Reinventing the Wheel or Reinventing the Wheel? Apple Open-sources MLX, a Custom Machine Learning Framework for Its Own Chips
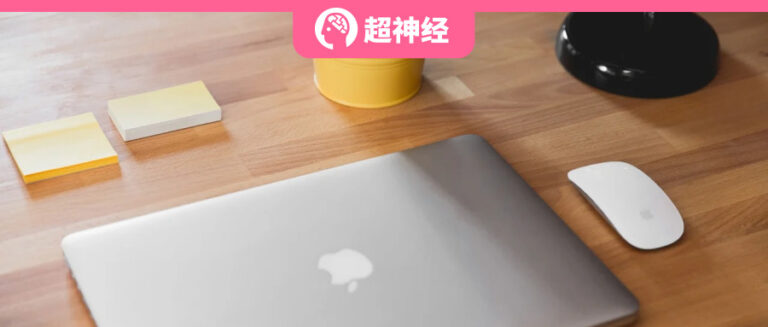
December 6, Beijing time,Apple machine learning research center (Apple machine learning research) open source MLX on GitHub.

A custom-made machine learning framework for Apple chips
MLX is a machine learning framework designed specifically for Apple chips.It aims to support efficient training and deployment of models on Apple chips while ensuring user-friendliness.
MLX is designed with a simple concept, based on frameworks such as NumPy, PyTorch, Jax, and ArrayFire. Its key features include:
* Familiar API:MLX Python API NumPy Very similar, and MLX also has a full-featured C++ API. In addition, MLX also has similar `mlx.nn` and `mlx.optimizer` These higher-level packages, much like the PyTorch API, simplify building complex models.
* Composable function conversion:MLX features composable function transformations for automatic differentiation, automatic vectorization, and computational graph optimization.
* Lazy computation:Computations in MLX are performed lazily, and Arrays are created only when they are needed.
* Dynamic graph construction:The computational graph in MLX is built dynamically, changing the shapes of function parameters does not slow down compilation, and debugging is simpler and more intuitive.
* Multi-device support:Operations can be run on any supported device (currently CPU and GPU are supported).
* Unified Memory:The unified memory model is a significant difference between MLX and other frameworks. Arrays in MLX are stored inShared MemoryYou can perform operations on any supported device without moving data.
According to official GitHub Repo Introduction, MLX has updated several popular tutorials, including:
* Transformer language model training
* Generate large-scale text using LLaMA and LoRA Perform finetuning
* Generate images using Stable Diffusion
* Speech recognition using OpenAI Whisper
For more information, please visit:
https://github.com/ml-explore/mlx/tree/main/examples
Reinvent the wheel from scratch or reinvent the wheel?
There are two completely different opinions about the MLX released by Apple.
✅ Representatives of the affirmative side:

MLX, a PyTorch-style neural network architecture optimized for Apple chips, may be Apple's most important move to date in the field of AI open source.

Yann LeCun, 2018 Turing Award winner and Meta's chief AI scientist, also praised the work.

Hope to put some pressure on Nvidia to reduce prices, improve efficiency, and launch better consumer-grade GPUs

Apple’s commitment to open source AI is commendable, and MLX is a revolution in deep learning on Apple chips.

Cool! When will there be a high-quality model like GPT-4 that can run locally? MLX brings great imagination.
❌ Representatives of the opposing side:

They could have worked with @PyTorchTeam to release a PyTorch backend optimized for Apple silicon!!
Now developers have to do two jobs, supporting this and building a wrapper to support both PyTorch and MLX!

How can this be deployed? Unless it is compatible with frameworks supported by Nvidia, AMD, etc., MLX will be a harm to the machine learning ecosystem (no MacBook, no machine learning)

Apple, reinventing the wheel again

A picture is worth a thousand words
GitHub Issue: I will discuss it later, but I will fix the bug first.
Talk is cheap. Show me the code.
While trying to install and run MLX from a MacBook terminal,We found that the official installation code reported an error.Several engineers in the MLX GitHub Repo also encountered similar problems.

Reported installation error under this issue
Whether MLX can become a practical framework for developers to train and deploy models on Apple devices,We just want to ask, can we fix the bug first? !
Waiting online, pretty urgent.