Li Honglin's Research Group at East China University of Science and Technology Develops Macformer to Accelerate the Discovery of Macrocyclic Drugs
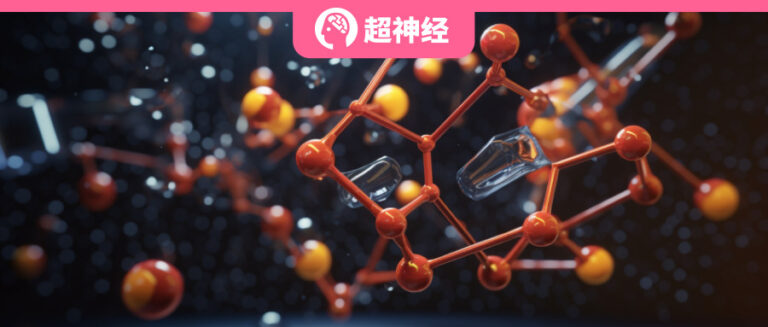
Macrocyclic compounds are small molecules or peptides composed of more than 12 atoms. Compared with other small molecule compounds, macrocyclic compounds have many advantages in structure and performance, and are therefore regarded as potential therapeutic drugs for various targets.
Macrocyclic analogs based on medicinal chemistry synthesis are a major source of macrocyclic drugs. However, due to the lack of synthesis methods, high synthesis difficulty and few reference materials, the development of macrocyclic drugs is rarely pursued.
To this end, Li Honglin's research group at East China University of Science and Technology developed Macformer based on Transformer. Macformer successfully macrocyclized the acyclic drug feizotinib to obtain a new compound with stronger efficacy, providing a new method for drug development.
Author | Xuecai
Editor | Sanyang
The history of macrocyclic drugs
Macrocycles are small molecules or peptides composed of more than 12 atoms.This type of compound has a high molecular weight and a large number of hydrogen bond donors, and has stronger affinity, selectivity and pharmacological properties.Macrocyclic drugs have been considered as potential therapeutic drugs for various targets, such as kinases, proteases and G-protein-coupled receptors.

In addition to natural macrocyclic drugs, analogues based on pharmaceutical chemical synthesis are also a major source of macrocyclic drugs.By macrocyclizing known acyclic drugs, new macrocyclic drugs can be directly and effectively obtained., and achieve the desired pharmacological properties. However,Due to the lack of synthetic methods and the high difficulty of synthesis, macrocyclic drugs still receive little attention in drug design.
Currently, the macrocyclization of linear molecules mainly relies on empirical inferenceMoreover, even if the literature presents the final synthesis results, the synthesis and inference process of the drug is always vague. The opaque and non-standard synthesis process has raised the threshold of the industry and hindered the development of macrocyclic drugs.
Although deep learning has shown great potential in different stages of drug development,But training neural networks requires a lot of dataConsidering that there are less than 90 clinically approved macrocyclic drugs, there is no research on the macrocyclization of drugs using deep learning algorithms.
to this end,Li Honglin's research group at East China University of Science and Technology developed Macformer based on Transformer, in order to achieve the macrocyclization of linear molecules. They expressed the same compound using different simplified molecular linear input specifications (SMILES) to achieve data expansion.
Subsequently, taking Fedratinib, a JAK2 inhibitor approved by the U.S. Food and Drug Administration (FDA), as an example, Macformer was used to macrocyclize it to obtain a new macrocyclic compound.This compound has better selectivity and pharmacokinetic properties, so the dose required is lower than that of fuzotinib. This result has been published in "Nature Communication".
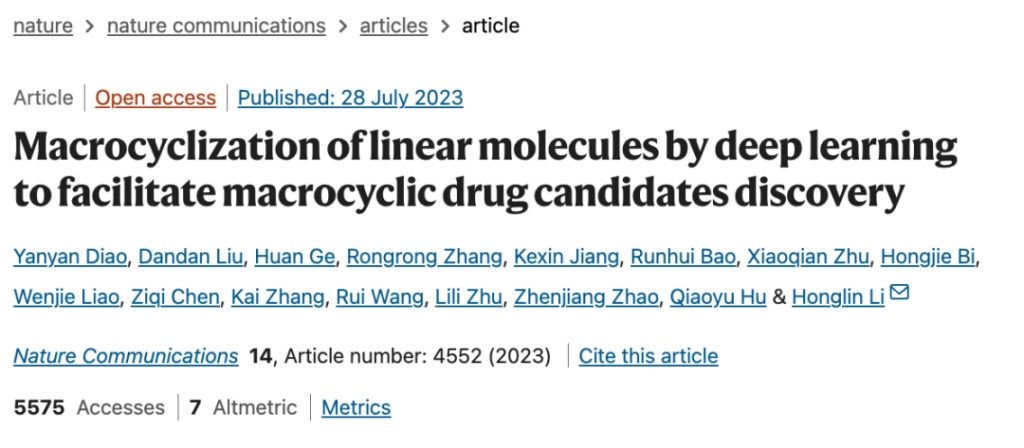
Get the paper:https://www.nature.com/articles/s41467-023-40219-8
Follow the official account and reply "macrocyclic drugs" to get the full paper PDF
Experimental procedures
Dataset:Data augmentation of the ChEMBL dataset
First, the researchers collected 18,357 biologically active macrocyclic compounds from the ChEMBL database and screened them. Then, they traversed any two chemical bonds of the macrocyclic compounds, split their linkers, and found the corresponding acyclic compounds.Finally, a total of 237,728 pairs of macrocyclic-acyclic compounds were obtained as the data set for this study..
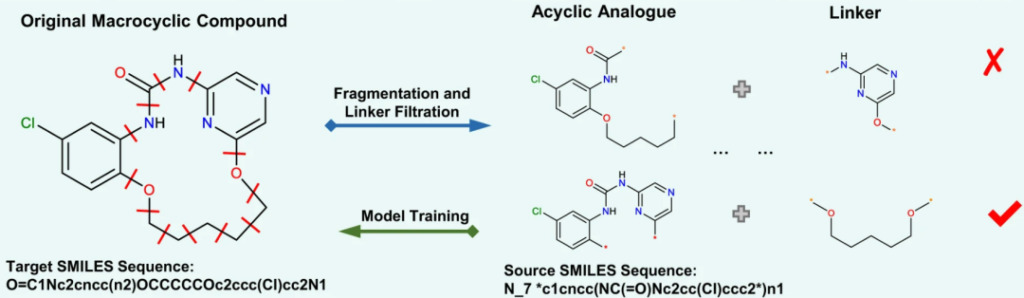
Any compound has a specific SMILES expression. However, recent studies have shown thatModel training using a set of random SMILES expressions that are chemically identical but syntactically different, which can significantly improve the performance of deep learning models. The researchers compared the data expanded by 2, 5, and 10 times with the original data, and all data showed good convergence after 50,000 steps.
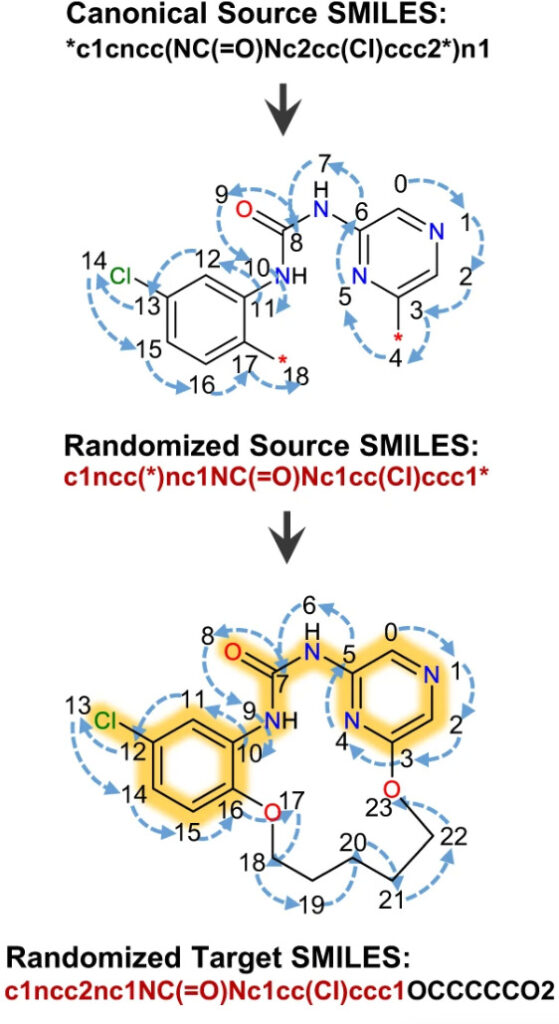
Model Architecture:Transformer Encoding and Decoding
Macformer is based on the Transformer architectureThe SMILES sequences of both input and target compounds are embedded into a trainable matrix and positionally encoded using sine and cosine functions.
The embedding matrix of the input compound is fed into the encoder to generate a latent representation to initialize the encoding process. Each encoder layer consists of a multi-head attention layer and a position feed-forward network.
The ultimate goal of Macformer is to minimize the gap between the predicted sequence and the corresponding target sequence through the cross entropy loss function and output the predicted macrocyclic compound..

Comparative Study:ChEMBL datasets
The researchers compared Macformer with a non-deep learning model called MacLS. After inputting an acyclic compound, both output a macrocyclic analog.The chemical validity, novelty and uniqueness of the macrocycles will be used as evaluation criteria for model performance.
Compared with the original dataset, the 2-fold expansion of the dataset improved the overall performance of the model, especially in terms of recovery rate (96.09% vs. 54.85%), effectiveness (80.34% vs. 66.74%) and connector novelty (58.91% vs. 40.56%), while further expansion of the dataset did not continue to improve the performance of the model.
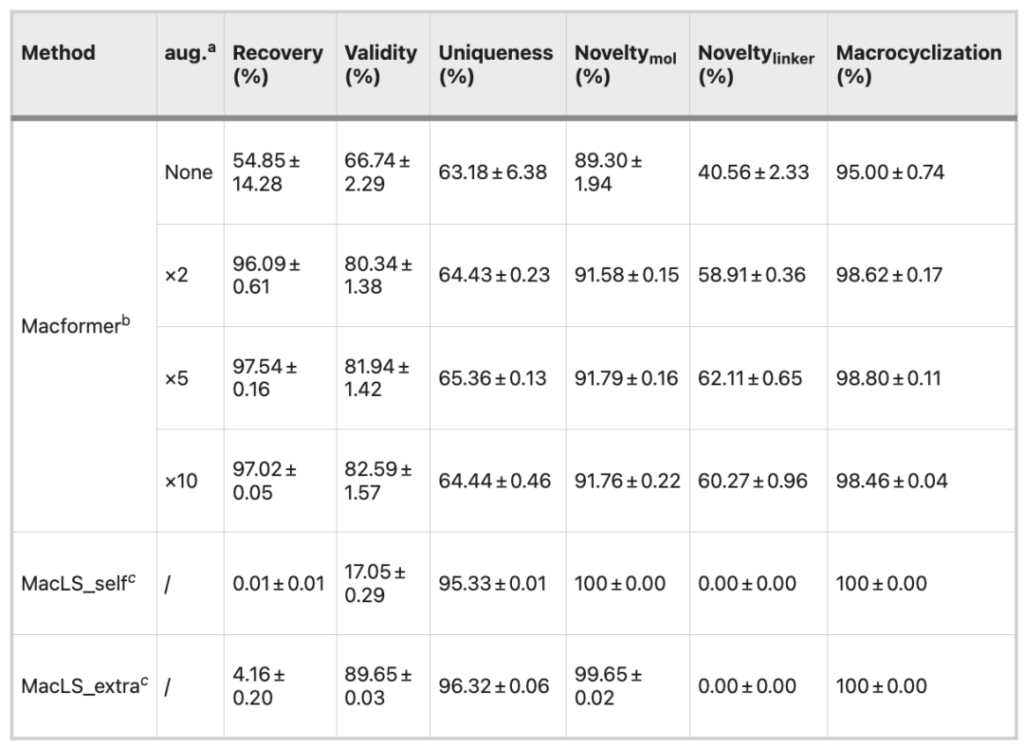
MacLS_self generates conformations de novo using acyclic SMILES, whereas MacLS_extra extracts conformations from low-energy 3D structures of target macrocycles.
The validity of MacLS_self is only 17.05%, while the novelty and uniqueness of MacLS_extra compounds surpass those of Macformer. However, MacLS can only search for linkers from the training set, so the novelty of linkers is 0. Moreover, the rate of macrocyclic compounds recovered by MacLS is also very low, less than 5%.
Comparative Study:ZINC Dataset
Furthermore, the two models were compared on the external dataset ZINC. The Macformer model trained on the dataset expanded 5 times has a recovery rate of more than 80%, an effectiveness of more than 84%, and a novelty of more than 99%.The above results show that Macformer has excellent generalization ability after data expansion.
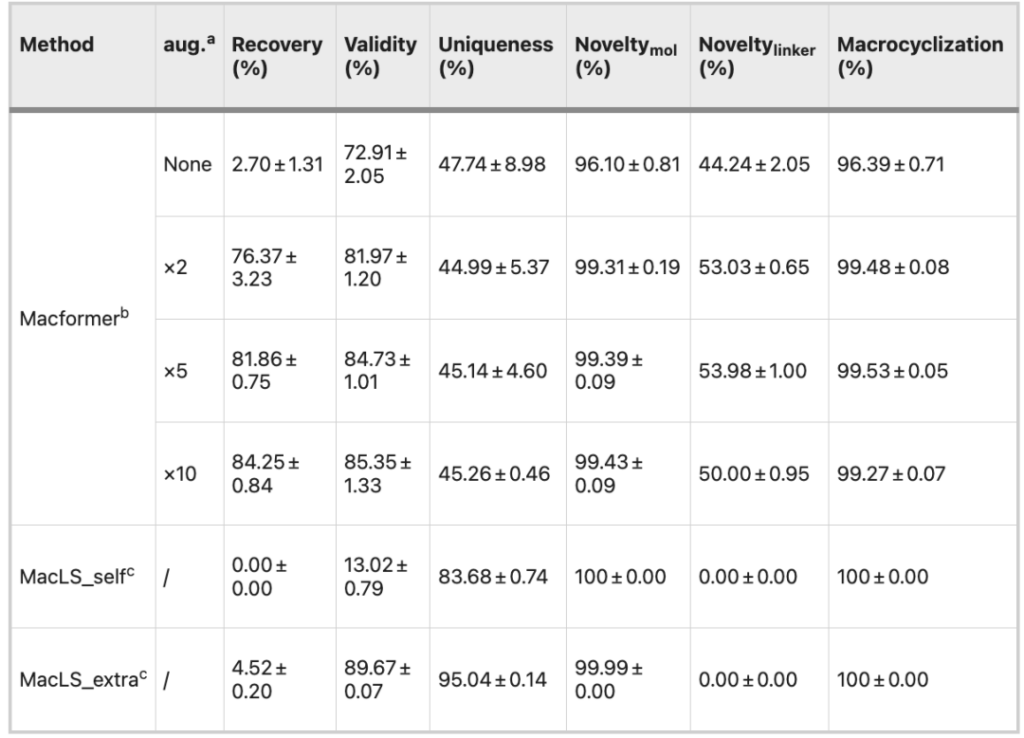
Since MacLS does not have the learning ability of Macformer, its results on different datasets are basically similar.
Chemical distribution:Macformer is more similar to input
Regardless of the novelty of the linker, both Macformer and MacLS have the ability to generate new macrocyclic compounds. Therefore, the researchers compared the distribution of the compounds generated by the two in chemical space.
First, the similarity between compounds was compared using the Tanimoto coefficient. Due to the structural similarity between acyclic compounds and macrocyclic compounds, the Tanimoto coefficients of most compounds generated by the model were above 0.7. However, the structural similarity between the compounds generated by Macformer and the original compounds was higher than that of MacLS_extra.
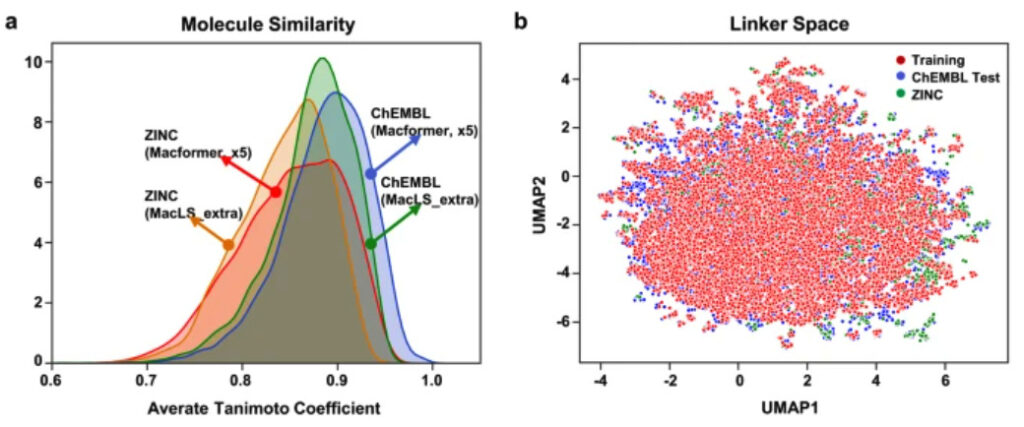
This result is rather unusual because Macformer can infer connectors that do not exist in the training set, while MacLS does not have this ability. To this end, the researchers used the unified manifold approximation and projection algorithm (UMAP) to reduce the dimensionality of the data. The results show thatThe new linkers generated by Macformer are distributed near the ChEMBL training set..
Experimental verification
Drug Development:Macrocyclization of fuzotinib
In recent years, macrocyclic compounds have attracted much attention as potential kinase inhibitors. To verify the predictive performance of the model, researchers used Macformer to design an inhibitor of JAK2. JAK2 belongs to the JAK family of kinases and is an important target for the treatment of myeloproliferative neoplasms and rheumatoid arthritis.
The input to the model is feizotinib, a small molecule drug used to treat myelofibrosis. Feizotinib is more selective for JAK2 than other JAK kinases, but has poor selectivity for other kinases, leading to other side effects.
The connection points of macrocyclization were set to the two terminal benzene rings, and the tert-butyl xanthamide that might be unfavorable for contact with the Asp994 target was removed. In order to increase the diversity of predicted macrocyclic drugs, each source SMILES sequence was expanded 10 times.Ultimately, Macformer output 10,700 results, including 281 new macrocyclic drugs.
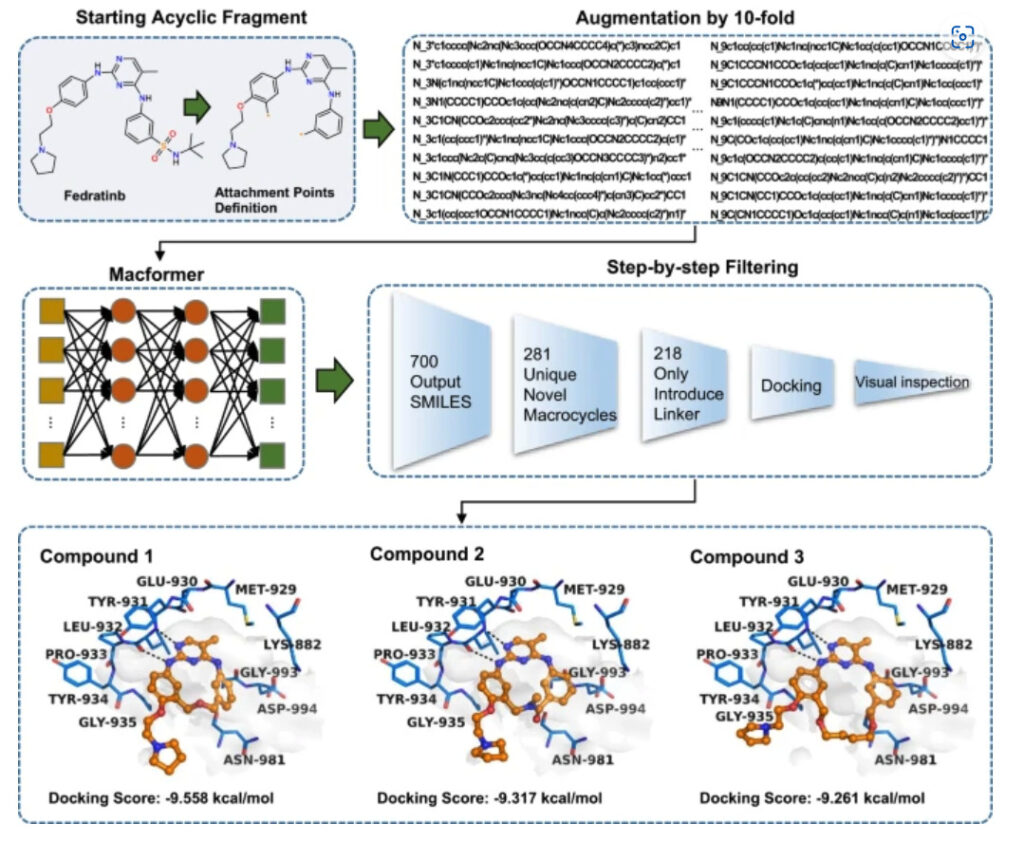
After evaluating the binding of macrocyclic drugs to targets and the feasibility of synthesis, the researchers finally selected three compounds for synthetic evaluation testing.Among them, the linker of compound 1 has not been reported in the design of macrocyclic drugs or the development of JAK2 inhibitors..
Among the 300 macrocyclic drugs designed by MacLS,These 3 compounds were not found, which once again proves the potential of deep learning algorithms in new drug design.
In vitro evaluation:Activity at the enzyme and cellular levels
Subsequently, the activities of these three compounds against JAK2 were evaluated.The half inhibitory concentrations (IC50) were 0.07, 0.364 and 0.006 μM, respectively. The most potent compounds 1 and 3 were evaluated for specificity at 100 μM, with only 10 and 17 wild-type kinases inhibited, respectively, whereas 34 wild-type kinases were affected by fuzotinib, indicating that compounds 1 and 3 were more selective.
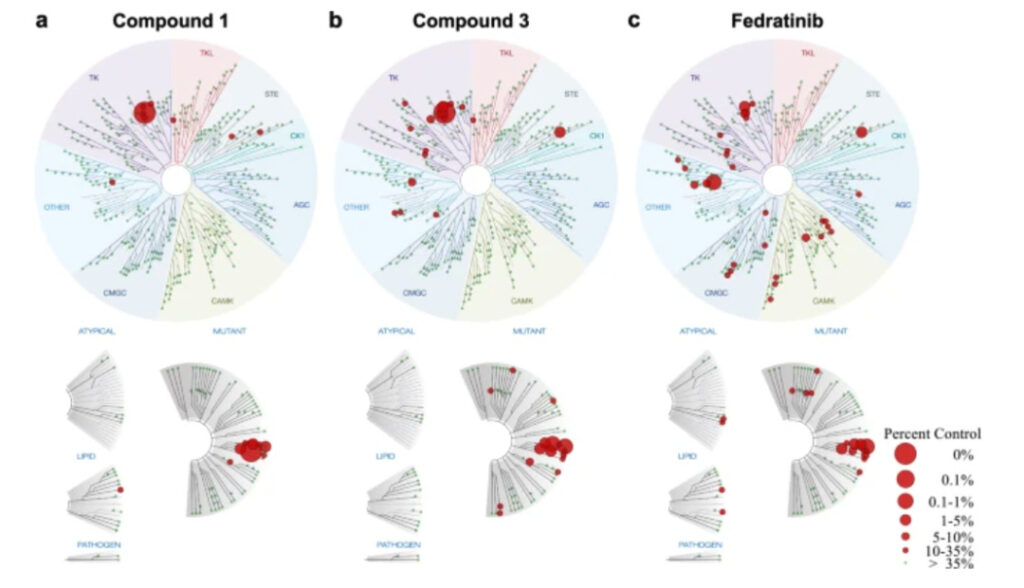
At the same time, the antiproliferative properties of compounds 1-3 on JAK2-dependent cells were also evaluated.Compounds 1 and 3 can inhibit the proliferation of JAK2-dependent cells at a lower dose than fuzotinib.
In vivo evaluation:Pharmacokinetic testing
Finally, the pharmacokinetic (PK) of compounds 1 , 3 and fuzetinib after intravenous (iv, 5 mg/kg) and oral (po, 5 mg/kg) administration was studied.
In addition to bioavailability (9.4% vs. 11.7%), compound 3 is superior to fuzotinib in all aspects. At the same time, compound 1 also has advantages in oral properties, such as systemic exposure (106.00 vs. 50.19 h*ng/mL) and bioavailability (14.1% vs. 11.7%). The above results show thatMacrocyclization is beneficial to improve the metabolic stability of fuzotinib-type drugs.
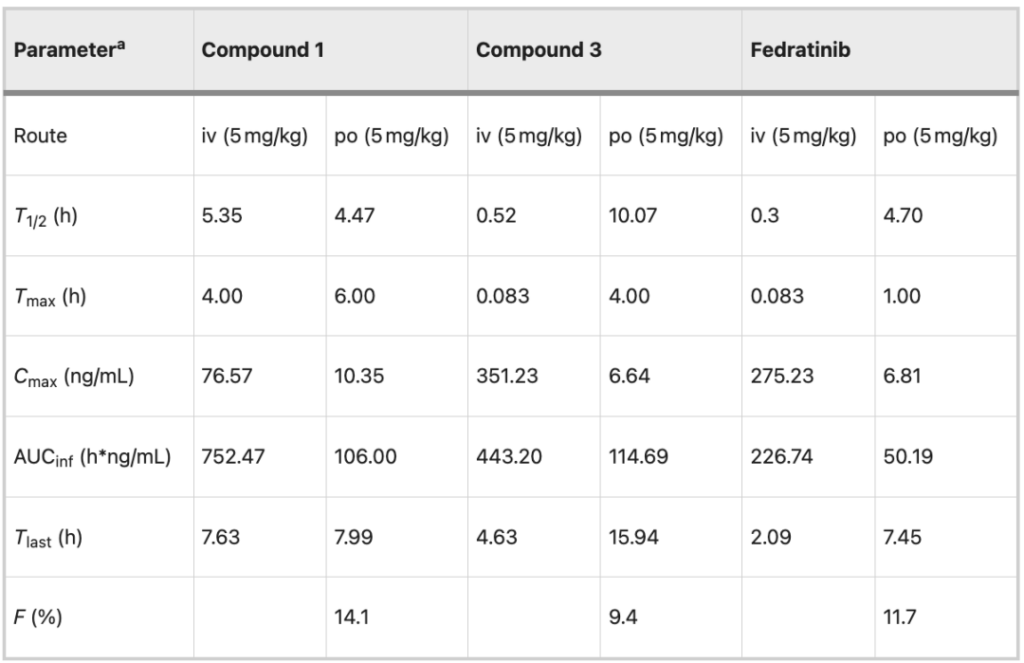
In vivo testing:Compound 3 inhibits inflammation
It has been reported that overexpression of JAK2 can lead to inflammatory bowel disease (IBD), which means that inhibiting the activity of JAK2 may be helpful in the treatment of IBD.The researchers tested macrocyclic drugs in mouse models to evaluate their role in the treatment of IBD.
According to the pharmacokinetic test results, the dosage of feizotinib was twice that of compound 3. The results showed that both compound 3 and feizotinib alleviated the weight loss caused by IDB, and the disease activity index of the experimental group was significantly reduced from the 8th day.
Finally, HE staining was used to analyze the severity of inflammation. The control group showed significant inflammatory response, including inflammatory cell infiltration and goblet cell loss, while the inflammatory response in the experimental group was mild and the colon structure was intact.

The above results show that the macrocyclic compounds deduced by Macformer are superior to traditional drugs in pharmacokinetics and selectivity, and can achieve disease treatment with lower doses.
High efficacy, difficult synthesis, the joy and sorrow of macrocyclic rings
As of 2020,The U.S. Food and Drug Administration (FDA) has approved 67 macrocyclic drugs, accounting for 41% of all approved drugs. Of these, 59 are natural macrocyclic drugs and only 8 are non-natural. In 2008, the FDA approved the first non-natural macrocyclic drug, Plerixafor, for cancer treatment.
The main indications of macrocyclic drugs are infectious diseases, accounting for 44.4%, followed by tumors (20.8%) and antifungals (8.3%). In recent years, the use of macrocyclic drugs in the anti-tumor direction has surged. There were only 4 types before 2007, and 11 types were approved afterwards.

Macrocyclic drugs can provide diverse functions and complex chemical structures in a semi-rigid, pre-organized structure, which can increase the affinity and selectivity of macrocyclic drugs for targets that are difficult to bind to with traditional small molecules, thereby improving drug efficacy. In addition, some macrocyclic drugs can adjust their conformation to adapt to the external environment. This ability improves their water solubility and cell permeability.
However,The synthesis of macrocyclic drugs is very complicatedWhile the macrocyclic structure enhances the ability to bind to specific targets, it also leads to ring strain, spatial interactions, and non-covalent cross-ring interactions.This makes it more difficult to predict molecular structure and properties..
AI is increasingly being used in drug development. However, limited data often limits the performance of AI.The researchers performed data augmentation using random SMILES expressions,While ensuring the richness of the data set, the prediction performance of Macformer is improved.
In the future, as people's understanding of drug structure and properties continues to deepen, AI will have a higher level of involvement in the development of new drugs, safeguarding people's health.
Reference Links:
[1]https://www.cambridgemedchemconsulting.com/resources/hit_identification/macrocycles/macrocycles.html
[2]https://pubs.acs.org/doi/10.1021/acs.jmedchem.3c00134