8 Times Faster Than the Best Technology: Hou Tingjun Et Al. From Zhejiang University Proposed ResGen, a 3D Molecular Generation Model Based on Protein Pockets
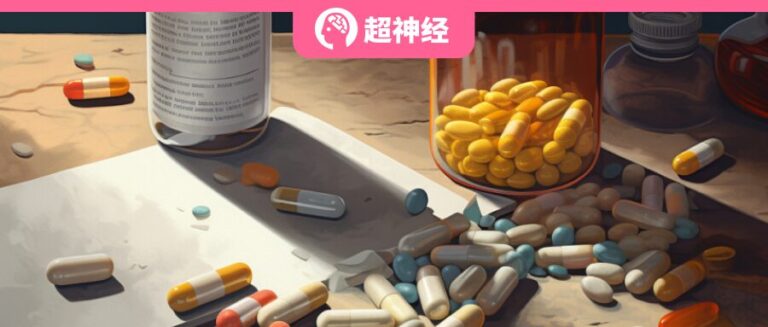
Author: Binbin
Editor: Li Baozhu, Sanyang
The research team of Zhejiang University and Zhijiang Laboratory proposed a 3D molecular generation model based on protein pockets - ResGen. Compared with the previous optimal technology, the speed is increased by 8 times and it successfully generated drug-like molecules with lower binding energy and higher diversity.
In the past, the discovery of innovative drugs often relied on ancient formulas or accidental events in experiments, such as penicillin. Over the years, advances in molecular biology and computational chemistry have enabled the drug design model to shift from blind screening to rational design.
Despite this, drug development and design is still a multi-link process with long links and high costs. Improving the efficiency of each link has great value. In recent years, with the widespread application of technologies such as AI and big data, AI-assisted drug design has become more mature in experiments. AI is upgrading and reforming multiple links of drug development to improve efficiency and quality.
Among them, high-quality molecular generation models can effectively improve the efficiency of lead compound discovery. At present, most molecular generation work uses the ligand-based method (LBMG), but this method has many limitations, such as the inability to consider the interaction mode between molecules and targets. Therefore, researchers are increasingly paying attention to the structure-based molecular generation (SBMG, structure-based molecular generative) method, that is, generating corresponding molecules based on the target structure.
Professor Hou Tingjun and Professor Xie Changyu from Zhejiang UniversityZhijiang LaboratoryChen Guangyong and his team proposed a 3D molecular generation model based on protein pockets - ResGen.The model adopts a parallel multi-scale modeling strategy, which can capture higher-level interactions between protein targets and ligands and achieve higher computational efficiency.
The molecule generation process is formulated as global autoregression and atomic autoregression to better account for the geometry of protein pockets. The results show that the molecules generated by ResGen have more reasonable chemical structures and better target affinity than existing state-of-the-art methods.

Get the paper:
https://www.nature.com/articles/s42256-023-00712-7
Reply "3D molecule generation" in the WeChat public account to get the complete PDF
Dataset: The sequence similarity between the training set and the test set is less than 40%
The training dataset used in this study is CrossDock2020, which is used for protein-small molecule interaction research, especially for evaluating the binding ability of molecules to protein pockets.
The initial data of this dataset contains more than 22 million protein-ligand pairs. To ensure that the sequence similarity between the training set and the test set is less than 40%, the researchers screened and obtained about 100,000 protein-ligand pairs. The test set contains 100 protein pockets.
Dataset link:
ResGen Model: Two Hierarchical Autoregressive
The ResGen model formulates the molecule generation problem conditioned by protein pocket awareness as an autoregressive problem at two scales, the global scale and the atomic component scale.Among them, global autoregression means that each atom generated by ResGen is based on the molecular fragments and protein pocket structures generated in the previous steps; atomic autoregression generates the newly added atomic coordinates and topology in turn.
ResGen can decompose the complete molecular generation process into step-by-step sampling, thereby achieving the generation of the entire molecule in an autoregressive manner. In addition, in order to better capture higher-level interactions and reduce computational costs, the research team introduced parallel multi-scale modeling technology in this three-dimensional conditional generation problem.

* Figure A illustrates: During the process of molecule generation, the growth points are gradually confirmed, atoms are added (global autoregression), the positions of atoms are confirmed, and then edges are added (atomic autoregression).
* Figure B shows pockets and reference molecules represented as atomic features (vector) and atomic coordinates (scalar).
* Figure E shows the molecular generation process. The gray dot cloud in i represents the newly generated atoms with position information; the green dot cloud in ii is the newly generated atoms with the atom type added. The red circle represents the focal atom (growth point) at each step, and the number is the probability of each atom becoming a growth point.
Effect verification: better than the current optimal model
All along,There are two widely used test indicators for the 3D molecular generation model based on protein pockets: whether the model has learned the characteristic topological distribution of ligands in different protein pockets (i.e., the molecular graph distribution of the target), and the distribution of ligands in the pockets.Geometric distribution(i.e. the rationality of atomic position and conformation).
To this end, the research team conducted a series of evaluations of ResGen and existing state-of-the-art models.
For the first test metric, the team evaluated the binding energies and drug-like properties of the molecules designed for the targets in the test set and real therapeutic targets.
For the second test indicator, the team designed a conformational rationality experiment and analyzed the interaction pattern between the protein and small molecules.
Generate molecules on the test set: Evaluate model generalization ability

The comparison results showed that the molecules generated by ResGen outperformed the GraphBP and molecules generated by Pocket2Mol.
* GraphBP:A 3D graph neural network is used to extract semantic information, and then the atoms are generated sequentially through an autoregressive flow model. A 3D molecule that binds to a given protein is generated by placing atoms of specific types and positions one by one into a given binding site.
* Pocket2Mol:It is used to model the chemical and geometric features of 3D protein pockets and adopts a new efficient algorithm to sample new 3D drug candidates based on pocket conditions.
As shown in the figure above, Vina Score represents the binding energy between the generated molecule and the corresponding protein target. This indicator can reflect to a certain extent whether the model senses the chemical environment in the pocket.
ResGen's performance on the Vina Score means thatResGen has a better chance of generating molecules that bind more tightly to the target.The research team believes that this may be because ResGen uses multi-scale modeling to characterize the structure, because this structure is more conducive to capturing higher-level interactions between protein pockets and ligands (such as fragment-residue interactions).
In addition, whether an organic compound can be promoted as a drug candidate depends not only on the strength of its interaction with proteins, but also on its drug-likeness and synthesizability. Therefore, drug-likeness indicators such as QED, SA, Lipinski and LogP are included in the evaluation. ResGen scored the highest in SA and Lipinski indicators.This suggests that ResGen has a greater potential to generate easily synthesizable drug-like ligands for unrecognized protein pockets.
Molecular Generation Against Real Targets: Evaluating Performance in Realistic Scenarios
In order to evaluate the performance of the model in real drug design scenarios, the research team used AKT1 and CDK2 (Cyclin-Dependent Kinase 2) in protein kinase B as cases, sorted out their target structures and ligand compounds with experimental activity, and randomly selected a batch of inactive small molecules as negative controls.

The figure above shows the binding affinity distribution of each group of molecules. The more the distribution is biased to the left, the greater the absolute value of the binding energy and the higher the affinity. The results show that the molecules generated by ResGen (green) not only have higher scores than the negative control (Random) and other molecules generated by the most advanced existing models, but the overall distribution is even slightly better than Active.
Bond length distribution experiment: assessing conformational plausibility
In the conformational rationality experiment, the research team calculated the root mean square deviation between the directly generated molecular conformations and those generated by traditional conformational software, and compared the bond length distribution between the generated samples and the training molecules.
Among the 7 bond lengths,ResGen performs best among the 5 bond lengths, significantly outperforming GraphBP (approximately 10 times)Compared to the other two existing state-of-the-art models, ResGen generates smoother conformations, highlighting its strong ability to capture the complex geometric distribution inside protein pockets.

AlphaFold Predictive structural analysis: Assessing model sensitivity to interactions
To verify whether ResGen has successfully learned the interaction patterns that depend on the target geometry and the model's sensitivity to protein-small molecule interactions, the research team generated two groups of molecules based on the X-ray crystal structure and the AlphaFold predicted structure, and compared the structural features of the two groups of molecules.

Molecules generated based on crystal structures and AlphaFold predicted structures. The white ligands are co-crystal ligands, and X Å is the RMSD between the predicted structure and the true structure after alignment. The white spheres in the first column represent possible binding sites.
The conformation predicted by AlphaFold "closes" the pocket existing in the crystal conformation, causing the model to be unable to generate a complete molecule at the original pocket position, but instead to generate small fragments in the newly formed cavity, indicating that ResGen's molecule generation process is sensitively dependent on the given protein pocket.

The pocket formed in the AlphaFold predicted conformation is less different from the crystal pocket, but the model can still capture this change. The ResGen generated molecule occupies more of the cavity structure in the AlphaFold predicted conformation (as shown in the red circle in the figure).
This experiment demonstrates the sensitivity of ResGen to target structure and also suggests the importance of correct protein structure for the SBMG strategy.
「AlphaFold2 infers protein structureDetailed tutorial:
https://openbayes.com/console/public/tutorials/m6k2bdSu30C
AlphaFold protein structure dataset:
https://openbayes.com/console/public/datasets/ETTgyY1oZat/1/overview
Click "Read original text" to enter with one click, without downloading the dataset
Hou Tingjun: Dedicated to the research of core issues in computer-aided drug design
Molecular generation is a typical multi-objective optimization task. The molecules we generate not only need to have good affinity, but also good drugability, low toxicity, high synthesizability, etc.
——Hou Tingjun
In the traditional drug discovery process, drug innovation has problems such as long R&D cycle, high investment, and high risk. The discovery and optimization of lead compounds is the most challenging stage in the entire drug discovery process, which requires overcoming the huge chemical space of compounds (which may reach the order of 10 to the 60th power); in addition, the screening, optimization and evaluation process of lead compounds is very complicated.
Through deep learning and big data analysis, AI can efficiently process and interpret large-scale bioinformatics data, discover patterns and associations hidden in huge data sets, improve the accuracy of identifying potential drug targets, and accelerate the process of drug screening and design.
Aiming at the field of AI-assisted drug development,Professor Hou Tingjun and his team have been conducting cutting-edge interdisciplinary research on core issues in computer-aided drug design.And achieved a series of valuable results, such as:
* In the field of molecular docking and virtual screening, we proposed a new scoring method for protein-small molecule interactions based on graph representation learning, IGN, and a high-throughput molecular docking framework based on deep learning KarmaDock wait.
* In terms of intelligent molecule generation and optimization, we proposed the ligand-based multi-constraint molecule generation method MCMG and the 3D molecule generation method SurfGen based on topological surfaces and geometric structures.
*In terms of molecular drugability and safety assessment, we proposed the toxicity prediction method MGA based on the multi-graph attention model and the drugability prediction software system ADMETlab2.0.
In addition, Professor Hou Tingjun’s team also developed an AI model interpretability method SME based on substructure masking, which proposed a solution to the interpretability of AI models.
Although the great value of AI in drug development is becoming increasingly prominent, as an emerging research, there may still be corresponding challenges in its actual implementation, and these will become the key research directions in the future.
In this regard, Professor Hou Tingjun said,How to effectively improve the predictive ability of AI-based property prediction methods, the predictive ability of AI-based scoring functions in virtual screening, and the prediction accuracy of key drugability parameters and toxicity endpoints will be the directions and challenges that need to be focused on in the field of AI-assisted drug discovery in the future.
References:
https://mp.weixin.qq.com/s/cxpbeGmrHULcWsbVbvQmJA