The Curse of "super Bacteria" May Be Broken. MIT Uses Deep Learning to Discover New Antibiotics
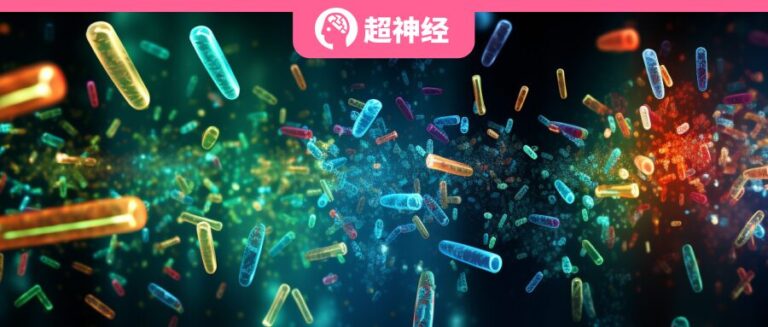
Author: Add Zero
Editor: Li Baozhu, Sanyang
MIT uses graph neural network Chemprop to identify potential antibiotics that specifically kill Acinetobacter baumannii.
There are all kinds of microorganisms in nature, such as Mycobacterium tuberculosis (causing tuberculosis) and Vibrio cholerae (causing cholera), which seriously endanger people's health. In human history, there is almost no way to fight these pathogenic bacteria except relying on the human immune system. It was not until 1928 that the discovery of penicillin gave humans a powerful weapon to defeat pathogenic bacteria for the first time.
However, the widespread use of antibiotics has also brought about a huge crisis - antibiotic resistance (AMR). According to statistics from the World Health Organization (WHO), in 2019, about 1.2 million people died from bacterial infections exacerbated by antibiotic resistance (AMR), which is higher than the number of deaths caused by AIDS. The misuse of antibiotics has led to the emergence of certain "super bacteria", which has become an important clinical cause of disease in the 21st century. To solve this problem, it is urgent to develop new antibiotics.
For specific bacteria, deep learning models can improve the accuracy of predictions on the efficacy and safety of compounds, effectively reducing the time and resource consumption of laboratory experiments and clinical trials, which is crucial for the discovery of effective and safe antibiotics.
To this end, researchers from MIT developed a deep learning method to discover antibiotics, using the graph neural network Chemprop to identify potential antibiotics from large chemical libraries and discovered a new class of antibiotics.They were able to specifically kill an important drug-resistant bacterium, Acinetobacter baumannii, and the related paper has been published in Nature.

The paper has been published in Nature
Paper address:
https://www.nature.com/articles/s41586-023-06887-8
Follow the official account and reply "Discover Antibiotics" to download the full paper
Experimental Method: Deep Learning with Graph Neural Networks
Dataset: Multiple compounds
Initial screening:The study initially screened 39,312 compounds and analyzed their antibiotic activity and human cytotoxicity characteristics.
Expanded forecast:To further expand the prediction scope, the models were tested on 12,076,365 compounds, of which 11,277,225 were from the Mcule database and 799,140 were from the Broad Institute database.

Molecular weight distribution of 39,312 compounds
Algorithm training: Training with graph neural networks
The graph neural network Chemprop was used to train the 39,312 screened compounds to predict their antibiotic activity and human cell toxicity. The training process is as follows.

The molecule represents:A graph-based molecular representation is generated from the SMILES (Simplified Molecular Input Line Entry Specification) string of each compound using RDKit.
Feature vector generation:Generate feature vectors for each atom and bond, including atomic features (such as atomic number, number of bonds, positive charge, etc.) and bond features (such as bond type, conjugation, ring membership, etc.).
Information transmission:Implement a key-based message passing convolutional neural network, update the key's message, pass it through the neural network layers and apply non-linear activation functions.
Model output:After a fixed number of information transfer steps, the model summarizes the messages of the entire molecule and predicts the activity of the compound, such as antibiotic activity, cytotoxicity, or activity in changing the proton motive force, through a feedforward neural network.
Optimization measures:This includes adding additional molecular-level features, selecting the best performance parameters using hyperparameter optimization, and enhancing the robustness of the model through ensemble learning.
Model architecture: ensuring the effectiveness and safety of compounds
Antibiotic activity models
The researchers predicted the antibiotic activity against Staphylococcus aureus (S. aureus) in culture medium at a compound concentration of 50 μM, distinguishing between activity and inactivity using the 80% normalized growth inhibition cut-off. A total of 10 graph neural network models were trained, validated, and tested on the same 80% – 20% training dataset.
The results show thatThe Chemprop model with RDKit features showed superior predictive ability, identifying 512 active compounds out of 39,312 compounds.

Comparison of deep learning models for predicting antibiotic activity
Human cytotoxicity models
The researchers used 39,312 compounds to screen for toxicity against human hepatoma cells (HepG2), human primary skeletal muscle cells (HSkMCs), and human lung fibroblasts (IMR-90). After treatment with each compound at 10 μM for 2-3 days, cell viability was assessed and compound activity was classified using the 90% cell viability cut-off.
Similarly, 10 Chemprop model sets were trained, validated, and tested. The comparison results are shown in the following figure:

Comparison of deep learning models for predicting human cytotoxicity
The results showed that 3,341 (8.5%), 1,490 (3.8%) and 3,447 (8.8%) compounds were toxic to HepG2 cells, HSkMCs and IMR-90 cells, respectively. Among the 512 active antimicrobial compounds screened in the previous step, 306 were non-toxic to these three cell types.
In summary, although this model has certain limitations compared to the antibiotic activity model, it balances the effectiveness of the drug and its harmlessness to the human body, demonstrating the potential of using advanced computational methods in drug discovery.
Experimental results: Screening and identification of antibiotics
Refining and scaling up models: screening and visualization of the entire chemical space
In this phase of the research, the focus was on refining and applying models to identify potential antibiotic compounds in a large chemical space and evaluate their cytotoxicity. The researchers retrained 20 Chemprop models to predict antibiotic activity and cytotoxicity in HepG2, HSkMC, and IMR-90 cells, and the improved models were applied to the prediction of 12,076,365 compounds.
Compound screening
Antibiotic activity screening: 3,004 compounds with antibiotic prediction scores exceeding 0.4 were screened from the Mcule database; 7,306 compounds with scores exceeding 0.2 were screened from the Broad Institute database.
Human Cell Toxicity Screening:
Compounds with a cytotoxicity prediction score below 0.2 were retained, resulting in the final screening of 3,646 compounds (1,210 from the Mcule database and 2,436 from the Broad Institute database)—accounting for 0.03% of all evaluated compounds.

Compound screening
a: Computer simulation filtering program b: Antibiotic activity and cytotoxicity prediction of HepG2, HSkMC and IMR-90 cells
Visualization of chemical space
Morgan fingerprints were used as molecular representations, and the t-distributed stochastic neighbour embedding (t-SNE) method was used to visualize the chemical space.
As shown in the figure below, the t-SNE visualization reveals a clear difference between hits (compounds that passed the screening) and non-hits (compounds with low antibiotic prediction scores).

t-SNE analysis of compounds
Further screening: identification of two potent compounds
Among 3,646 compounds, two compounds (No. 1 and No. 2) were screened out that showed high activity against S. aureus and good selectivity against human cells. The performance of these two compounds under various test conditions, especially the growth inhibition ability in serum-containing culture medium, was excellent and worthy of further study.
The study of these compounds demonstrated that the structural classes predicted by deep learning models can effectively guide experimental screening to discover new antibiotic candidates.
Screening process
Compound screening: Compounds containing PAINS and Brenk alerts for possible reactivity, mutagenicity, or unfavorable pharmacokinetic properties were eliminated from the initial set of 3,646 hits, resulting in a screen of 2,209 compounds.
Structural screening: Compounds with structures different from those in the training set were further screened, using a maximum Tanimoto similarity score ≤ 0.5 as a preliminary cutoff and excluding compounds containing a β-lactam ring or a quinolone bicyclic core, resulting in 1,261 compounds.
Identification of effective compounds
Growth inhibition test:Among the nine hits compounds related to rational groups G1-G5, four compounds (44%) were found to be active against Staphylococcus aureus (S. aureus) with a minimum inhibitory concentration (MIC) ≤32 μg/ml.
Structural classes and efficacy: These effective compounds were related to rational groups G1, G2, and G5, among which two compounds (No. 1 and No. 2) from group G2 were confirmed to be active. These two compounds met both Lipinski's rule and Ghose's criteria, indicating that they have good oral bioavailability and drug-like properties and are worthy of further study.

The two compounds screened
Further study: Properties of the two compounds
Through in-depth mechanism studies and in vitro and in vivo experiments, compounds 1 and 2 showed potential as novel antibiotic candidates. They are not only effective against multidrug-resistant strains, but also have a low tendency to develop drug resistance and good safety.
These findings suggest that these two compounds could serve as a promising chemical series for antibiotic drug development.
Mechanism of action and drug resistance
Common structure:Compounds 1 and 2 share the N-[2-(2-chlorophenoxy)ethyl]aniline core structure, which was predicted to be the basic structure for antibiotic activity.
Growth inhibition assay:In time-kill experiments against Staphylococcus aureus and Bacillus subtilis, these two compounds showed antibiotic activity similar to that of vancomycin but with lower bactericidal potency.
Drug resistance studies:In experiments with antibiotic-resistant Staphylococcus aureus strains, the two compounds only slightly increased their minimum inhibitory concentrations (MICs), suggesting that they may have a different mechanism of action than common antibiotics.
Development of drug resistance:After 30 days of continuous culture, the MICs of these two compounds showed almost no changes, showing a low trend of resistance development.
Effect against multi-drug resistant bacteria
Broad resistance:Both compounds showed activity against 40 different bacterial species, including vancomycin-resistant Enterococci, with median MICs of 4 and 3 μg/ml, respectively.
Effectiveness against dormant bacteria:Both compounds also showed activity against stationary cells of Bacillus subtilis.
Toxicology, Chemical Properties and Efficacy
Safety studies:Both compounds showed good safety in in vitro experiments, including non-hemolysis, non-binding to metal ions, non-genotoxicity, chemical stability, and safety in mice.
In vivo efficacy experiments:In the mouse Staphylococcus aureus skin infection and thigh infection models, compound 1 showed significant antibacterial activity.

In vivo efficacy of compounds
Deep learning: a powerful tool to combat antibiotic resistance
Researchers have been exploring effective and easy-to-promote methods to combat antibiotic resistance for many years. In this process, the emergence of deep learning has provided researchers with new ideas for solving problems. The application value of deep learning in combating antibiotic resistance lies in:
Beyond Traditional Antibiotic Discovery Approaches:Traditional antibiotic discovery methods often rely on known active structures, which limits the scope of new drug discovery. Deep learning methods can identify new compounds that are structurally different from traditional antibiotics and may be effective against current resistant strains.
Personalized and Precision Medicine:Deep learning can be used to analyze the genetic and phenotypic characteristics of specific pathogens, thereby facilitating the development of personalized antibiotics for specific pathogens or types of infection.
The road ahead is long and arduous, but the road will eventually be reached. The application of deep learning in drug development is still in a relatively early stage and may face challenges such as data quality and interpretability. However, as an important line of defense for humans against bacteria, related research is of great significance and I believe it will continue to move forward with the support of technological iterations.
References:
https://www.nature.com/articles/s41586-023-06887-8