AGU of the United States Releases AI Application Manual, Clarifying 6 Major Guidelines
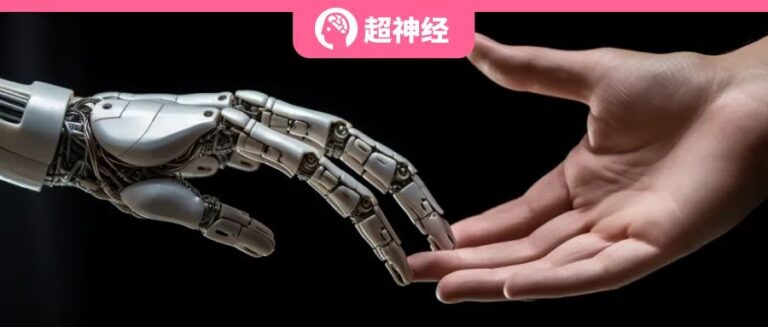
Explosive AI applications: risks and opportunities coexist
In the fields of space and environmental science, AI tools are increasingly being used in areas such as weather forecasting and climate simulation, energy and water resource management, etc. It can be said that we are experiencing an unprecedented explosion of AI applications, and we need to think more carefully about the opportunities and risks involved.
The American Geophysical Union (AGU)’s follow-up report further reveals the widespread application of AI tools.From 2012-2022, the number of papers mentioning AI in their abstracts grew exponentially, highlighting its huge impact in weather forecasting, climate simulation, resource management, and more.

AI-related paper publication trends
However, while AI releases powerful power, it also brings potential risks. Among them, undertrained models or improperly designed data sets may lead to unreliable results and even potential harm. For example, using tornado reports as an input data set, the training data may be biased towards densely populated areas because more weather events are observed and reported there. As a result, the model may overestimate tornadoes in urban areas and underestimate tornadoes in rural areas, causing harm.
This phenomenon also raises important questions: when and to what extent can people trust AI and avoid possible risks?
With support from NASA, AGU convened experts to develop a set of guidelines for the application of artificial intelligence in space and environmental sciences., focusing on the ethical and moral issues that may exist in AI applications, not only limited to the specific field of space and environmental science, but also providing guidance for all-round AI applications. Related content has been published in "Nature".

The paper was published in Nature
Paper link:
https://www.nature.com/articles/d41586-023-03316-8
Follow the official account and reply "Guidelines" to get the full PDF of the paper
Six guidelines to help build trust
At present, many people are still on the sidelines regarding the trustworthiness of AI/ML. To help researchers/research institutions build trust in AI, AGU has established six guidelines:
In order to preserve the original meaning, the author attaches the translation and the original text together.
Guidance for researchers
1. Transparency, Documentation and Reporting
Transparency and comprehensive documentation are essential in AI/ML research. Not only should data and code be made available, but also participants and how problems were solved, including dealing with uncertainty and bias. Transparency should be considered throughout the research, from concept development to application.
2. Intentionality, Interpretability, Explainability, Reproducibility and Replicability
When conducting research using AI/ML, it is important to consider intentionality, explainability, reproducibility, and replicability. Open scientific methods should be prioritized to improve the explainability and reproducibility of models, and the development of methods to explain AI models should be encouraged.
3. Risk, Bias and Effects
Understanding and managing potential risks and biases in datasets and algorithms is critical to research. By better understanding the sources of risk and bias, and ways to identify these issues, adverse outcomes can be managed and responded to more effectively, maximizing public benefit and impact.
4. Participatory Methods
In AI/ML research, it is important to adopt inclusive design and implementation approaches. Ensuring that people from different communities, fields of expertise, and backgrounds have a voice, especially for communities that may be affected by the research. Co-production of knowledge, participation in projects, and collaboration are essential to ensuring that research is inclusive.
Guidance for academic organizations (including research institutes, publishers, associations and investors)
5. Outreach, Training and Leading Practices
Academic organizations need to provide support to all sectors to ensure that training on the ethical use of AI/ML is provided, including to researchers, practitioners, funders, and the broader AI/ML community. Scientific associations, institutions, and other organizations should provide resources and expertise to support AI/ML ethics training and educate societal decision-makers on the value and limitations of AI/ML in research so that responsible decisions can be made to reduce its negative impacts.
6. Considerations for Organizations, Institutions, Publishers, Societies and Funders
Academic organizations have the responsibility to take the lead in establishing and managing policies related to AI/ML ethical issues, including codes of conduct, principles, reporting methods, decision-making processes, and training. They should clarify values and design governance structures, including cultural construction, to ensure that ethical AI/ML practices are implemented. In addition, it is necessary to implement these responsibilities across organizations and institutions to ensure that ethical practices are implemented throughout the field.
More detailed advice on AI applications
1. Watch out for gaps and biases
When it comes to AI models and data, be wary of gaps and biases. Factors such as data quality, coverage, and racial bias can affect the accuracy and reliability of model results, which may bring unexpected risks.
For example, some regions may have far better coverage or veracity of environmental data than others. Regions with frequent cloud cover (such as tropical rainforests) or areas with less sensor coverage (such as the poles) will provide less representative data.
The richness and quality of data sets often favor wealthy areas, ignoring disadvantaged groups, including communities that have long been discriminated against. And these data are often used to provide recommendations and action plans for the public, businesses and policymakers. For example, in health data, dermatology algorithms trained on white data are less accurate in diagnosing skin lesions and rashes in black people.
Institutions should focus on training researchers, reviewing the accuracy of data and models, and setting up professional committees to oversee the use of AI models.
2. Develop ways to explain how AI models work
When researchers use classic models to conduct research and publish papers, readers usually expect them to provide access to the underlying code and related specifications. However, researchers are not yet explicitly required to provide such information, resulting in a lack of transparency and explainability in the AI tools they use. This means that even if the same algorithm is used to process the same experimental data, different experimental methods may not accurately replicate the results. Therefore, in publicly published research, researchers should clearly record how to build and deploy artificial intelligence models so that others can evaluate the results.
The researchers recommend conducting comparisons across models and dividing data sources into comparison groups for examination. The industry urgently needs further standards and guidance to explain and evaluate how AI models work so that the results can be evaluated with a level of statistical confidence.
Currently, researchers and developers are working on a technology called explainable AI (XAI), which aims to enable users to better understand how AI models work by quantifying or visualizing outputs. For example, in short-term weather forecasting, AI tools can analyze large amounts of remote sensing observation data obtained every few minutes, thereby improving the ability to predict severe weather disasters.
Clearly explaining how outputs were reached is critical to assessing the validity and usefulness of predictions. For example, when predicting the likelihood and extent of fires or floods, such explanations can help humans decide whether to alert the public or use the outputs of other AI models. In the geosciences, XAI attempts to quantify or visualize the characteristics of input data to better understand the model outputs. Researchers need to check these explanations and ensure they are reasonable.

Artificial intelligence tools are being used to assess environmental observations
3. Forge partnerships and foster transparency
Researchers need to focus on transparency at every stage: sharing data and code, considering further testing to ensure replicability and reproducibility, addressing risks and biases in methods, and reporting uncertainties. These steps require more detailed descriptions of methods. To ensure comprehensiveness, research teams should include experts in using various types of data, as well as inviting community members who provide data or may be affected by the findings. For example, one AI-based project combined traditional knowledge of the Tharu people of Canada with data collected using non-indigenous methods to identify areas best suited for aquaculture. (See go.nature.com/46yqmdr).

Aquaculture Project Pictures
4. Sustain support for data curation and stewardship
Requirements for data, code, and software reporting across interdisciplinary research need to meet the FAIR principles: findable, accessible, interoperable, and reusable. To build trust in AI and machine learning, recognized, quality-assured datasets are needed, with bugs and solutions made public.
The current challenge is data storage. For example, the widespread use of general repositories may lead to metadata issues, affecting data source tracking and automatic access. Some advanced disciplinary research data repositories provide quality inspection and information supplementation services, but this usually requires human and time costs.
In addition, the article also mentions issues such as funding for repositories, limitations on different repository types, and insufficient demand for domain-specific repositories. Academic organizations, funding agencies, etc. should provide sustained financial investment in supporting and maintaining appropriate data repositories.

Researchers are increasingly choosing common data repositories
5. Look at long-term impact
As we study the widespread application of artificial intelligence and machine learning in science, we must look at the long-term impact and ensure that these technologies can reduce social gaps, enhance trust, and actively include different opinions and voices.
What China says about AI use
"How to use AI and how to use AI well" is also a hot topic in my country's AI field in recent years.
In the eyes of this year's two sessions, artificial intelligence is one of the most active areas of digital technology innovation, with generative AI (AIGC), large-scale pre-trained models, and knowledge-driven AI are releasing new opportunities in the industry, and we need to seize the "time window" of technological development.
Lei Jun, founder, chairman and CEO of Xiaomi Group, proposed encouraging and supporting the scientific and technological innovation industry chain, promoting the planning and layout of the bionic robot industry; accelerating the formulation of data security standards for the entire life cycle of automobiles to guide industrial development; building an automobile data sharing mechanism and platform to promote the sharing and use of automobile data.
360 founder Zhou Hongyi hopes to create China's "Microsoft + OpenAI" combination, lead the research on large model technology, and create an open innovation ecosystem for open source crowdsourcing.
Academician Zhang Boli suggested setting up a major special project for biopharmaceutical manufacturing, supporting the research and development of key technologies and equipment for intelligent pharmaceutical manufacturing, and encouraging the development of biopharmaceutical equipment.
It can be seen that the representatives and members of the two sessions are very optimistic about the artificial intelligence track. In addition to empowering technology, we also hope that AI can better help the development of enterprises and society under the principle of building trust and using it with caution.
References:
https://www.nature.com/articles/d41586-023-03316-8
https://doi.org/10.22541/essoar.168132856.66485758/v1