The Accuracy of Early Diagnosis of Parkinson's Disease Has Been Improved to 90.2%. Shenzhen Institute of Advanced Technology and Zhongshan First Hospital Jointly Proposed the GSP-GCNs Model
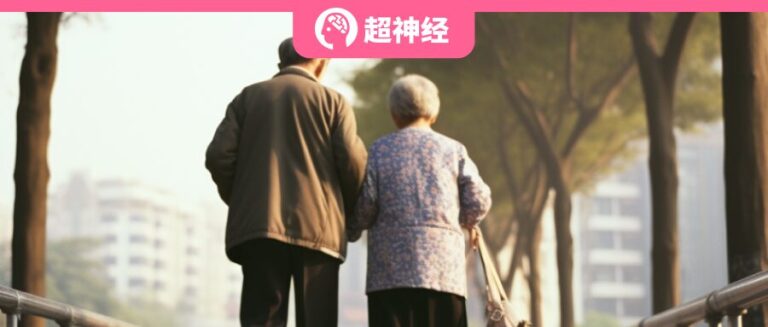
A research team from the First Affiliated Hospital of Sun Yat-sen University and the Institute of Advanced Technology of USTC proposed a deep learning model - graph signal processing-graph convolutional networks (GSP-GCNs), which uses event-related EEG data obtained from specific tasks involving tone regulation to diagnose Parkinson's disease.
Tremor, slow movements, stiff expression... When talking about Parkinson's disease, most people will first think of "hand tremors". However, they don't know that in the middle and late stages of the disease, patients may even have problems such as balance disorders and difficulty turning over. Their lower limbs and head will shake involuntarily, seriously affecting their quality of life.
Data released by the Chinese Rehabilitation Medicine Association in April 2023 showed that there are more than 3 million Parkinson's disease patients in my country, more than a quarter of the world's total number, and about 100,000 new cases are added each year.It is estimated that by 2030, the total number of Parkinson's disease patients in my country will reach 5 million, almost half of the global number of patients.
However, the pathogenesis of Parkinson's disease is still unknown, and only 20% cases can be attributed to specific genetic factors, so early diagnosis faces major challenges.
Resting-state electroencephalogram (EEG) has become an important way to diagnose Parkinson's disease because of its non-invasiveness and ability to capture brain activity with high temporal resolution. In recent years, related studies have begun to combine deep learning with EEG for early diagnosis of Parkinson's disease. However, most of these methods require stable EEG signals to work, and cannot capture the dynamic characteristics of changes in brain activity related to Parkinson's disease.
In view of this,Researchers from the Shenzhen Institutes of Advanced Technology of the Chinese Academy of Sciences and the First Affiliated Hospital of Sun Yat-sen University proposed an interpretable graph signal processing-graph convolutional network (GSP-GCNs) model using abnormal EEG features of speech motor tasks in Parkinson's disease., using the EEG data of the speech fundamental frequency modulation task and mining the neural markers of the large-scale brain functional network of Parkinson's patients, we achieved high-precision intelligent diagnosis of Parkinson's disease.

Get the paper:
https://www.nature.com/articles/s41746-023-00983-9
Reply "Parkinson" in the WeChat public account to get the complete PDF
Dataset: vocalization experiment with 100 participants
The research team invited 100 participants, including 52 people diagnosed withIdiopathic Parkinson's diseasepatients (24 females and 28 males) and 48 sex- and age-matched healthy controls.All participants performed the vocalization experiment.
During the experiment, participants were required to produce vowels for 5-6 seconds with a duration of 200 milliseconds. Each utterance consisted of 4-5 perturbations, withPseudo-randomParticipants uttered the sound 20-25 times in succession for a total of 100 trials.
While the participants performed the vocalization experiment, the researchers used NetStation software to record EEG signals at a sampling frequency of 1kHz using a 64-lead electrode cap connected to a Net Amps 300 amplifier (EGI).
GSP-GCNs model: consists of 4 consecutive components
The GSP-GCNs model framework consists of four modules: graph signal processing module (GSP), graph-network module, classifier and interpretable model.

* First, the GSP module analyzes and processes large-scale EEG networks to identify dynamic connection patterns;
* Secondly, the graph network module captures these connectivity patterns as key features for classification; * Subsequently, the classifier component leverages these extracted features to distinguish Parkinson's disease patients from healthy individuals; * Finally, the interpretability model enhances the interpretability of the framework by providing a global visualization of the learned essential features and aligning them with speech-related EEG micromorphic features.
By adopting this innovative approach, the GSP-GCNs framework aims to provide explanatory information to facilitate the use of deep learning models in Parkinson's disease diagnosis with task-related EEG data.
Model effect: good interpretability
The study proposed four graph network-based models: PCC + GCN, PLV + GCN, PCC + GSP-GCN and PLV + GSP-GCN.
Among them, PCC (Pearson Correlation Coefficient) and PLV (Phase Locking Value) represent different features used to construct brain networks. However, regardless of the feature type,The GSP-GCNs models all show significantly better performance than the GCNs models.
As shown in the figure below, the ROC values of the GSP-GCN model proposed by the institute are all kept below 0.08. This highlights the potential of GSP to improve classification performance by balancing local and global information of single-hop and multi-hop networks through graph aggregation.

By combining local and global information,The GSP-GCNs model proposed in this study has an average classification accuracy of 90.2% for distinguishing Parkinson's disease patients from normal controls, which is significantly higher than other deep learning models by 9.5%.In addition, the GCNs model shows lower computational complexity than all baseline models.

Comparison of classification performance and computational complexity
In addition, the research team analyzed the interpretability of the GSP-GCNs model and revealed its learning results on the difference distribution between the large-scale EEG network and the MS5 microstate EEG topography. In the speech fundamental frequency regulation task, the brain areas with significant differences between Parkinson's patients and normal controls are mainly located in the left ventral premotor cortex, superior temporal gyrus and Broca area, which is highly consistent with the previously discovered brain network of speech movement disorders in Parkinson's disease.
This shows thatThe GSP-GCN model proposed in this study is able to identify unique EEG biomarkers from large-scale networks and provides good interpretability.
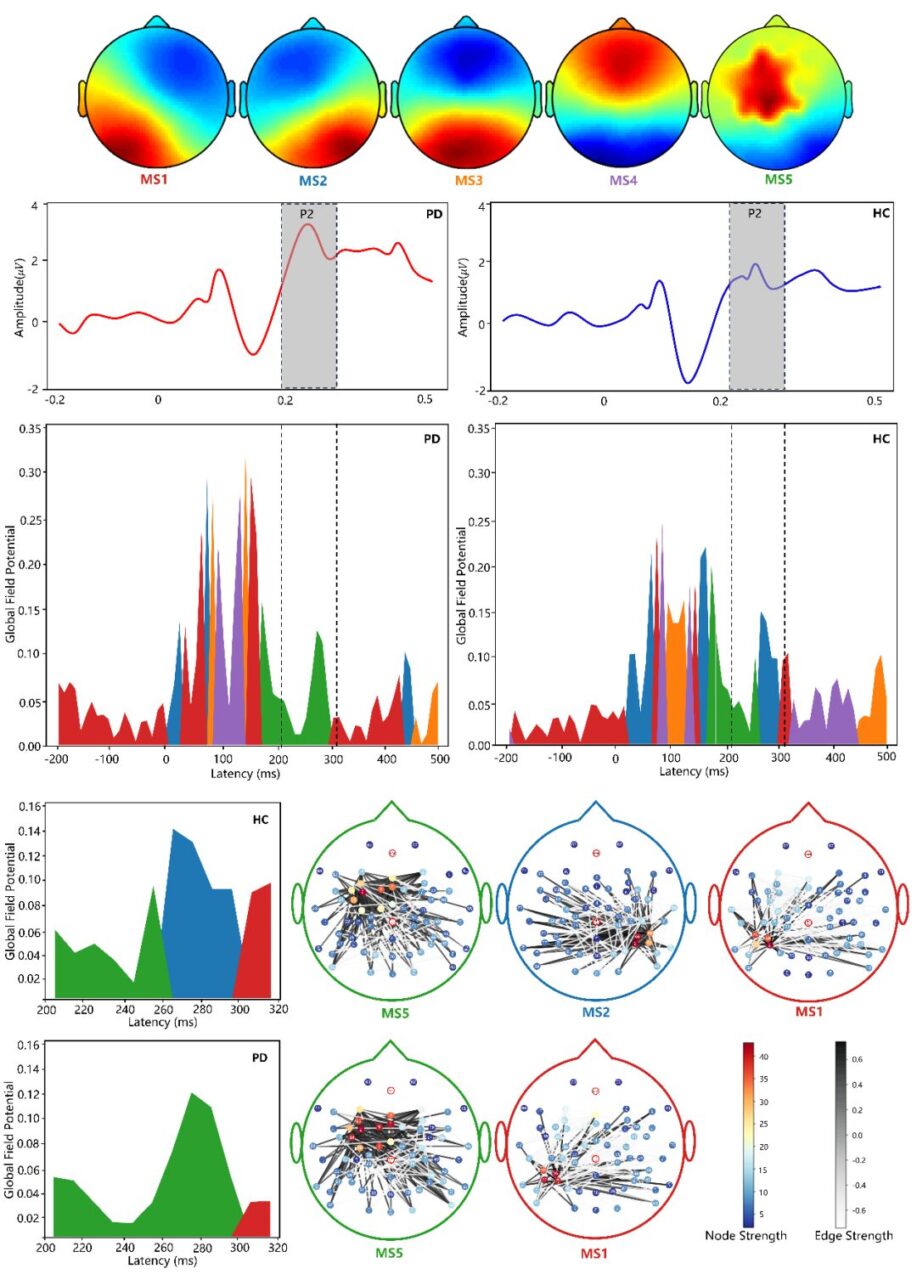
Comparison of ERP microstate distribution
(PD in the upper right corner is the Parkinson's disease group, and HC is the normal control group)
More importantly, the model can effectively learn the differences between brain functional networks in Parkinson's disease patients and normal controls in auditory feedback tasks, providing a new method for analyzing the interpretability of deep learning models applied to disease diagnosis and classification.
AI identifies biomarkers to help diagnose Parkinson's disease
Parkinson's disease onsetParkinson's disease is hidden and progresses slowly, so early diagnosis and timely intervention are extremely important to improve the efficacy. An important way to diagnose Parkinson's disease in the early stage is to identify biomarkers in various behavioral areas, including handwriting patterns, motor functions, gait patterns, and language characteristics.
In recent years, machine learning has become a powerful tool in the field of medical imaging. This technology helps to extract advanced features and patterns from complex neuroimaging data. In addition, due to its excellent multidimensional analysis capabilities, machine learning can also be used for individual classification in the field of medical imaging. The GSP-GCNs model proposed by the joint research team of Zhongshan Affiliated Hospital achieves diagnosis by mining language-related neural markers of Parkinson's patients, and the application of AI deep learning models in the identification of various behavioral areas of Parkinson's disease still has considerable room for development.
Similarly,A research team from the Union Hospital of Fujian Medical University recently developed a machine learning model to predict the risk of freezing of gait in Parkinson's disease patients at the individual level.It can be used to early identify Parkinson's disease patients who are likely to develop delayed freezing of gait, thereby providing valuable guidance for clinicians to prevent and intervene in freezing of gait symptoms in Parkinson's disease patients.
also,The research team from University College London (UCL) trained an AI algorithm using video data from 300 Parkinson's patients and 300 healthy volunteers.It enables it to identify the movement patterns of Parkinson's patients, with an accuracy rate of up to 90% in diagnosing Parkinson's disease.
In the future, we expect that machine learning AI based on neuroimaging can be further applied to help people effectively identify biomarkers in various behavioral areas related to Parkinson's disease symptoms, thereby promoting the further development of early diagnosis of Parkinson's disease.