Defeating 8 Human Planners: Tsinghua Team Proposes a Reinforcement Learning Urban Spatial Planning Model
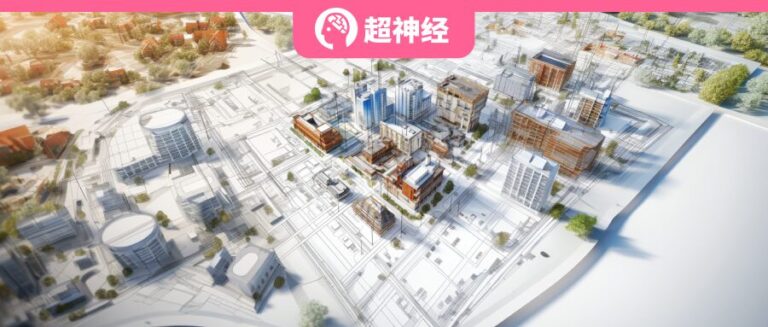
Author: Binbin
Editor: Li Baozhu, Sanyang
The research team of Tsinghua University proposed a reinforcement learning model and method for urban community spatial planning, and realized an urban planning process in which human planners collaborate with artificial intelligence algorithms, providing a new idea for the automated planning of smart cities.
Cities not only carry people's eager expectations for a peaceful and happy life, but are also an important foundation for supporting various economic activities. From the agricultural age to the industrial age, and then to today's digital age, people have never stopped improving the comfort and safety of cities. In this process, the importance of urban planning has become increasingly prominent.
In recent years, the concept of "15-minute city" has received more attention with the goal of being more livable. The core of the concept is that residents can walk or ride to basic service facilities within 15 minutes, which reflects people's expectations for efficient spatial layout in urban communities. However, due to the diversity of urban geographical space, urban land use layout and road planning is a very complex and difficult task, and has always been highly dependent on the experience of professional planners.

In view of this,Tsinghua University’s Urban Science and Computing Research Center and School of Architecture collaborated across disciplines to innovatively propose a method for urban community spatial planning based on deep reinforcement learning, the proposed AI model can learn urban planning from massive data, continuously optimize spatial efficiency, and ultimately achieve a planning level that exceeds that of human experts.
Paolo Santi, a research scientist at the MIT Senseable City Lab, said:This successfully demonstrated the feasibility of humans and AI collaborating to complete spatial layout planning tasks, providing rich research directions for urban science.Currently, the research has been published in Nature Computational Science.

Paper address:
https://www.nature.com/articles/s43588-023-00503-5
GitHub link:
https://github.com/tsinghua-fib-lab/DRL-urban-planning
Reply "urban planning" in the WeChat public account to get the full paper
Combining millions of planning data, 3 key concepts are proposed
In response to the new community planning of "15-minute city", the research team of Tsinghua University conducted millions of planning in virtual urban environment.We propose a city adjacency graph, a deep reinforcement learning urban planning model, and a human–artificial intelligence collaborative framework.
Urban adjacency graphs: Overcoming the challenges of diverse and irregular neighborhoods
Convolutional neural networks are suitable for tasks with regular inputs, such as Go and chip design, but urban communities are diverse and irregular, and convolutional neural networks do not have a significant advantage in similar tasks. In order to overcome this problem,The research team proposed a city adjacency graph to describe the topological structure of urban geometry.
Among them, urban geographical elements are nodes, including plots, roads, intersections, etc., and spatial adjacency relationships are edges. The use of graph models can give a unified representation to any form of urban communities, thereby giving full play to the efficient search capabilities of deep reinforcement learning algorithms in huge action spaces and realizing the intelligent layout of urban community land and roads.

(b) Graphical representation of road layout
Deep reinforcement learning models: reducing the action space
By modeling the city adjacency graph, urban spatial planning is restated as a sequence of choices made on a dynamic graph, where the action of selecting edges and nodes at each step in the graph space causes the graph to evolve accordingly. This is another major challenge - the huge action space. The action space of a medium-sized community may exceed 4000 to the power of 100 (4000 possible actions per step, a total of 100 steps for community spatial planning), which makes the modeling process cumbersome and labor-intensive.
To reduce the action space, the researchers constructed two strategies and a value network.The policy network is used to assist the AI Agent in selecting the location of land and roads, and the value network predicts the quality of spatial planning based on the concept of the "15-minute city". By sampling from the policy network and using the value network to estimate the planning quality, the action space is greatly reduced.

(a) Graph neural network state encoder
(b) Land use strategy network
(c) Community Planning Value Judgment Network
(d) Road layout strategy network
(e) Schematic diagram of the Markov decision process on the community planning map
In order to obtain an effective representation of urban geographical elements,The researchers further developed a state encoder based on a graph neural network (GNN), which is shared between the value network and the policy network, exploits message passing and neighbor aggregation on the urban continuity graph to capture the spatial relationships between land, road segments and intersections.
Human-artificial intelligence collaborative: 3,000 times faster
Considering the complexity of urban planning methods,The research team proposed a workflow for AI and human planners to collaborate, where human planners focus on concept prototyping and the model is used to do the heavy and time-consuming planning work.
This workflow is superior to the workflow completed entirely by humans in both objective planning indicators and subjective blind tests by 100 professional planners, and is 3,000 times more efficient in time. This shows that human planners can benefit from the collaborative workflow of AI and humans. By designing a collaborative workflow between human planners and artificial intelligence, the work efficiency of human planners can be greatly improved, and community planning schemes of different styles can be efficiently generated.
New method beats 8 human planners
Experiments show that the community planning scheme based on this research method can significantly improve the various indicators of the "15-minute city".Under the same initial conditions and planning constraints, our method significantly outperforms state-of-the-art algorithms and human planners, improving the objective metric of spatial efficiency by more than 48.6%.
Especially when using existing real communities as initial conditions,The model can generate land use transformation plans that significantly improve residents' accessibility to various facilities by more than 18.5%.

(ae) Planning schemes of different algorithms
(a) Centralized heuristic method (b) Decentralized heuristic method (c) Genetic algorithm (d) Multilayer perceptron reinforcement learning algorithm (e) The method proposed by the research (fg) Comparison of 15-minute city performance indicators: f service indicators, g ecological indicators


Design of 2 community space plans and their corresponding space performance
(ah) Spatial plans generated by human planners.
(i) A spatial plan generated by the proposed method.
(j) Comparison of the service and eco-efficiency performance of the two.
(k) Comparison of the accessibility of services for five basic residential needs between the two designs.
The results show that the method proposed by the research team beat 8 professional human planners in terms of service and ecological indicators.Specifically, the proposed method improves the service efficiency by 13.64% and 19.52% respectively compared with the best and average planner performance. In terms of ecological indicators, the efficiency is improved by 15.38% and 59.65% respectively.
In addition, in terms of accessibility to five basic residential demand services (i.e., education, medical care, shopping, work, and entertainment), the method proposed by the research team still achieved a more balanced performance, with 3/5 ranking the highest relative to all human planners.
Human-machine integration: the future of urban planning
On the one hand, urban planning needs to deal with a complex ecosystem that includes population, organization, environment, and technology. On the other hand, it is at the downstream of technological application, and the industry's digital upgrade started late.
In recent years, advances in AI have brought new opportunities to urban planners. Using AI algorithms and data analysis techniques, planners can gain a deeper understanding of the complex systems that make up modern cities, helping them make more informed decisions about issues in urban planning. However, it is worth noting that urban planning is not a game that only requires choosing land use and road locations, but a complex interaction between multiple stakeholders.
At present, AI models involved in urban planning are mainly guided by static indicators. Although they can generate community plans with high spatial efficiency, planning an entire city is a more complex task that requires considering diverse goals including economic growth and resident health. In addition, land ownership, public access, urban segregation, and renovation all require attention.
We hope that in addition to helping human planners speed up the spatial layout process, AI models can also bring broader benefits to other participants. For example, by introducing customized options into the model, and then building a public platform to promote residents and developers to participate in the planning process. The framework proposed by the Tsinghua University research team this time shows the possibility of a higher degree of participation by all participants, which is also an important step towards a more transparent and inclusive city.