The AI4S Team of Shanghai Jiao Tong University Proposed the Concept of "intelligent Scientific Facilities" to Establish an Interdisciplinary AI Research Assistant
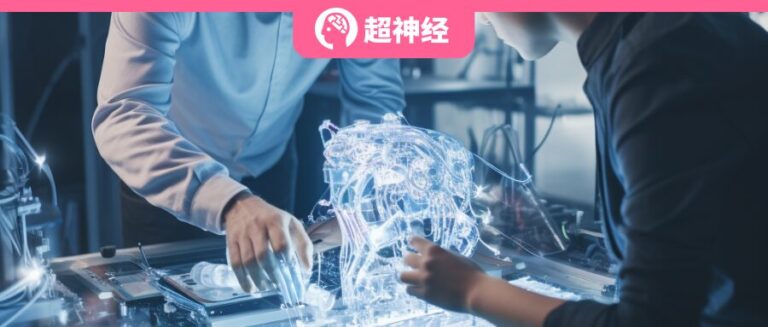
In recent years, the application of artificial intelligence in scientific research has continued to develop in depth, while also expanding the breadth of its application areas, from protein folding to new material discovery, from disease prediction to prognosis and treatment, from astronomical exploration to natural disaster analysis... Behind the blossoming of AI for Science, on the one hand, domestic and foreign AI companies have carried out research and development in the field of scientific research, which has lowered the threshold for the use of AI tools; on the other hand, in the process of accepting "AI helpers", scientific researchers have developed an efficient collaboration model with them.
An analysis by Nature shows that in the Scopus database, the proportion of papers that mention artificial intelligence or artificial intelligence-related keywords in the title or abstract has increased from 2% ten years ago to 8% now. However, looking at the big models and other tools released by technology giants such as Google DeepMind, as well as the related research results published by research teams from universities at home and abroad, most of them are aimed at specific problems in specific fields and lack replicability.
Professor Lin Zhouchen of the School of Intelligence at Peking University said in an interview with HyperAI: "Current AI tools lack uniformity. Even if we only focus on mathematics, there are already big differences between AI tools developed for number theory and geometry, not to mention interdisciplinary AI tools. AI tools have not yet become a basic foundation like today's computers, which can be easily accessed. Current computer languages include C, Java, Python, etc., and it is entirely possible to solve problems in multiple disciplines such as mathematics, physics, and chemistry based on any one of these languages, which reflects its versatility. However, this is not the case with AI tools. Just look at Google DeepMind's Alpha series to know this."
In view of this,Professor Yang Xiaokang and others from the AI for Science team at the Shanghai Jiao Tong University Institute of Artificial Intelligence proposed a concept for the construction of intelligent scientific facilities.The company has developed innovative functions such as large-scale scientific models, generative simulation and inversion, autonomous intelligent unmanned experiments, and large-scale reliable scientific research collaboration.Bulletin of the Chinese Academy of Sciences》.

Paper address:
http://www.bulletin.cas.cn/previewFile?id=52965146&type=pdf&lang=zh
Follow the official account and reply "AI4S" to get the full paper
Balancing innovation and empowerment
The AI for Science team of Shanghai Jiao Tong University proposed that in order to achieve the source innovation of basic science and major downstream technological innovation in the intelligent era, two core problems need to be solved urgently:
*How to build a new scientific intelligence facility based on the research paradigm of AI for Science?
*How to use the new generation of AI to empower traditional scientific facilities?

In terms of innovation,It is necessary to systematically and holistically unleash the creativity and versatility of the new generation of AI (especially generative AI and large models) in the field of basic science, realize innovative functions such as spontaneous hypothesis generation, automatic law deduction, autonomous unmanned experiments, and self-driven trusted collaboration, and promote ultra-large-scale, high-speed iterative scientific exploration.
Under traditional scientific facilities and research paradigms,The entire scientific research process is difficult to communicate scientific issues, conduct scientific experiments, and share scientific data. Some large-scale, high-tech scientific facilities and their scientific research environments are highly complex, and such difficulties are particularly prominent. Using the new generation of AI to achieve an efficient closed loop of "scientific issues (scientists) - experimental equipment (experimenters) - scientific research data and literature (scientific research institutions and intermediaries)" is not only a standard feature of newly built scientific facilities, but also a new demand and new opportunity in the process of upgrading and renovating existing scientific facilities.
Therefore, the team's idea of building an "AI enabled Scientific Facility" (AISF) takes into account two aspects: "creating highly intelligent new scientific facilities" and "empowering existing large scientific facilities."

As shown in the figure above, intelligent scientific facilities form a three-layer architecture of scientific intelligent facilities with people in the loop.
*Base support layer, through high-performance computing and computing power network, to form computing power support;
*Scientific model layer, build interdisciplinary and cross-modal scientific models and "AI research assistants";
* Experimental application layer, through AI-operated robots and intelligent experimental environments, autonomous unmanned experiments and multi-party scientific research collaboration can be achieved.
4 innovative features
Based on the three-layer architecture, intelligent scientific facilities can form four major new functions that the traditional paradigm does not have, namely, large scientific models, generative simulation and inversion, high-throughput autonomous unmanned experiments, and large-scale trusted scientific research collaboration.

Scientific Model
As an "AI assistant" to assist human scientists in conducting scientific research, scientific big models need to have an interdisciplinary knowledge background, be able to process cross-modal data input, and be able to master the calling of external scientific tools, combined with feedback and evaluation, to achieve continuous evolution.

As shown in the figure above, building a big scientific model requires forming four specialized capabilities on top of the basic big language model and building corresponding evaluation benchmarks.
The first is the ability to unify input across disciplines and modalities.The data generated by scientific research generally includes multimodal data such as formulas, charts, and molecular formulas in addition to text data. How to achieve unified input of these cross-modal data and then jointly model interdisciplinary professional knowledge is a challenging problem that needs to be solved urgently in establishing a big scientific model.
The second is the ability to effectively call on external scientific tools.Large models have the problem of outputting seemingly reasonable but actually erroneous content. A feasible technical approach to solve this problem is to use the large model as a planning and reasoning engine, and to improve credibility and accuracy by calling different types of external scientific tools.
The third is the model’s ability to continuously feedback and evolve.On the one hand, scientific big models can utilize high-quality feedback from researchers to improve professional knowledge in the scientific field, enhance the ability to model and infer scientific knowledge, and improve the level of scientific content generation, literature induction and reasoning. On the other hand, they can use experimental feedback to continuously improve their ability to generate scientific hypotheses and optimize experimental plans.
The fourth is illusion (Hallucination) Elimination capability.Hallucination refers to the phenomenon that the content generated by the model is inconsistent with real-world facts or user input. How to effectively screen expert knowledge and use high-precision professional knowledge for reinforcement learning to achieve iteration and updating of large models is an important method to eliminate scientific hallucinations. In addition, targeted optimization and integration based on the base model to improve the credibility and accuracy of the model in specific fields is also an important way to correct scientific hallucinations.
at last,The evaluation benchmark of scientific big models tests the cross-modal and cross-disciplinary data understanding and modeling capabilities of scientific big models on the one hand; on the other hand, it evaluates whether the scientific big models have the ability to use scientific tools to accurately and robustly complete complex tasks, and whether they have a strong ability to refuse to generate false and harmful content.
Generative simulation and inversion
Using simulation and computer simulation tools to achieve the deduction between "theory-phenomenon" is one of the important paradigms of scientific research. Traditional simulation methods based on numerical calculations have limitations and challenges in terms of solution speed and accuracy in many real-world scenarios.

Generative simulation and inversion of simulation space
As shown in the figure above, the generative neural network is the basic structure.The problem of numerical solution of complex systems can be transformed into a data fitting problem.Establish an efficient mapping from hypothesis space to simulation space to accelerate the solution. In addition, using generative rendering technology, it is also possible to generate the appearance of scientific phenomena from simulation space to observation space, thereby achieving closed-loop learning across the three spaces of "hypothesis-simulation-observation" and driving the inversion of laws.
Autonomous intelligent unmanned experimental system
The autonomous intelligent unmanned experiment system aims to combine the technological achievements in the fields of AI and robotics with scientific experiments, and improve experimental efficiency and reusability through unmanned, standardized, and large-scale experimental processes.

Among them, the task flow of the unmanned experimental operation platform and intelligent system mainly includes three steps:
*Automatic optimization of solutions
*Autonomous mission planning
*Unmanned experimental operation
At the same time, depending on the operation accuracy,Unmanned experimental operations can be divided into two spatial scales: microscopic and macroscopic.The objects of unmanned experiments at the microscopic scale are usually tiny particles such as living cells and proteins, and fixed experimental platforms are often used. The core issue is to improve the high-precision operation throughput. Unmanned experimental operations at the macroscopic scale focus on the completeness of the experimental process, mainly using mobile robots equipped with mechanical arms to move autonomously between experimental equipment to complete multi-task full-process automated experiments.
In addition, on the basis of the hardware platform, building intelligent system software is another basic issue of the autonomous intelligent unmanned experimental platform, which drives the processes of self-state perception, external environment perception, mobile navigation, instrument positioning, experimental operation planning and control execution through software. Deep reinforcement learning and imitation learning can conduct autonomous learning through the experience trajectory of environmental interaction or the expert teaching trajectory, and build a mapping relationship between observation information and optimal actions.
Large-scale scientific research collaboration
Intelligent scientific facilities support data sharing to facilitate the development and testing of AI models, but a series of measures need to be designed to confirm and protect the intellectual property rights and interests of data developers. In recent years, decentralized science (DeSci) has attracted much attention from researchers. DeSci aims to use Web3 tools, including smart contracts and blockchain, to solve intellectual property issues in scientific research and promote the sharing and circulation of scientific data.
In the intelligent scientific facility architecture proposed by the research team,Blockchain technology provides the foundation for creating a secure and trusted collaborative environment. Federated learning technology can solve the data island problem in a decentralized collaborative environment and ensure data security and scientific research efficiency. Internet swarm intelligence can integrate different scientific research modules into a unified platform to achieve efficient large-scale scientific research collaboration.

Practice and promote the development of AI for Science
As the first stage of the implementation of the concept of intelligent scientific facilities, Shanghai Jiao Tong University has built the AI for Science scientific data open platform, which includes four sub-platforms: scientific computing power base, scientific data, artificial intelligence, and scientific collaboration. Based on this platform, it has developed the "Magnolia Science Model", and released versions 1.0 and 2.0 in July and December 2023, respectively, covering chemistry, fluid, law, cross-science evaluation benchmarks and other fields.
in,The "Magnolia Science Model 2.0" released not long ago includes "Open Source Law (BAI-Law-13B)" and "Chemical Synthesis 2.0 (BAI-Chem 2.0)".
BAI-Law-13B has been pre-trained on a large amount of authoritative data such as the Chinese Internet, open source code, judicial documents, legal documents, and legal books to form the BAI-Law-13B-Base model. On this basis, it integrates legal knowledge memory, legal knowledge understanding, legal knowledge application, and other judicial application scenario supervision and fine-tuning to form the BAI-Law-13B-SFT model. It is reported that in the third-party legal comprehensive evaluation benchmark LawBench In the test, it surpassed all open source Chinese general big models and Chinese legal big models in the test.
BAI-Chem 2.0 can achieve fast and efficient drug molecule design, and can design 30 drug-like and high-affinity molecules per second; the accuracy of the single-step retrosynthesis Top1 on the public data set USPTO test set is 15% higher than the current best in the literature. The yield prediction R² for high-throughput experimental data reached 0.88; the catalyst recommendation accuracy reached 93.7%. At present, BAI-Chem 2.0 has effectively connected wet experiment verification, realized experimental optimization online, and quickly improved the efficiency of chemical experiments.
The early attention paid to AI for Science and the establishment of a corresponding research team are enough to show that Shanghai Jiao Tong University attaches great importance to AI4S, a cutting-edge application of AI. At present, the team has not only produced relevant results, but also continues to cooperate with related companies. It is believed that in the near future, the interdisciplinary and platform-based collaboration in the "intelligent scientific facility concept" will be truly realized.
References:
1.https://news.sjtu.edu.cn/jdzh/20240124/193351.html
2.https://cloud.baidu.com/news/news_85aaa2be-241d-45c2-8aa1-3c8bbcfbd09b