Shanghai Jiao Tong University and Tsinghua University Jointly Released DeepDR Plus, Which Can Predict the Progression of Diabetic Retinopathy Within 5 Years Using Only Fundus Images
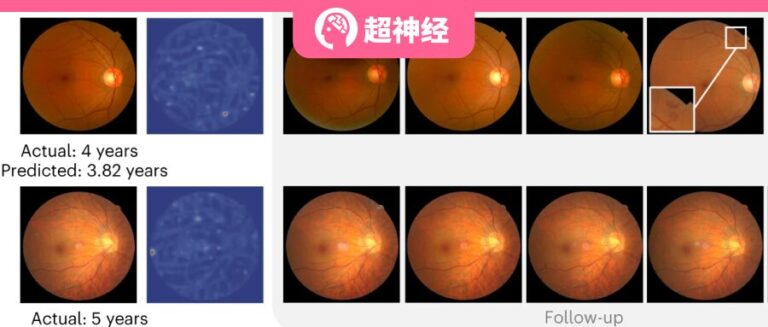
In 1996, Ella Fitzgerald, known as the first lady of jazz,Beverly HillsThe talented singer, who won 13 Grammy Awards and sold over 40 million albums, eventually died of diabetic retinopathy (DR). Today, diabetes has been listed as one of the four major chronic diseases in my country, with one in every 10 people suffering from diabetes. According to research data published by Academician Jia Weiping in July 2023, there are currently about 19.5 million patients with diabetic retinopathy in my country.
Data source:https://www.nature.com/articles/s41467-023-39864-w
Generally speaking, diabetic retinopathy is relatively hidden in the early stages and develops without symptoms, but in the late stages, patients are almost blind and irreversible, and has become the main cause of preventable blindness in adults aged 20-74. Diabetic retinopathy usually progresses slowly, but is affected by multiple risk factors. The risk of onset and progression varies greatly among different individuals, so accurate diagnosis and assessment of development risk have become a major challenge for doctors and patients.
As AI plays an increasingly important role in the medical field, deep learning and convolutional neural networks have been used to automatically detect diabetic retinopathy from retinal photographs.But few are able to predict risks in a forward-looking manner.
To this end, the team of Professor Jia Weiping and Professor Li Huating, Dean of the Institute of Active Health Strategy and Development of Shanghai Jiao Tong University, Department of Endocrinology and Metabolism of Shanghai Sixth People's Hospital, and Shanghai Key Laboratory of Diabetes, the team of Professor Sheng Bin from the Department of Computer Science, School of Electrical Engineering of Shanghai Jiao Tong University/Key Laboratory of Artificial Intelligence of the Ministry of Education, and the team of Professor Huang Tianyin, Vice Dean of Academic Affairs and Director of the School of Medicine of Tsinghua University, built DeepDR Plus, a diabetic retinopathy complication early warning system based on deep learning of time-series image sequences. It can predict the progression of diabetic retinopathy within 5 years based on fundus images alone.
Research highlights:
* Developed and validated a deep learning system (DeepDR Plus) to predict diabetic retinopathy progression using fundus images alone
* The system was applied to real clinical cases in China and India, extending the average screening interval in clinical applications from 12 months to 31.97 months
* The system can significantly reduce screening frequency and public health costs while maintaining a very low missed diagnosis rate

Paper address:
https://doi.org/10.1038/s41591-023-02702-z
One-click download of the dataset:
https://hyper.ai/datasets/29716
Follow the official account and reply "DR" to get the complete PDF
Dataset: Clinical data from China and India
First, to learn features associated with diabetic retinopathy, the DeepDR Plus system was pre-trained using 717,308 fundus images of 179,327 diabetic patients from the Shanghai Comprehensive Diabetes Prevention and Care System (Shanghai Comprehensive Model) and the Shanghai Diabetes Prevention Program (SDPP).
Among them, SDPP is a community-based longitudinal cohort study including 79,284 participants who underwent physical examinations at Huadong Sanatorium and Shanghai Sixth People's Hospital from December 2015 to November 2022, of which 25,231 participants completed at least 4 years of annual follow-up.
The researchers then developed and validated the model in an internal dataset consisting of 76,400 fundus images of 19,100 diabetic patients from the Diabetic Retinopathy Progression Study (DRPS) cohort, which was split into a development dataset and an internal test set in a 9:1 ratio. Eight independent longitudinal cohorts were used for external validation.

To evaluate the effectiveness of the DeepDR Plus system integrated into clinical workflow,The study also conducted a real-world study in a community-based prospective cohort study of Chinese adults.A total of 2,185 participants were included in the analysis, including 538 participants in the integrated management (IM) group (general hospital-community diabetes management program) and 1,647 participants in the non-IM group.The study also conducted a real-world study in an Indian prospective cohort (SN-DREAMS).Among them, 992 diabetic patients were followed up for 4 consecutive years.
Model: Fundus images alone can effectively predict disease progression
The DeepDR Plus system includes three models for predicting diabetic retinopathy progression:Data Model, fundus model and combined model. Among them:
The fundus model uses ResNet-50 as the backbone to extract features from fundus images and uses a soft-attention layer to select the features with the most information.The study first used Momentum Contrast (MoCo,v2) to create a pre-trained feature extractor using self-supervised learning to extract features from fundus images. At the same time, the study also used the concordance index (C-index) and IBS The integrated Brier score assessment model predicted the performance of diabetic retinopathy in participants over the next 5 years.
The metadata model inputs metadata to generate survival predictions,These included age, sex, smoking status, duration of diabetes, baseline DR level, body mass index, glycated HbA1c, systolic blood pressure, diastolic blood pressure, triglycerides, low-density lipoprotein cholesterol, and high-density lipoprotein cholesterol.
The combined model inputs both the fundus score and metadata of the fundus model.
In the internal validation, the consistency indexes of the metadata model, fundus model, and combined model for predicting the progression of diabetic retinopathy in patients were 0.696, 0.823, and 0.833, respectively. The results showed that the performance of the combined model was similar to that of the fundus model and better than that of the metadata model. This demonstrates the accurate predictive performance of the fundus model. In 8 independent external datasets, the models achieved similar performance in predicting the progression of diabetic retinopathy.This indicates that the DeepDR Plus system has high consistency and strong calibration.

To determine when patients should seek ophthalmologist help to assess the extent of DR progression, the study also conducted three subgroup analyses to further demonstrate the predictive power of the DeepDR Plus system. The three subgroups included diabetic patients without retinopathy transitioning to DR (subgroup 1), DR not requiring referral to DR requiring referral (subgroup 2), and non-vision-threatening DR to vision-threatening DR (subgroup 3).
The study used the DeepDR Plus system for three subgroups to predict the deterioration of different types of DR grades within 5 years through baseline retinal images. The results showed that the consistency index of the metadata model in the three subgroups was 0.700-0.711, and IBS was 0.261-0.328; the consistency index of the fundus model increased to 0.826, 0.820, and 0.824, and IBS decreased to 0.153-0.189; the consistency index of the combined model was 0.835-0.852, and IBS was 0.145-0.167.
In addition, the study evaluated the predictive performance of the fundus model on an external dataset and achieved comparable results to an internal dataset.The results showed that fundus images alone could effectively predict disease progression.

AI-driven personalized screening intervals
In this study, the IM group received regular clinical tests and metabolic measurements, and was provided with guidance and advice by experts from a general hospital. Therefore, the study further divided the IM group and the non-IM group into low-risk and high-risk groups, and evaluated all participants using the fundus model and metadata model in the DeepDR Plus system.

In the non-IM group, patients in the high-risk group of the fundus model were more likely to develop diabetic retinopathy, and patients in the low-risk group of the fundus model were less likely to develop diabetic retinopathy compared with the metadata model.

In addition, the study evaluated the performance of personalized screening regimens recommended by metadata models or fundus models compared with fixed annual screening.If all participants in the IM and non-IM groups followed the recommended personalized screening interval given by the fundus model, the average screening interval could be extended from 12 months to 31.97 months.The fundus model achieved similar reductions in screening frequency compared to the metadata model, while significantly reducing the delayed detection rate of DR. In addition, the delayed detection rate of any DR progression was lower in the IM group compared to the non-IM group using the screening intervals recommended by the fundus model (0.37% vs. 1.28%), indicating that the DeepDR Plus system is guaranteed to reduce the delayed detection rate of DR regardless of future interventions.

Kaplan-Meier plot of vision-threatening DR
In conclusion, compared with metadata models, fundus models can more accurately stratify participants, thereby enabling personalized interventions and reducing the frequency of DR screening while reducing the delay in detecting DR progression.
China is in the first echelon of AI diagnosis of diabetic retinopathy
recently,AGILE The Global Artificial Intelligence Governance Assessment Index was officially released, which is the first assessment to decode the new global artificial intelligence governance landscape. The assessment results show that in terms of the level of artificial intelligence development, the United States and China are in the leading position in terms of total volume.

Thanks to the rapid development of domestic artificial intelligence technology, my country has made gratifying progress in the field of artificial intelligence-assisted medical care, and can keep pace with the United States in the screening of diabetic retinopathy alone. As early as 2013, Jia Wei's team and Li Huating's team from the Sixth People's Hospital affiliated to Shanghai Jiao Tong University School of Medicine, together with Sheng Bin's team from the Department of Computer Science at Shanghai Jiao Tong University, began to explore the automatic extraction technology of diabetic retinopathy features.
In 2016, Google used a deep learning system to accurately diagnose moderate to severe DR after training with a large amount of diabetic retinopathy image data. The results were published in the Journal of the American Medical Association (JAMA) .
Inspired by Google, Huang Tianyin, then director of the Singapore National Eye Center, was shocked. At the same time, Huang Tianyin believed that Google's research still had the limitation of lacking multi-ethnic verification. In 2017, Huang Tianyin and the Singapore National Eye Center team successfully developed a new deep learning system, which was the first to effectively diagnose DR and other related eye diseases in multi-ethnic and multi-national cohorts. The results were subsequently published in the JAMA journal.
Also in 2017, the Hangzhou Health and Family Planning Commission took the lead in launching a diabetic retinopathy screening project, introducing a portable fundus camera based on artificial intelligence-assisted diagnosis to solve the problem of lack of professional medical resources at the grassroots level. At the same time, a number of artificial intelligence medical devices have entered the market in China, bringing new hope of disease cure to more patients.
In 2018, Jia Weiping's team cooperated with Huang Tianyin's team and joined hands with world-class academic institutions such as the Singapore National Eye Center to obtain approval to establish the Shanghai "Belt and Road" International Joint Laboratory for Intelligent Prevention and Control of Metabolic-Related Diseases to carry out cooperation in the field of diabetes prevention and treatment.
Although everything is developing in full swing, the AI diagnostic system has not been further verified in clinical diagnosis and treatment immediately. In 2020, the Google team released a report stating that its AI diagnostic system for diabetic retinopathy showed strong "incompatibility" in clinical application in Thailand. More than one-fifth of the images were rejected by the system due to clarity issues. Nurses had to retake photos, and patients were transferred to other hospitals for treatment. The relevant AI system was rejected after being implemented in 11 clinics in Thailand.
While AI diagnostic systems are encountering clinical obstacles overseas, domestic AI diagnostic systems are beginning to enter a period of rapid development. In August 2020, my country's first batch of fundus image-assisted diagnosis software for diabetic retinopathy based on deep learning technology was approved for marketing. In 2021, Nankai University released CABNet (Category Attention Block) and proposed the Global Attention Block, which can significantly improve the performance of existing deep architectures with only a small number of additional parameters and achieve excellent performance in DR grading.
Also in 2021, Jia Weiping's team and Sheng Bin's team jointly launched the DeepDR system, which can accurately distinguish different degrees of retinal lesions from mild to proliferative stages.The core achievements have been granted 3 invention patents in China and 1 invention patent in the United States, and have been put into use in many hospitals across the country. In the same year, Huang Tianyin became a professor at Tsinghua University. The medical and engineering cross-disciplinary teams of Shanghai Jiaotong University and Tsinghua University began to carry out closer multidisciplinary cooperation and collaborative research on diabetes AI-assisted management technology and clinical practice, which also accelerated the previous research of both parties.
Today, the launch of the DeepDR Plus system has greatly improved the efficiency, fairness and accessibility of fundus film screening in developing countries, and has opened up a new path for improving the quality, efficiency and reform and innovation of diabetes management models in low- and middle-income countries and regions around the world. In the near future, artificial intelligence will also bring new hope of cure to more diabetic patients.
References:
1.https://new.qq.com/rain/a/20240123A05OOZ00
2.https://m.thepaper.cn/baijiahao_20929983
3.https://baijiahao.baidu.com/s?id=1791412684120469650&wfr=spider&for=pc
4.https://www.nsfc.gov.cn/csc/20340/20343/57820/index.html