Aftershock Intensity Prediction Capabilities Upgraded, Nature Published an Article Certifying That the Performance of the Neural Network-based Model Is Better Than the Traditional Model
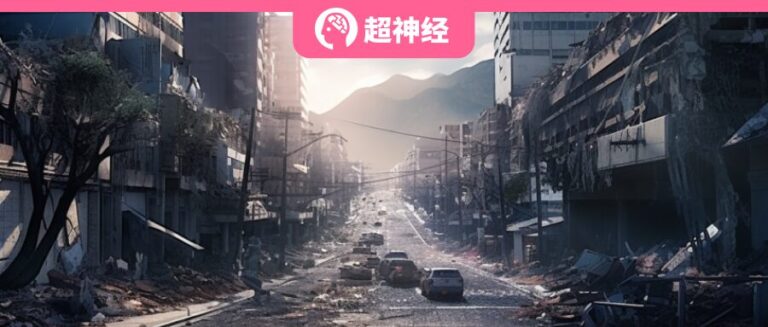
Author: Li Baozhu
Editors: Li Weidong, xixi, Sanyang
The occurrence of earthquakes involves many variables, and "prediction" is challenging, but significant progress has been made in predicting the number and intensity of aftershocks.
At 23:59 on December 18, 2023, a magnitude 6.2 earthquake occurred in Jishishan County, Linxia Prefecture, Gansu Province, with a focal depth of 10 kilometers. As of 06:00 on the 19th, 275 aftershocks had been monitored locally. Coincidentally, a magnitude 7.6 earthquake occurred on the Noto Peninsula in Ishikawa Prefecture, Japan on the afternoon of January 1, 2024. As of 06:00 local time on the 2nd, 129 aftershocks with an intensity of more than 2 degrees had occurred.
(A 2 on Japan's earthquake intensity scale means many people in quiet buildings could feel the shaking.)
Although not as violent as the main earthquake, the power of aftershocks should not be underestimated. On the one hand, the magnitude of aftershocks of strong earthquakes is often not small; on the other hand, the secondary damage caused by aftershocks may further destroy the already extremely fragile buildings, causing larger areas to collapse.
In addition, frequent aftershocks may cause structural instability of the mountain, and if there is precipitation, it may also cause secondary geological disasters such as landslides and mud-rock flows. However, aftershocks are usually unpredictable because it is difficult to determine how the main shock will remotely trigger fault zones in other areas in the short period of time after the earthquake.
In fact, people have never stopped exploring how to achieve earthquake prediction, especially the data processing and reasoning capabilities demonstrated by technologies such as AI and big data, which have provided more flexible problem-solving ideas and have made gratifying progress. Nature reporter Alexandra Witze previously published a report in Nature introducing the potential of machine learning models in predicting earthquake aftershocks and their intensity.

Original link:
https://www.nature.com/articles/d41586-023-02934-6
Innovation of prediction models powered by neural networks
Disasters often occur in a split second and are difficult to counter with human power, so people are more eager to predict when an earthquake will occur so that they can evacuate dangerous areas in advance. Although earthquake prediction with a clear time and location is still difficult to achieve, aftershock prediction has become possible with the help of deep learning, which will undoubtedly help post-disaster evacuation and reduce casualties to a great extent.
Just as large language models need to be trained with millions of words, sentences, paragraphs, etc., training earthquake prediction models also requires a large amount of past earthquake data to predict the probability of aftershocks. However, researchers have found that it is not easy in practice to clearly predict the detection indicators needed by rare large earthquakes. In the past few years, seismologists have used machine learning to discover small earthquakes that have never been discovered in past earthquake records, thereby enriching existing data and providing new material for the second round of machine learning analysis.
The prediction model currently used by the United States Geological Survey (USGS) predicts possible earthquakes based on the magnitude and location of past earthquakes.Currently, three papers have adopted prediction methods based on neural networks to better capture the complex laws of earthquake occurrence.
first,Kelian Dascher-Cousineau, a geophysicist at the University of California, Berkeley, and colleagues tested their model on data from thousands of earthquakes that occurred in Southern California between 2008 and 2021. The model outperformed the standard model in predicting the number of earthquakes that would occur over a rolling two-week period. In addition, the model can better capture the range of possible earthquake magnitudes, thereby reducing the chances of unexpected large earthquakes.
Currently, the earthquake evolution simulation method widely used in the industry is the ETAS (epidemic-type aftershock sequence) model.
Specifically, the study tested the properties of the main shock, as well as how the background (depth, plate boundary type, etc.) and source (radiated energy, source size, etc.) affect the number of aftershocks, and introduced the neural-temporal point processes model into the standard earthquake prediction framework.

Source: Kelian Dascher-Cousineau's GitHub profile https://keliankaz.github.io/academic-profile/
Secondly,Samuel Stockman, an applied statistician at the University of Bristol in the United Kingdom, has also developed a model based on neural point processes that performed well when trained on earthquake data from central Italy in 2016-2017. The machine learning model performed even better when the researchers reduced the magnitude of the earthquakes in the training set.

The study has been published in Earth's Future. The study shows that the neural point process has better prediction performance for low-magnitude data than the earthquake aftershock statistical model ETAS (Epidemic-Type Aftershock Sequence), and has a fast training speed.
Paper address:
https://agupubs.onlinelibrary.wiley.com/doi/10.1029/2023EF003777
at last,Yohai Bar-Sinai, a physicist at Tel Aviv University in Israel, led a team to develop an encoder-decoder-based model called FERN (Forecasting Earthquake Rates with Neural networks). When tested on 30 years of earthquake data in Japan, the model also performed better than the standard model.

Model architecture
As shown in the figure above, the model input is encoded by the neural network to generate a latent representation of the constructed state, which is then passed to the decoder network. The advantage of this idea is that different data sources and patterns can be naturally incorporated and added to the model through specific data source encoders. In addition, the same encoded state can be used as input to multiple prediction heads (decoders) for different prediction tasks. This research has been published in Nature.
Paper address:
https://www.nature.com/articles/s41598-023-38033-9
Smart Earthquake——AI Real-time Earthquake Monitoring System
People's expectation of "preventing disasters before they happen" is a huge driving force for the continuous upgrading of earthquake early warning systems, but we must first make it clear thatWhat has been put into use and is being continuously iterated is the earthquake early warning system, not earthquake prediction.
Although there is only one letter difference between the two, the technical difficulty and actual effects are very different.Earthquake early warning,It means that after an earthquake occurs, before the seismic waves spread to the fortified area, an alarm is issued several seconds to tens of seconds in advance to inform people to take emergency measures, thereby reducing casualties;Earthquake prediction,It refers to the forecast of earthquake events that have not yet occurred but may occur.
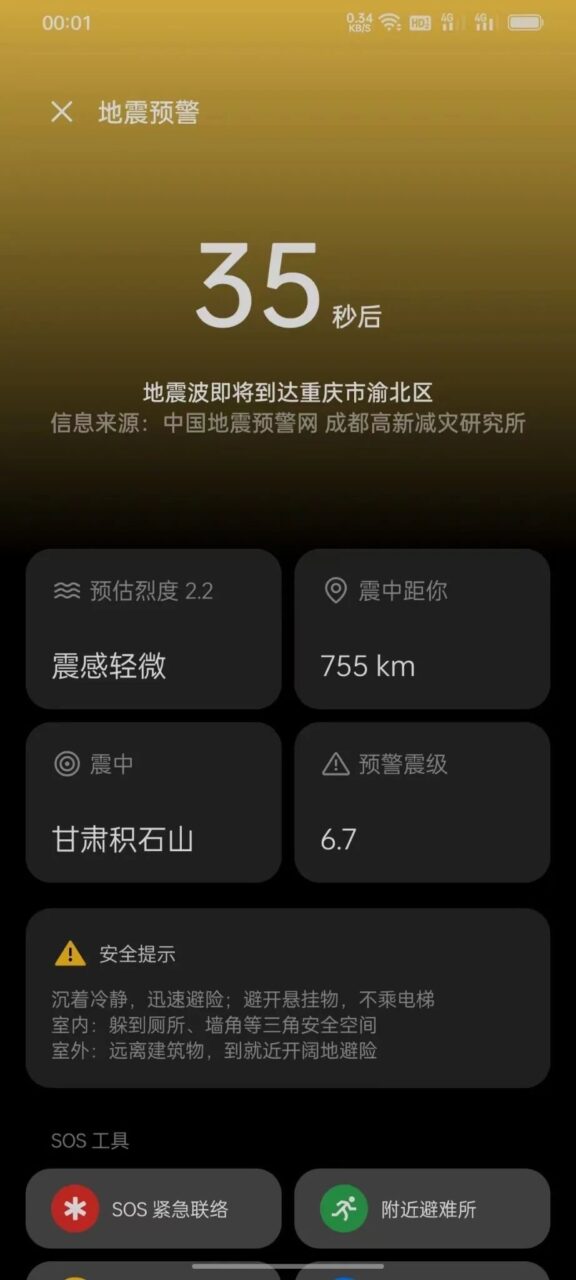
Image source: Weibo @冲鋒号角
From the ground composition of different regions to the types of interactions between seismic plates and the way seismic waves propagate through the earth, the assessment of earthquakes involves many variables, and people need to fully understand all factors to make accurate judgments. Therefore, "prediction" is not easy, but "early warning" is not.
Looking at "earthquake early warning" from the perspective of the model, first of all, it is necessary to ensure the timely input of earthquake data, and secondly, to quickly and accurately process the earthquake data that is occurring, and then infer the rupture direction and speed of the fault, and finally transmit it to the disaster-stricken area in real time through communication means. This process is comparable to racing with death, and it can only buy a few seconds of escape time. Data shows that when an earthquake occurs, if the earthquake warning information can be received 3 seconds in advance, casualties will be reduced by 14%, and if it can be received 10 seconds in advance, casualties will be reduced by 39%.
At present, earthquake early warning systems have been deployed in many places around the world, but the time to receive early warning information is mostly 3-10 minutes. Japan's REIS earthquake early warning system can calculate the location and magnitude of an earthquake 5 seconds after receiving the seismic wave signal, and estimate the source mechanism of the earthquake rupture about 2 minutes later; the automatic rapid reporting system of the United States Geological Survey takes 3-5 minutes to report earthquake information; my country released the world's first artificial intelligence earthquake "real-time" monitoring system - Smart Earthquake in 2021.
The system was developed by Professor Zhang Jie's team at the University of Science and Technology of China in collaboration with the China Earthquake Administration. It can obtain the three elements of an earthquake - time (time of earthquake occurrence), space (location of the epicenter), and strength (magnitude of the earthquake) - within 1 second, and obtain the epicenter mechanism, i.e. the direction and speed of the fault rupture, thereby achieving real-time, automatic detection and release of earthquake information.

In terms of its principle, "Smart Earthquake" is mainly based on deep learning capabilities. It uses millions of earthquake data collected in the database and combines them with seismological theory to quickly process data on earthquakes that are occurring.
What is more important is how to issue early warning information as soon as possible after monitoring earthquake data.
In this Gansu earthquake, users in Xining and Chengdu near Jishishan County received mobile earthquake warnings, ranging from 120 seconds to 240 seconds. Many netizens are amazed at the power of domestic mobile phones, but in fact, the credit should be attributed to the China Earthquake Early Warning Network jointly built by the Chengdu High-tech Disaster Reduction Research Institute and the China Earthquake Administration, which provided early warning information. Among them, the early warning network issued a warning to Linxia City, 56 kilometers away from the epicenter, 12 seconds in advance, and issued a warning to Lanzhou City, 110 kilometers away from the epicenter, 29 seconds in advance.
According to Wang Tun, director of the Chengdu High-tech Disaster Reduction Research Institute, the principle of earthquake early warning technology is that electromagnetic waves propagate much faster than seismic waves. Before the seismic shear waves reach the warning target area, the faster propagating electromagnetic waves are used to issue warnings to the potentially affected areas. At present, domestic mobile phones such as Huawei and Xiaomi have been connected to the earthquake early warning function of the China Earthquake Early Warning Network. This is also the 80th time that the China Earthquake Early Warning Network has warned of destructive earthquakes.
Humans are so insignificant in the face of natural disasters, but with the continuous upgrading of technologies such as AI, big data, and the Internet of Things, we are also arming ourselves with technology and becoming increasingly powerful. With the accelerated iteration of emerging technologies, people will continue to optimize the earthquake early warning system and work towards the goal of "predicting earthquakes"!