AI Screening Battery Materials, Academician Ye Siyu of Guangzhou University Developed a Machine Learning Algorithm Model That Can Be Used for P-SOC Material Prediction
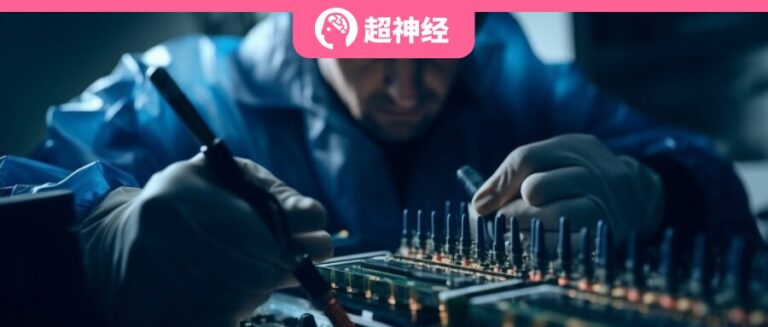
Author: Tian Xiaoyao
Editor: Li Baozhu, Sanyang
Cover image source: Photo Network
Researchers from Guangzhou University established a machine learning model based on the extreme gradient boosting (XGBoost) algorithm, which can be used for the screening of P-SOC air electrodes.
How popular is new energy now? According to data from the China Association of Automobile Manufacturers, from January to November 2023, the market share of new energy vehicles in my country has reached 30.8%, which is due to the continued prosperity of the lithium battery industry. However, in addition to lithium battery technology, solid-state batteries are also the direction pursued by capital and enterprises, and are even hailed as the "end point of electric vehicles." In recent years, automobile and battery companies from China, the United States, Japan and Germany have entered the market one after another. The solid-state battery industry has experienced the initial capital carnival and entered a more pragmatic landing exploration stage.
The high attention of capital and industry has greatly promoted the scientific research progress in related fields, and the development of battery technology fueled by clean energy has also driven the innovation of key materials. Among them, proton conductive solid oxide battery (P-SOC) has the advantages of low temperature operation and low ion conduction activation energy, and has gradually become known to people and received more and more attention.
However, a major obstacle to the development of high-performance P-SOCs is the lack of efficient proton conductor air electrodes. Currently, the widely used air electrode materials for P-SOCs are Co/Fe-based perovskite oxides, but there has been no systematic study on the role of different elements on the B site in Co/Fe-based perovskite oxides.
To solve this problem,Researchers from Guangzhou University have established a machine learning model based on the extreme gradient boosting (XGBoost) algorithm, which can be used for the screening of P-SOC air electrodes.It also expands the application of machine learning in key fuel cell materials.Advanced Functional Materials".

Get the paper:
https://onlinelibrary.wiley.com/doi/10.1002/adfm.202309855
Reply "fuel cell" to get the full paper PDF
Dataset: Control system variables
By comparing the prediction performance of random forest (RF) and XGBoost on the sample data set, this study first screened out 792 samples containing 29 features as a database for studying Co/Fe-based perovskite oxides with different dopants at the B site.
Model architecture: choose the best of two models
The regression prediction task in this study compared two ensemble learning models, namely
* RF, which can build multipleDecision Tree, and integrate the predictions of each decision tree
* XG-Boost, which is based on the gradient boosting decision tree algorithm (as shown below)

Although both the XGBoost model and the RF model show excellent prediction performance, it can be seen from the figure below that when the actual value of the target variable is large, the RF model underestimates its prediction value. In contrast, the prediction value of the XGBoost model is more evenly distributed on both sides of the 1:1 line.This study selected XGBoost as the main model for research analysis.

As shown in the figure below, the machine learning model based on XGBoost uses the element structure as input to screen the P-SOCs air electrodes.

Subsequently, the research team successfully screened out a high-efficiency air electrode material LCN91 for P-SOCs based on machine learning prediction results and density functional theory (DFT) calculations, whose activation energy is comparable to that of well-known air electrodes.
Experimental conclusion: LCN91 has better catalytic activity
Proton conductivity is determined by a combination of proton absorption (PAA), proton diffusion, and proton transfer in the oxide. Experiments have shown that the gap in PAA is positively correlated with the gap in proton conductivity, especially when the proton mobility (i.e., diffusion coefficient) of the two compounds is the same, and PAA can be used directly to measure proton conductivity.
In machine learning, feature importance scores are used to determine the relative importance of each feature when building a prediction model. Combining experimental experience and theory, feature importance ranking can help us verify the constructed machine learning model and improve the interpretability of the model. The figure below shows the feature importance ranking in the XGBoost model PAA prediction.

In order to clearly study the role of doping elements at the B site, this study fixed La at the A site and used Mg, Al, Si, Ca, Sc, Ti, Mn, Fe, Co, Ni, Cu, Zn, Ga, Ge, Sr, Y, Zr, Nb, In, Sn, Sb, Bi, Nd, Sm, Gd, Dy, and Yb as doping elements (B1).
The decrease of ΔE (hydration enthalpy) with the presence of Ni indicates that Ni is beneficial to the hydration reaction in LCN91 because the smaller the ΔE, the easier the hydration reaction proceeds.

In summary,LCN91 is more conducive to hydration reaction.This is consistent with the results predicted by the machine learning model, where the PAA of LCN91 is higher than that of LCN82. At the same time, LCN91 oxide has better catalytic activity.
Experimental evaluations have shown that LCN91 is considered to be a good P-SOC air electrode, however, the thermal expansion coefficient (TEC) of LaCoO3 family oxides is very high compared to the electrolyte material, which may cause the electrode to fall off from the electrolyte during long-term operation, thereby shortening the battery life.
Although LCN91 still has areas that need improvement and enhancement, this study verified the excellent proton conductivity of LCN91 by combining machine models and DTF calculations, and also provided guidance for the future development of new air electrode oxides with high proton conductivity and appropriate thermal expansion coefficients.
Academician Ye Siyu: Hydrogen fuel cells are just the starting point
At present, "carbon peak and carbon neutrality" has become a global consensus. Hydrogen energy, as a clean, efficient and renewable energy with large reserves, provides an important way for decarbonization in various industries. Proton conductor solid oxide cells (P-SOC) have the advantages of low raw material cost, small environmental impact and high theoretical electrolysis efficiency. They are the research focus and hotspot in the field of green hydrogen production by electrolysis of water.
As an internationally recognized leading expert in fuel cell electrocatalysis and catalyst layer/MEA design,Academician Ye Siyu has long been committed to various aspects of proton exchange membrane fuel cell research and development.He is currently a member of the Canadian Academy of Engineering, a professor at the School of Chemistry and Chemical Engineering of Guangzhou University, director and chief scientist of the Huangpu Hydrogen Energy Innovation Center, and vice chairman and chief technology officer of Hongji Chuangneng Technology (Guangzhou) Co., Ltd.
As the first national specialized and innovative "little giant" enterprise in the field of fuel cell membrane electrode research and development and production to settle in Guangzhou, Hongji Chuangneng is committed to the large-scale localization and industrialization of high-performance membrane electrodes (MEA) for proton exchange membrane fuel cells, providing low-cost, high-performance membrane electrode core components to domestic and foreign fuel cell manufacturers, solving the problem of China's long-term reliance on foreign technology for core fuel cell materials.
It is understood thatHongji Chuangneng has independently developed the first fully automated MEA packaging production line and produced automotive fuel cell membrane electrodes with independent intellectual property rights that are in line with the international leading level.It broke the technological monopoly of foreign companies in the industry and filled the gap in the domestic industry. In 2022, Hongji Chuangneng's annual membrane electrode shipments reached 1.7 million pieces.
The International Hydrogen Energy Commission pointed out that the demand for green hydrogen is expected to grow to 75 million tons by 2030. In the next 10 years, the global demand for renewable low-carbon hydrogen will increase by 50%. Thanks to the "friendship" between traditional energy companies and hydrogen fuel cells, the development of hydrogen fuel cells will no longer be limited to pure fuel cell vehicles, but will eventually be closely related to the entire energy system.