumulative photovoltaic installed capacity had surpassed hydropower and became the second largest power source in the country.
With the large-scale application of photovoltaic power generation, how to ensure the stability and sustainable development of photovoltaic power supply has also become an important research topic in the industry. Photovoltaic modules are the basic units of photovoltaics. Affected by various external factors such as long production chains and complex application scenarios, modules are prone to damage during their manufacturing, transportation, and installation. If these defective modules are put into actual operation, it is very likely to cause the power of the entire power supply system to decrease or even cause safety problems. Therefore, the requirements for on-site maintenance and fault diagnosis of photovoltaics are becoming increasingly higher.
At present, the more traditional PV defect detection methods include current-voltage (IV) curve, infrared thermal imaging (IRT), etc., but due to the limitation of recognition accuracy, the above methods cannot effectively identify potential risk factors such as microcracks. Convolutional neural network (CNN) is becoming the mainstream method of PV defect detection with its powerful feature capture ability, combined with high-resolution electroluminescence (EL) imaging.However, CNN-based model parameters are usually large and have strict requirements on hardware resources, so it is difficult to be deployed on a large scale in actual industrial projects.
In order to meet the industrial field's requirements for both accuracy and speed of defect detection, Professor Zhang Jinxia's team from the School of Automation at Southeast University,A new lightweight and high-performance photovoltaic cell electroluminescence (EL) image defect automatic detection model based on neural architecture search (NAS) and knowledge distillation is proposed.
Currently, the research results have been published on arXiv, titled "A lightweight network for photovoltaic cell defect detection in electroluminescence images based on neural architecture search and knowledge distillation".
Research highlights:
* Proposed a lightweight model for photovoltaic cell defect detection with an accuracy of up to 91.74%;
* For the first time, NAS was introduced into the field of photovoltaic cell defect detection for automated lightweight network design, reducing the workload of manual design;
* The introduction of knowledge distillation makes full use of various prior knowledge, and experiments have proved the effectiveness of this design in improving the ability to identify defective photovoltaic cells.

Paper link:
https://arxiv.org/abs/2302.07455
Follow the official account and reply "photovoltaic cells" to download the paper
Datasets and Data Augmentation
The dataset for this study is a public photovoltaic cell dataset, which contains 2,624 photovoltaic cell EL images with a resolution of 300 x 300 pixels, including both single crystal and multi-crystalline types.The research team used 0.5 as the threshold to divide the samples into functional samples and defective samples. 75% images, or 1,970 images, were randomly selected as the training set, and the remaining 654 images were used as the test set. The training set was further divided into a search training set and a search test set. All images were resized to 150 x 150 pixels.

Data augmentation is to obtain more representations from the original data while increasing the data slightly, thereby improving the quality of the original data and helping the model reduceOverfitting, and enhance robustness.Data augmentation operations include random horizontal flip, random vertical flip, random rotation in the range of (-2°, 2°), random rotation in the range of {0°, 90°, 180°, 270°}, and randomAffine transformation.
The model proposed in this study is composed of stacked Normal cells and Reduction cells. The internal structure obtained by the search algorithm is shown in the following figure:

A defect detection method based on NAS and knowledge distillation
The lightweight network design architecture is shown in the figure below. The researchers automatically obtained a lightweight network in the designed search space through the NAS algorithm, and made full use of the prior knowledge obtained from the existing pre-trained large-scale model through knowledge distillation to improve the model performance.

First, the research team used the continuous gradient-based NAS framework DARTS to automatically design a model for photovoltaic cell defect detection. Based on the fast search characteristics of DARTS, the researchers further considered the visual multi-scale features of PV defects and designed a suitable search space to enhance feature recognition of different defect sizes.

The search space used by the lightweight network is mainly composed of two stacked unit structures, namely 5 normal cells and 4 reduction cells.The normal cell is set to maintain the input size, and the reduction cell is set to have the function of downsampling. As shown in the figure above, each unit fuses two features of different scales from the first two units, and the first normal cell takes the same feature as two inputs.

The first three reduction cells perform downsampling and channel expansion, and the number of channels in the last reduction cell remains unchanged. The proposed lightweight network classifies the input photovoltaic cells as functional or defective.
Secondly,Knowledge distillation is one of the most effective model compression methods. It adopts the Teacher-Student model and can transfer knowledge from the Teacher model to the Student model.Those network architectures that cannot utilize the prior knowledge in the pre-trained model can improve their performance by learning the knowledge of the Teacher network. In this experiment, since the lightweight network can only be trained from scratch, by using knowledge distillation, it can better utilize prior knowledge for training.
The researchers transferred four different knowledge priors - attention information, feature information, logit information, and task-oriented information to enhance the distillation effect on the photovoltaic cell defect detection task.

Outstanding model performance
The research team compared the proposed lightweight model with the Teacher model and other studies, and tested it on private datasets to further demonstrate the effectiveness of the network architecture.
The research team compared the model with 6 manually designed neural networks and the Teacher model on a public dataset under the same 200 epochs. The results are shown in the figure below:

The results show thatThe lightweight model proposed in this study achieved an accuracy of 91.74% on the test set, even exceeding the Teacher model level of 1.22%.At the same time, the model proposed by the institute has smaller parameters, uses fewer resources than some classic large models, and is easier to deploy on actual terminal devices.

The figure above is a comparison of the model's accuracy in identifying defective photovoltaic cells and functional photovoltaic cells. The accuracy of the model proposed by the researchers in identifying defective photovoltaic cells reached 86.28%, far exceeding other methods.
It is concluded thatCompared with other manually designed models, the model proposed by the research team can not only be automatically searched through the NAS algorithm, reducing the workload, but also achieve higher performance under a relatively lightweight architecture, proving the effectiveness of the proposed method.
To further evaluate the performance of the proposed model, the research team also provided a performance comparison on monocrystalline or multicrystalline PV, as shown in Table 4:

Right table: Comparison of polycrystalline photovoltaic cells only in the ELPV public dataset with other methods under the same data augmentation conditions
On monocrystalline PV cells, every indicator of the proposed model reaches the highest level, and as for the more difficult multicrystalline model, the model also far exceeds other models.
Efficiency and generalization
Deployment on terminal devices requires comprehensive consideration of model size and computational effort. To test efficiency, the research team evaluated the model on a CPU platform (Intel i9-10980XE 24.75M Cache, 3 GHz).

Comprehensive evaluation found that the model is far superior to other small models and even large classical models.This lightweight model can meet the deployment requirements of some common low-power embedded devices.Such as Raspberry Pi-4B (4GB, 15W, 9~10 GFLOPS) and NVIDLA Jetson Nano (4GB, 10W, 7.368 GEFLOPS FP64).
In order to verify the generalization performance of the model on different data sources, the research team trained the model on a private photovoltaic cell dataset. 8,580 images with a pixel resolution of 256 x 256 were extracted from photovoltaic cells of three specifications: 6 x 10, 6 x 12, and 6 x 24, including 482 defective samples and 8,089 functional samples.
The results show thatThe model is about 2.3% higher than the Teacher model in terms of balanced accuracy and defect sample accuracy, respectively.Compared with other methods, the gap is huge. The accuracy of this model for defect samples reaches 94.26%, especially in real-world scenarios, it shows better defect recognition capabilities. As shown in the following figure:

Photovoltaic power generation started a hundred years ago, and it is still the "darling" of green energy
As a representative of new energy, the research on photovoltaic power generation technology has actually been going on for over a hundred years. In 1839, French physicist Becquerel first discoveredPhotovoltaic effectAfter that, after continuous research and efforts by scientists from various countries, the first practical photovoltaic cell was finally introduced in 1954. Today, the photovoltaic industry has once again caught the wind of global green development, especially after the "dual carbon" goal was clearly put forward in China, the development of the photovoltaic industry has a bright future.
This can be seen from the national power industry statistics released by the National Energy Administration not long ago. The data shows that as of the end of December, the country's cumulative installed power generation capacity was about 2.92 billion kilowatts, a year-on-year increase of 13.9%, of which the installed capacity of solar power generation was about 610 million kilowatts, achieving a year-on-year increase of 55.2%.
From a policy perspective,May 30, 2019The National Energy Administration issued the "Notice on Matters Related to the Construction of Wind Power and Photovoltaic Power Generation Projects in 2019".It clearly states that priority should be given to the construction of grid-parity projects without subsidies, and then the competitive allocation of projects that require national subsidies should be carried out. This shows that although my country's photovoltaic industry has entered a mature period of stable development, promoting the development of the photovoltaic industry will still be the key task of realizing the transformation of the domestic energy structure in the future.
In 2023, the National Energy Administration, the National Development and Reform Commission and other departments successively issued a number of documents on energy work.It is clearly stated that photovoltaics is the key direction of the energy industry in 2023.
This study has brought good news to the development of the photovoltaic industry from an industrial level. The research results of Professor Zhang Jinxia's team provide effective tools for photovoltaic cell defect detection from the practical application side. In particular, the introduction of NAS and knowledge distillation provides a new idea for designing application scenario models. This may bring new inspiration to the industry for the application of deep learning in the photovoltaic field, thereby better combining technology and industry.