Independent Research and Development! The Team of the Military Medical Research Institute Proposed MIDAS, Which Can Be Used for Mosaic Integration of Single-cell Multi-omics Data
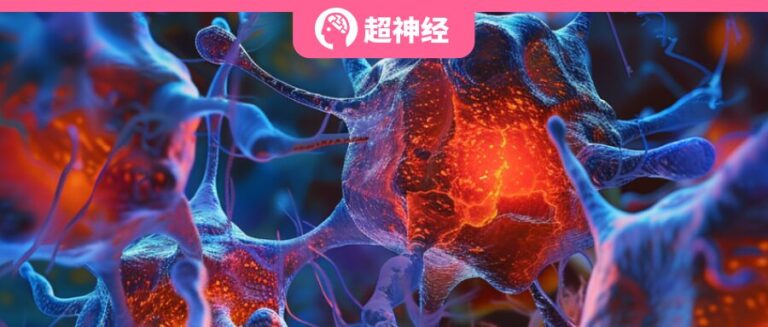
As we all know, cells are the smallest building blocks of life. The human body contains 40-60 trillion cells, which form the basis of our growth and development. Conducting research at the single-cell level is crucial for accurately understanding cell growth and development as well as the diagnosis and treatment of diseases.
In recent years, single-cell sequencing technology has emerged as a hot topic in molecular biology research. The industry has generated a large amount of single-cell sequencing data around clinical and basic research issues such as disease and development. However, the massive data from different omics combinations, different sequencing technologies, and different sequencing samples are as scattered and diverse as mosaic tiles on the floor.How to integrate such huge and messy data and conduct biomedical research is a common challenge faced by scientists around the world.
In order to overcome this challenge, Ying Xiaomin's team and Bo Xiaochen's team from the Military Medical Research Institute recently conducted Nature Biotechnology The journal published a research paper titled "Mosaic integration and knowledge transfer of single-cell multimodal data with MIDAS".This study proposed a computational tool MIDAS for mosaic integration of single-cell multimodal omics (scMulti-omics) data (i.e., different data sets only share some detection modalities) and knowledge transfer.Based on self-supervised learning and information-theoretic approaches, we have realized for the first time the universal integration functions of single-cell multi-omics mosaic data, such as modal alignment, data completion, and batch correction, providing important original technologies for constructing large-scale multi-omics cell maps and realizing large-scale single-cell multi-omics analysis and knowledge transfer.
Research highlights:
* Independently developed a new algorithm based on generative artificial intelligence, MIDAS
* For the first time, the integration functions of modality alignment, data completion, batch correction, etc. of common single-cell multi-omics mosaic data were realized
* The new algorithm is of great significance for revealing the functions of cells and molecular regulatory mechanisms and studying the occurrence and development of diseases

Paper address:
https://www.nature.com/articles/s41587-023-02040-y
Follow the official account and reply "single cell" to get the complete PDF
Dataset: Multiple datasets, multi-dimensional evaluation performance
In order to compare the advantages of the MIDAS model from various dimensions, this study constructed multiple data sets.
First, to compare MIDAS with state-of-the-art methods,This study evaluated the performance of MIDAS in trimodal integration with full modalities (a simplified form of mosaic integration), a task the research team named “rectangular integration”. The team used two published single-cell trimodal human PBMC Datasets (DOGMA-seq and TEA-seq) were constructed by measuring RNA, ADT and ATAC of each cell at the same time. Note: PBMC stands for peripheral blood mononuclear cell, which is commonly used in scientific research activities in the field of immunology.
Secondly, to evaluate the performance of MIDAS in mosaic integration,Based on the previously generated rectangular dataset, the research team further constructed 14 incomplete datasets, each of which was generated by deleting multiple modal batch blocks from the full modal dataset.
Third, in order to study the knowledge transfer capability of MIDAS,The research team re-divided the atlas dataset into a reference dataset for atlas construction and a query dataset. By removing DOGMA-seq from the atlas, the research team obtained a reference dataset named atlas-no_dogma.
Fourth, to investigate the application of MIDAS in single-cell datasets with continuous cell state changes,The research team constructed a human BMMC mosaic dataset by combining three different samples (ICA, ASAP and CITE) obtained from public scRNA-seq (single-cell RNA-sequencing).
Model architecture: Deep generative model MIDAS
MIDAS is a deep generative model that represents the joint distribution of incomplete single-cell multimodal data, which includes measurements of transposase-accessible chromatin (ATAC), RNA, and antibody-derived tags (ADTs).

Specifically, MIDAS assumes that multimodal measurements of each cell are generated based on a deep neural network via two modality-independent and decoupled latent variables: biological state and technical noise.Its input includes a mosaic feature-cell count matrix consisting of different single-cell samples (batches) and a vector representing the cell batch ID.These single-cell samples may come from different experiments or be generated by applying different sequencing technologies (such as scRNA-seq, CITE-seq, ASAP-seq, and TEA-seq), and therefore may have different technical noise, modalities, and characteristics.

The outputs of MIDAS include biological state and technical noise matrices, and estimated and batch-corrected count matrices, from which missing modalities and features in the input data are interpolated and batch effects are removed.These outputs can be used for downstream analyses such as clustering, cell type delineation, and trajectory inference.
MIDAS is based on the architecture of variational autoencoder (VAE), with modular encoder network and decoder network. The former can process mosaic input data and infer latent variables, and the latter can use latent variables to start the generation process of observed data. MIDAS uses self-supervised learning to align different modalities in latent space to improve cross-modal inference in downstream tasks such as interpolation and translation. At the same time, information theory is also applied to decouple biological state and technical noise to further achieve batch correction.
The researchers combined these elements into the optimization objectives of this study and achieved scalable learning and inference of MIDAS through stochastic gradient variational Bayes (SGVB), which also made large-scale mosaic integration and atlas construction of single-cell multimodal data possible. In addition, in order to transfer the knowledge in the constructed atlas to query datasets with different modal combinations, the researchers developed transfer learning and cross-reference mapping schemes for the transfer of model parameters and cell labels.
Research results: MIDAS is versatile and efficient
The results of this study indicate that MIDAS is a powerful, versatile and efficient single-cell multimodal integration tool.
The research team compared the performance of MIDAS with nine recently published methods in terms of eliminating batch effects and preserving biological signals.
The results show thatMIDAS ideally eliminates batch effects and preserves cell type information on dogma-full and teadog-full datasets, while the performance of other methods is slightly inferior.For example, BBKNN+average, MOFA+, PCA+WNN, Scanorama-embed+WNN, and Scanorama-feat+WNN did not mix different batches well, and the cell clusters generated by PCA+WNN and Scanorama-feat+WNN were largely inconsistent with the cell types.

Results obtained from evaluation and downstream analysis
In terms of batch alignment – MIDAS is able to align cells from different batches very well and group them consistently with cell type labels.While other methods cannot mix cells from different batches well and produce cell clusters that are largely inconsistent with cell types. The scIB benchmark shows that MIDAS has stable performance on different mosaic tasks and its overall score is much higher than other methods.

Qualitative and quantitative performance evaluation scores
In terms of knowledge transfer capability, researchers aligned each query dataset with the reference dataset and used k-nearest neighbors (kNN) algorithm to transfer cell type labels. Mapping and visualizing biological states shows consistent cross-referencing results across query datasets and high agreement with atlas integration results obtained with the dogma-full dataset. MIDAS enables robust and accurate label transfer, obviating the need for de novo integration and downstream analysis.Therefore, MIDAS can be used to transfer atlas-level knowledge to various forms of user datasets without expensive de novo training costs or complex downstream analysis.

In summary, by modeling the single-cell mosaic data generation process, MIDAS can accurately separate biological states and technical noise from inputs and robustly adjust modalities to support multi-source and heterogeneous integrated analysis. MIDAS provides accurate and robust results when performing various mosaic integration tasks and outperforms other methods.
Furthermore, MIDAS efficiently and flexibly transfers knowledge from reference datasets to query datasets, making it easy to process new multi-omics data. With its superior dimensionality reduction and batch correction performance, MIDAS supports accurate downstream biological analysis. In addition to enabling clustering and cell type identification of mosaic data, MIDAS can also assist in pseudo-temporal analysis of cells with continuous states, which is particularly valuable when no RNAomics data is available. When transferring knowledge between different tissues, MIDAS is able to align heterogeneous datasets and identify cell types, even new types.
Single-cell multi-omics analysis continues to advance
Just as we can see the world from a grain of sand, scientists can also see the multiverse, or more accurately, "multi-omics," from within a tiny cell.
A range of different techniques are used to study the genome, transcriptome, epigenome and other features of single cells, and although each technique is informative on its own, their combined analysis – known as multi-omics – provides a more complete picture.Currently, driven by single-cell multi-omics, cell biology and translational research have made significant progress, but data integration and analysis remain challenges for many scientists.
Based on this, in addition to the Ying Xiaomin team and the Bo Xiaochen team mentioned above, there are more research teams and companies that are following suit, trying to explore more efficient and simpler data processing methods.
for example,Analytical methods such as the Chromium single-cell platform from 10x Genomics continue to expand, allowing the assessment of multiple cellular features in different combinations.Including whole transcriptome gene expression, protein expression, full-length pairing TCR and BCR sequencing, antigen specificity, and open chromatin analysis. Cell Ranger The solution uses a set of free and easy-to-use analysis processes to analyze Chromium single-cell data, which can process raw data, perform comparisons, and count genes. In addition, Cell Ranger can also be integrated with cloud analysis platforms to monitor, manage, and process data.
For example,On May 2, 2022, Gao Ge's research group at Peking University/Changping Laboratory published a research paper titled "Multi-omics single-cell data integration and regulatory inference with graph-linked embedding" in Nature Biotechnology.A deep learning method called GLUE based on graph coupling strategy was proposed, which for the first time achieved unsupervised precise integration and regulatory inference of millions of single-cell multi-omics data.
The continuous development of these bioinformatics tools and software will help researchers interpret complex multi-omics data sets and promote the development of cell biology. It is of great significance for revealing the functions and molecular regulatory mechanisms of cells and studying the occurrence and development of diseases, and ultimately benefiting the people.
References:
1.https://www.chinagut.cn/articles/ss/02bc1e86e3734acebff57395d6e044a6
2.https://m.ebiotrade.com/newsf/2023-10/20231023151001602.htm
3.https://news.bioon.com/article/e49a810955a1.html
4.https://m.thepaper.cn/newsDetail_forward_26137031