Shenzhen Institute of Advanced Technology of Chinese Academy of Sciences Proposed SBeA, Which Analyzes Animal Social Behavior Based on a Few-shot Learning Framework
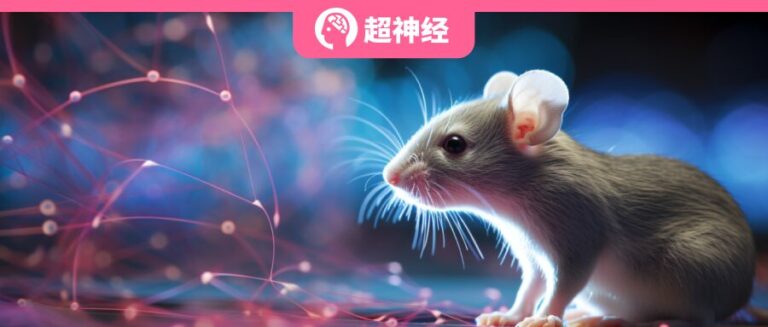
Birds spread their wings, wolves gather around their leader, penguins raise their offspring together... What is the meaning behind the social behavior of animals? Does the progression of reproduction, predation, defense, and establishment of social hierarchy reveal the process of human evolution?
The study of these questions is called animal social behavior, and its ability to precisely quantify, identify, and classify behaviors helps to shed light on the role of brain function and mental disorders in interactions. Animal social behavior models also play an important role in the study of human social disorders, which are highly common symptoms of diseases such as autism, depression, and social anxiety disorder.
In the past, the study and understanding of animal social behavior has been limited by insufficient data annotation. Although deep learning techniques such as multi-animal DeepLabCut, SLEAP, and AlphaTracker provide powerful tools for data annotation, their application in experimental scenarios is limited by the availability of high-quality benchmark datasets. Existing multi-animal pose estimation datasets often fail to cover a wide range of social behavior test models. This limits the breadth and depth of research, as behaviors of different species or in different environments may not be fully considered.
Animal behavior research urgently needs to improve research efficiency and accuracy through technological innovation. Based on this,SBeA (Social Behavior Atlas) came into being and was developed by the Shenzhen Institute of Advanced Technology of the Chinese Academy of Sciences. It can comprehensively quantify the behavior of free-living animals, use a small number of labeled frames (about 400 frames) to estimate the three-dimensional posture of multiple animals, and through a two-way transfer learning strategy, the accuracy of multi-animal identity recognition exceeds 90%.The related results were published in Nature.

Paper link:
SBeA's two main structures: posture tracking and behavior mapping
To comprehensively quantify the behavior of free-living animals, The main tasks of SBeA include 3D pose tracking and social behavior mapping.
3D pose tracking: multi-animal social behavior and identity recognition

The 3D posture tracking process is shown in the figure above:
The team first collected videos of two mice engaging in free social interactions using a camera array, a process that was divided into two phases. The first phase focused on capturing free social interactions between mice, while the second phase aimed to identify and record the identity of each mouse in these interactions, using checkerboard images for calibration.
Then the collected multi-animal contours and single animal postures were annotated in detail and input into the multi-stage artificial neural network for analysis. The output results are shown in Figure d and are divided into three parts:
* AI output video instances, multi-animal postures, and multi-animal identity information;
* 3D reconstruction of video, pose and identity information combined with camera calibration parameters;
* Visual display of 3D pose with identity information.
Behavior Mapping: Low-Dimensional Representation of Time and Space

The process of social behavior mapping is shown in the figure above:
Parallel dynamic decomposition: Raw 3D trajectories of two mice, decomposing them into locomotion (physical movement such as walking, running, jumping, climbing), non-locomotor movement (movement that does not involve changes in the position of the whole body in space, such as tail wagging, ear shaking, or body posture changes), and body distance (physical distance between two or more animals).
In order to gain a deeper understanding of the distribution of features within the social behavior module, the three extracted trajectory features are converted into spatial and temporal representations. The spatial level is captured by low-dimensional embedding distance features in SBeA (as shown on the left side of Figure f), and the temporal level is represented by the social behavior graph (as shown on the right side of Figure f).
Training SBeA: Bidirectional Transfer Learning and Unsupervised Learning
Bidirectional transfer learning: multi-animal identification without labeling
During the free social behavior of animals, animals of the same species often occlude each other, resulting in inaccurate manual identification annotations. To address this challenge, researchers proposed using bidirectional transfer learning in SBeA (Figure a below), that is, the trained multi-animal segmentation model can be transferred to single-animal videos, and the trained single-animal identification model can also be transferred to multi-animal identification, which greatly reduces the workload of manually labeling animal identities.

Training methods using bidirectional transfer learning include:
* Reuse of segmentation model (Figure b): Use a camera array to capture video streams of animals, use the trained VisTR (VIS with transformers) model to segment single animal video instances, and obtain data from multiple angles.
* Single animal identification model training (Figure c): Crop, concatenate and resize the data, and use EfficientNet as the backbone to train a multi-animal identity recognition model.
* Multi-animal segmentation and 3D reprojection (Figure d): Reproject the masks of each camera view, crop, concatenate and resize these data, and input them into the multi-animal identity recognition model.
* Reuse of identity recognition model (Figure e): Reuse the trained single-animal identification model in multi-animal identification.
This process involves complex image processing and data conversion to extract effective information from different perspectives and situations, and ultimately predict the visual results of the animal's identity and 3D posture.
To evaluate the identification performance of SBeA, the researchers recorded the free social behavior of the above-mentioned mice and manually verified the masked reprojected images and 3D postures frame by frame. The results showed that although the recognition accuracy of some single mice was low, the overall accuracy of identifying pairs of mice was higher than 0.85.

Unsupervised learning: Uncovering the structure of social behavior
In unsupervised learning applications, algorithms do not rely on pre-labeled or categorized data; instead, they attempt to discover patterns and relationships directly from the data.
In this study, unsupervised learning decomposes the dynamic structure of behavior by using the dynamic time alignment kernel (DTAK), and uses logical addition to merge the decomposed time points to transform continuous posture trajectories into discrete social behavior modules, as shown in the figure below.

The PAIR-R24M dataset was used to conduct supervised validation of SBeA. The results showed thatThis study successfully quantified the clustering performance of the social behavior module, and SBeA can effectively classify social behaviors.

SBeA: Accuracy + Versatility
Accuracy of SBeA: behavioral studies in Shank3B knockout mice
To explore whether SbeA can detect differences in social behavior at the genetic level, the researchers conducted experiments on mice. Mice with the Shank3B gene knocked out exhibit individual autistic behavior, but the limitations of existing technology make it difficult for us to fully understand their abnormal free social behavior. Through SBeA analysis, the research team found that there were significant differences in social behavior between Shank3B knockout mice (KO) and normal mice (wild-type, WT).
There are three groups of experimental mice: KO-KO, WT-WT, and WT-KO. The results are shown in the figure below. The WT-WT group has more flexible social behavior, the KO-KO group shows more abnormal social behavior than the WT-WT group, and the WT-KO group shows closer social interaction than the WT-WT group.

At the same time, SBeA was used to identify and distinguish subtle social behavior modules between KO and WT mice. Through cluster analysis, these modules were merged into 9 major social behaviors, including curling up, exploratory behavior, peer sniffing, and independent grooming.

The social behaviors of the three groups are as follows:

The WT-WT group (green) showed more allo-regulation and exploratory behaviors, reflecting prosociality.
The KO-KO group (orange) showed more abnormal social behaviors, lower motor ability and social novelty, and these abnormal behaviors may be indicative of antisocial behavior.
The social behaviors observed in the WT-KO group (purple) were mainly peer sniffing and independent grooming. Peer sniffing was more common in the curious WT mice, and independent grooming may be an imitation behavior of KO mice to WT mice.
This result indicates that SBeA can not only distinguish the social behaviors of genetically mutant animals, but also identify subtle social behavioral modules associated with genetic mutations.
Universality of SBeA: Analysis in Different Species and Environments
To assess the generalizability of SBeA across different animal species and experimental settings, the research team conducted studies using birds and dogs.


To increase the difficulty of SBeA testing, the animals in the experiment looked as similar as possible. By mapping the bird and dog data onto the social behavior map, the study identified 34 and 15 social behavior categories for birds and dogs, respectively (Figures c and g), and visualized their typical cases in 3D (Figures d and h).
The results show that SBeA's 3D posture tracking of birds can identify the action of their claws touching their tail feathers, and the 3D posture tracking of dogs can cope with occlusion situations such as lying down.
Advantages of SBeA: a few-shot learning framework
In 2021, Wei Pengfei's team at the Shenzhen Institute of Advanced Technology spent two years using self-developed behavior acquisition equipment to obtain three-dimensional animal movement postures. Based on the language-like hierarchical structure of animal behavior, they proposed a hierarchical animal behavior decomposition model that simplifies continuous and complex behaviors into action modules that can be understood by people. The study has conducted behavioral identification on autism model mice and successfully achieved automatic and accurate identification of their characteristic behavioral abnormalities at the sub-second level.
The SBeA architecture in this article is a further expansion of the "Behavior Atlas", a general animal three-dimensional behavior map framework proposed in 2021, to classify animal social behaviors.
The few-shot learning framework used by SBeA requires only a relatively small number of manually annotated frames for multi-animal 3D pose estimation, enabling unlabeled identity recognition and social behavior classification using unsupervised learning. Such a data generation strategy reduces the reliance on large-scale, widely annotated datasets and can be applied to larger amounts of unlabeled data.
Few-shot learning frameworks, such as transfer learning (leveraging a model pre-trained on a large dataset and then fine-tuning it to fit a smaller dataset for a specific task) and data augmentation (generating new data points from existing data using techniques such as cropping, rotation, or color adjustment to increase the size of the training dataset), have great potential and are becoming increasingly popular in AI research.
References:
1.https://www.nature.com/articles/s42256-023-00776-5
2.https://www.siat.ac.cn/kyjz2016/202401/t20240109_6952837.html