1.6 Million+ Unlabeled Images, 3-dimensional Comprehensive Evaluation, Zhou Yukun and Others Developed the RETFound Model to Predict Multiple Systemic Diseases Using Retinal Images
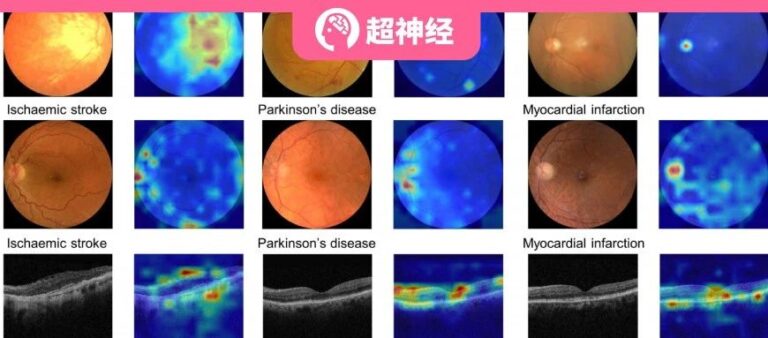
Whether it's 3D bioprinting in "Westworld", Luke Skywalker's mechanical arm in "Star Wars", or the virtual world created by AI in "The Matrix", the rich imaginations in these science fiction films all reveal mankind's yearning for health and longevity.
Nowadays, medical technologies that often appear in movies, such as robotic arms and artificial intelligence, have become a reality. Imagine that in the future, doctors only need to scan your eyes to know your heart health and predict your risk of Parkinson's disease. Doesn't it sound sci-fi? But this is not a movie, but a real thing.
Author: Qiao Qiao
Editor: Sanyang
The retina is the only part of the human body where the capillary network can be directly observed. It is also part of the central nervous system. Traditional medical artificial intelligence often diagnoses eye diseases by identifying health conditions in retinal images.
However,The development of AI models requires a large amount of data annotated by professionals, and the models are usually targeted at specific disease tasks.It cannot be extended to various clinical applications.
To address this situation, Zhou Yukun, a doctoral student from University College London (UCL) and Moorfields Eye Hospital, and others proposed a retinal image basic model RETFound.It is trained using self-supervised learning on more than 1.6 million unlabeled retinal images.It has excellent performance in tasks such as eye disease diagnosis/prognosis and prediction of systemic diseases.
The related paper has been published in Nature.
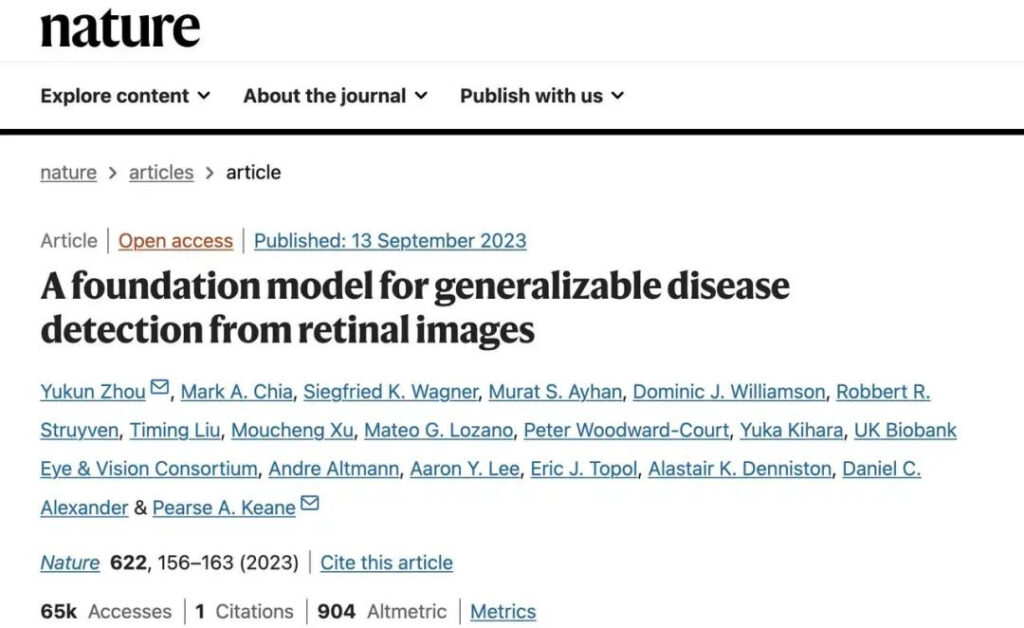
Get the paper:
https://www.nature.com/articles/s41586-023-06555-x
Reply "retina" in the WeChat public account to get the full paper PDF
RETFound model training details
Training data: CFP+OCT, a total of 1.64 million+ images
Building the RETFound dataset consists of two parts:
* CFP Image:A total of 904,170 images, of which 90.21 TP3T came from MEH-MIDAS and 9.81 TP3T came from Kaggle EyePACS33
* OCT images:A total of 736,442, of which 85.2% came from MEH-MIDAS and 14.8% came from other references
MEH-MIDAS is a retrospective dataset.Complete ocular imaging records of 37,401 patients (16,429 women, 20,966 men, and six of unknown sex) with diabetes attending Moorfields Eye Hospital, London, between 2000 and 2022 were included.
The mean age of these patients was 64.5 years with a standard deviation of 13.3 years. Taking into account the diversity of ethnic distribution, patients included British (13.7%), Indian (14.9%), Caribbean (5.2%), African (3.9%), other races (37.9%) and patients who did not disclose their race (24.4%).
The data of the MEH-MIDAS dataset come from a variety of imaging devices, such as topcon 3DOCT-2000SA (Topcon), CLARUS (ZEISS) and Triton (Topcon).
The data imaging devices for the EyePACS dataset include Centervue DRS (Centervue), Optovue iCam (Optovue), Canon CR1/DGi/CR2 (Canon), and Topcon NW (Topcon).
RETFound:Base model for retinal images
RETFound is a foundational model for retinal images.It is trained on 1.6 million unlabeled retinal images using a self-supervised learning method and can be applied to other eye and systemic disease detection tasks with clear annotations.
The implementation of the RETFound model uses a masked autoencoder with a specific configuration.This masked autoencoder consists of two parts:
* An encoder:Using the large vision Transformer (ViT-large), which contains 24 Transformer blocks and an embedding vector of size 1,024, the input is unmasked patches (16×16) and is projected into a feature vector of size 1,024. These 24 Transformer blocks include a multiheaded self-attention mechanism and a multilayer perceptron, which accepts feature vectors as input and generates high-level features.
* A decoder:Use small vision Transformer (Vit-small) with 8 Transformer blocks and embedding vector of size 512. Insert masked dummy patches into the extracted high-level features as model input, and then reconstruct the image patches after linear projection.
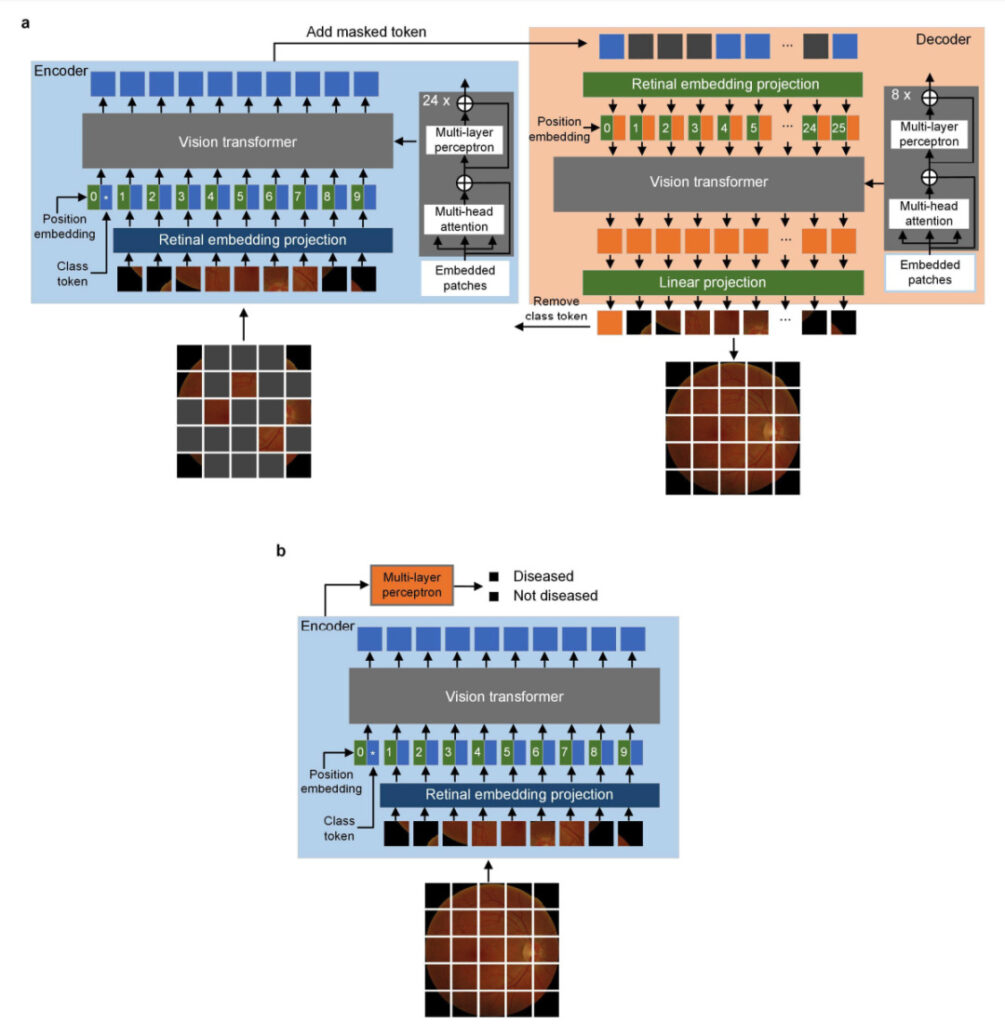
RETFound model architecture diagram
The goal of model training is to reconstruct retinal images from highly masked versions.The mask ratio of CFP is 0.75, the mask ratio of OCT is 0.85, the batch size is 1,792 (8 GPUs × 224 per GPU), the total number of training epochs is 800, and the first 15 epochs are used for learning rate warm-up (increasing from 0 to 1×10-3 The model weights of the final epoch are saved as a checkpoint for adaptation to downstream tasks.
3 dimensions to evaluate RETFound model performance
In order to evaluate the performance and labeling efficiency of the RETFound model, researchers compared the RETFound model with three other pre-trained models.They are SL-ImageNet, SSL-ImageNet and SSL-Retinal.All models use different pre-training strategies but have the same model architecture and tuning process for downstream tasks.
1. Diagnosis of eye diseases
The researchers used eight public datasets to validate the performance of the RETFound model across a variety of eye diseases and imaging conditions.
Internal Assessment
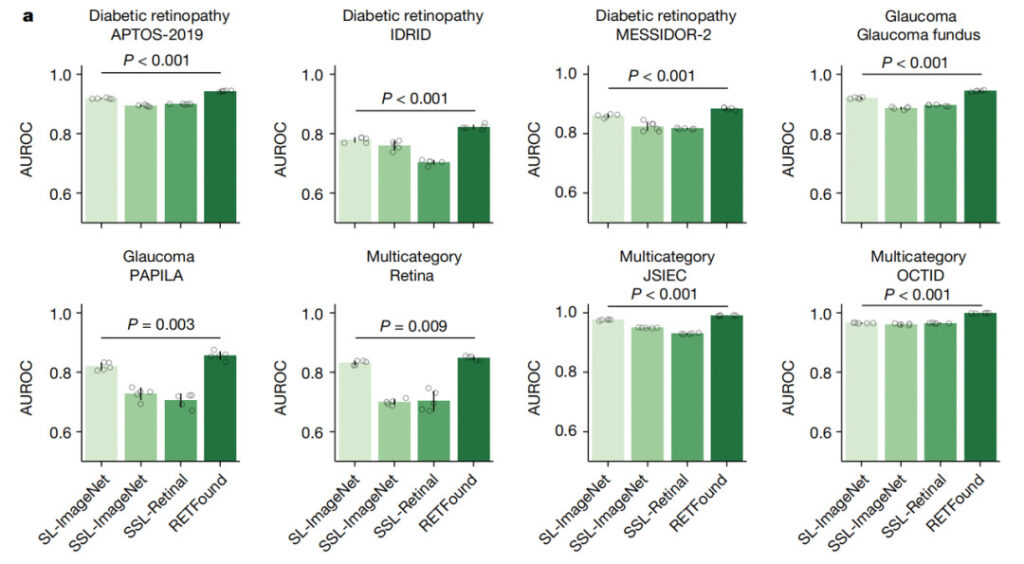
The figure above shows the internal evaluation, where the tuned model is applied to each dataset and internally evaluated on the reserved test data in the ophthalmic disease diagnosis task (such as diabetic retinopathy and glaucoma).
The experimental results show that:RETFound achieved the best performance in most datasets, with SL-ImageNet ranking second.
External evaluation
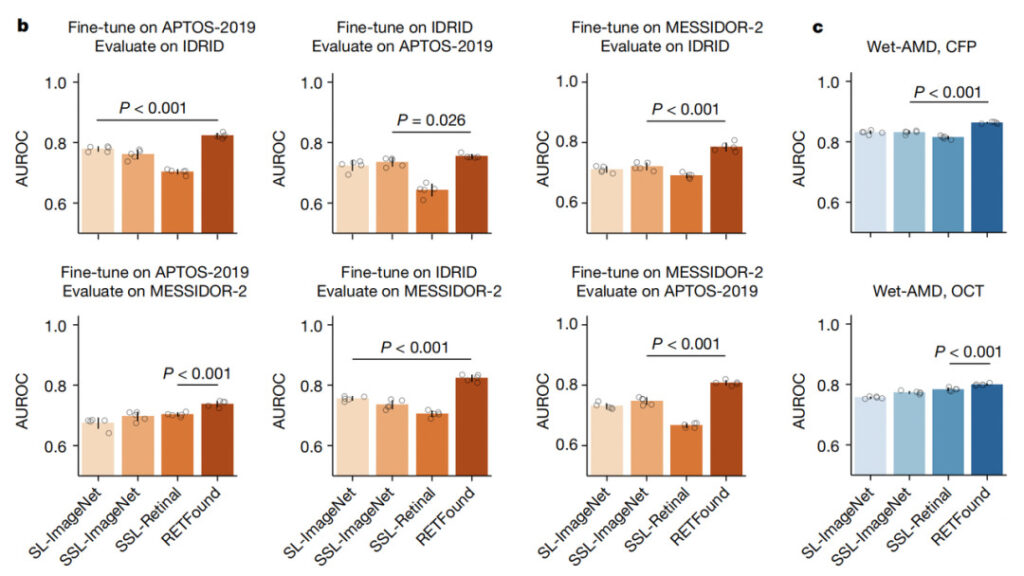
For external evaluation, researchers evaluated the performance of the RETFound model on diabetic retinopathy datasets (Kaggle APTOS-2019, IDRID and MESSIDOR-2), which are annotated on the 5-level International Clinical Diabetic Retinopathy Severity Scale. Cross-evaluation was performed between the three datasets, that is, the model was tuned on one dataset and evaluated on the other datasets.
Experimental results show that the RETFound model achieves the best performance in all cross-evaluations.
2. Prognosis of eye diseases
The researchers also tested the prognosis of the other eye converting to wet age-related macular degeneration (wet-AMD) within 1 year on the AlzEye data.turn out:
* When the input is CFP, RETFound has the best performance, with AUROC reaching 0.862 (95% CI 0.86, 0.865), which is significantly better than the comparison group;
* When the input was OCT, RETFound scored the highest with an AUROC of 0.799 (95% CI 0.796, 0.802), showing a statistically significantly higher AUROC than SSL-Retinal.
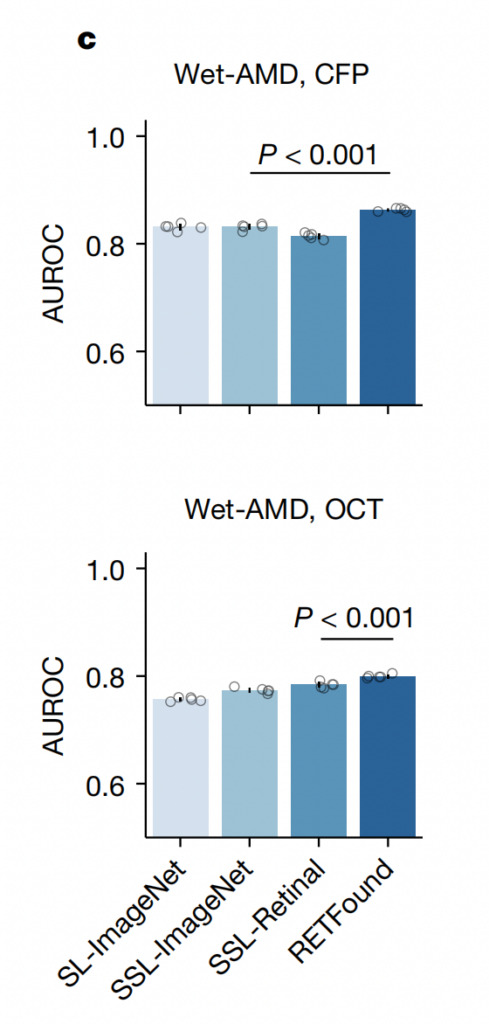
Experimental results show that the RETFound model performs best in all tasks.
3. Prediction of systemic diseases
The researchers used four systemic diseases to evaluate the performance of the RETFound model in predicting the correlation between retinal images and systemic diseases.
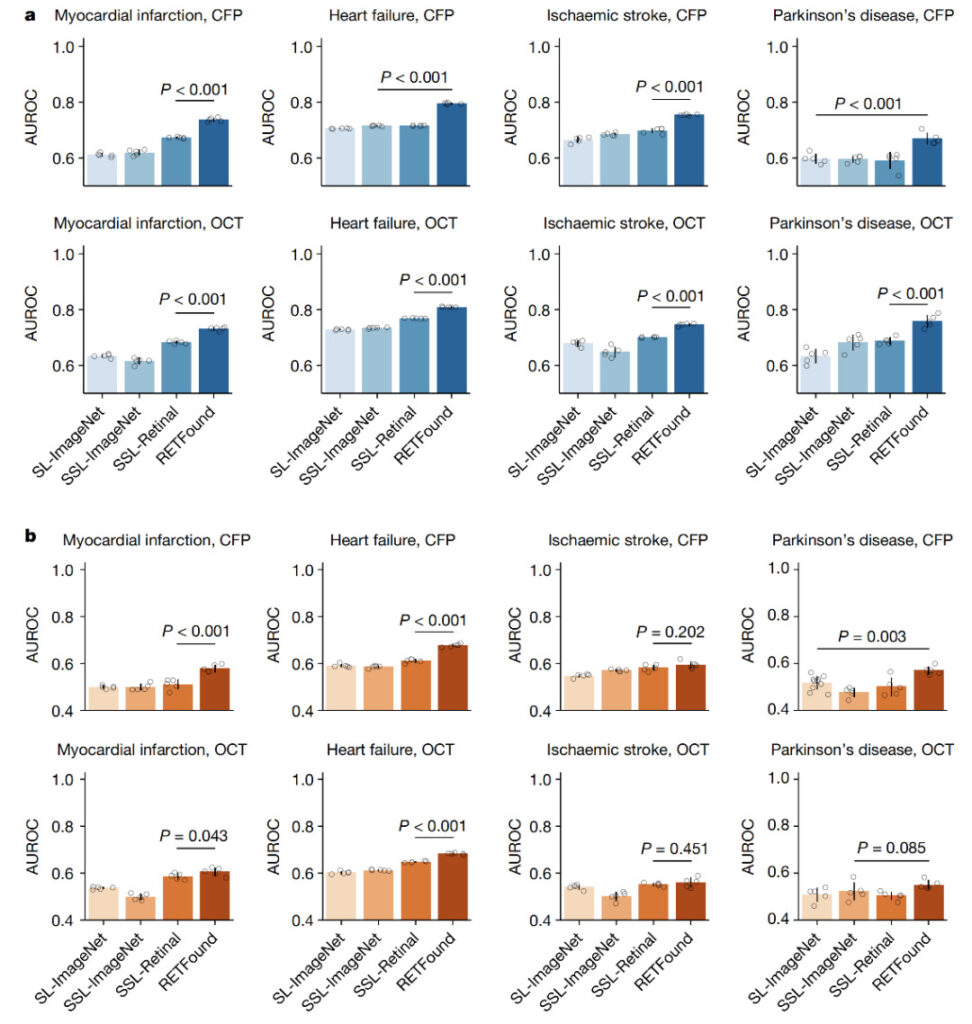
Performance of a model for predicting the 3-year incidence of systemic diseases using retinal images
The four systemic diseases are: myocardial infarction, heart failure, ischemic stroke and Parkinson's disease.
Experimental results show that the RETFound model outperforms other comparison models and ranks first in the prediction of four diseases.
Limitations and Challenges of the RETFound Model
Although the research process systematically evaluated the role of RETFound in diagnosing and predicting systemic diseases such as heart disease, heart failure, stroke, and Parkinson's disease, there are still some limitations and challenges that need to be further explored in future work.
Firstly, most of the data used to develop RETFound comes from the UK, so it is necessary to consider the impact that the introduction of global retinal images in the future may have on the model results.It is necessary to introduce more diverse and balanced data into the model.
Second, although this study explored the performance of the model under CFP and OCT,However, the multimodal information fusion between CFP and OCT has not been studied yet.This may lead to further performance improvements for RETFound.
Finally, some clinically relevant information,For example, demographics and visual acuity,Although they may serve as valid covariates for ophthalmological studies, they have not been included in the SSL models.
Currently, the developers of RETFound have made this model public, hoping that talents from all over the world can adjust and train RETFound.Making it applicable to different patient groups and healthcare settings.
AI helps the new future of smart healthcare take shape
So far, RETFound is one of the few successful applications of the basic model in medical imaging.While improving model performance and reducing the labeling burden on medical experts, it has also aroused people's attention to the practical application of medical AI.
Today, the medical industry is entering a period of explosive growth in digitalization, and capital from various industries has entered the market to promote the application of AI technology in the medical industry.
According to statistics from the China Business Industry Research Institute, AI+ medical care accounted for 18.9% of the artificial intelligence market in 2020, with a market size of 6.625 billion yuan. According to IDC statistics, the total value of the artificial intelligence application market will reach 127 billion US dollars by 2025, of which the medical industry will account for one-fifth of the market size. From the basic layer to the application layer, there is a lot to do in the vast market of medical AI.
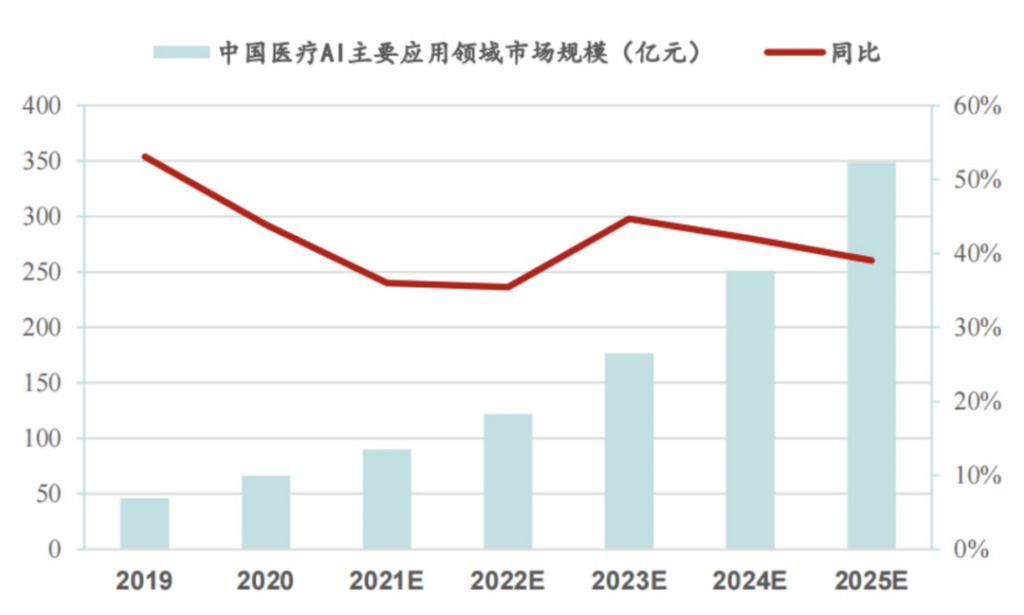
Source: China Business Industry Research Institute
Looking at overseas markets, medical AI applications are gradually being implemented:In March of this year, Nuance, a clinical documentation software company owned by Microsoft, added GPT4 to its latest voice transcription application; in April, Microsoft and Epic announced that they would introduce OpenAI's GPT-4 into the healthcare field to help medical staff respond to patient information and analyze medical records; in the same month, Google announced that it would release its medical large model Med-PaLM 2 to the user group.
Domestically, iFlytek, SenseTime and others are actively making plans and accelerating the exploration of industry applications. AI+healthcare has become a trend that the global technology community has reached consensus on.
Industry insiders believe that the application of large AI models is expected to significantly alleviate the pain points of the medical industry. With the further deepening of application scenarios, the era of intelligent medical industry is expected to officially begin, and the industry has huge long-term opportunities.
Reference Links:
[1]https://www.nature.com/articles/s41586-023-06555-x
[2]https://www.nature.com/articles/d41586-023-02881-2