Argonne National Laboratory Releases FAST, a Fast Automated Scanning Kit That Makes “fast Reading” of Microscopy Possible
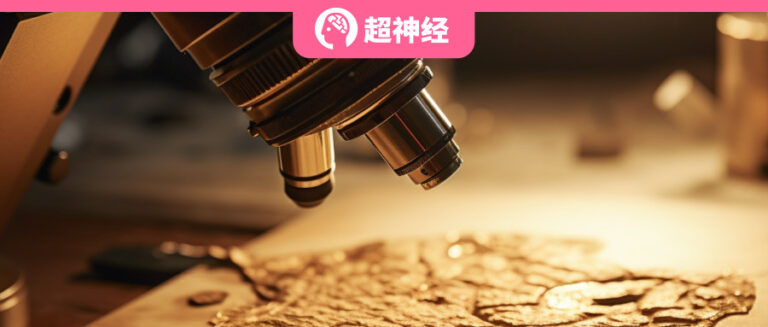
"I am happy to see the red national flag raised in Tiananmen Square in Beijing"
If you read this sentence quickly, you may find that only a few words like "I watched the flag-raising ceremony at Tiananmen Square" can summarize the information we need. In other words, we don't need to read it word by word, but can decipher all the information by grasping the key points. So, can scientific research be done in this way?
Inspired by this, researchers combined artificial intelligence (AI) with microscopy technology, training AI to actively identify key features in samples for researchers to analyze. Unlike traditional microscopy, which involves full-point scanning of samples, the AI + microscopy method has completely changed the way researchers obtain sample data, significantly speeding up the experimental process and achieving "fast reading" at the microscopic level.
Author | Add Zero
Editor | Xuecai, Sanyang
The principle of the microscope is to generate spatially resolved signals by scanning the sample, collect the signals for analysis, and thus form an image of the sample. With the continuous advancement of instrumentation technology,The scanning speed and resolution of microscopes have been greatly improved.
However, high-resolution scanning microscopy experiments have some significant disadvantages:The amount of data generated is huge, and the probe causes great damage to the sample.For example, X-ray imaging of a 1 mm^3 volume at a resolution of ≈10 nm is performed.Traditional scanning methods produce 10^15 voxels of data and require a fairly large probe dose.
At the same time, most areas in the sample have low information density and can be directly ignored.On the contrary, a small number of "boundaries, defects, special elements" areas contain rich information and need to be studied in depth..
To accurately locate key areas with high information density, traditional methods mainly rely on experienced operators to analyze data and guide probe scanning, which greatly increases the workload and slows down the progress of the experiment.
So, is it possible to introduce AI to help researchers identify research priorities in samples and speed up data collection and analysis?

Artist's impression of automated dark-field scanning microscopy experiments at the APS.
To this end, researchers at the Argonne National Laboratory in the United States developed FAST (Fast Autonomous Scanning Toolkit), which is a fast automatic scanning kit that is different from the full-point scanning of traditional microscope research samples.FAST allows AI to automatically identify the scanned location, thereby acquiring sample information efficiently and accurately.The relevant results have been published in "Nature Communications".
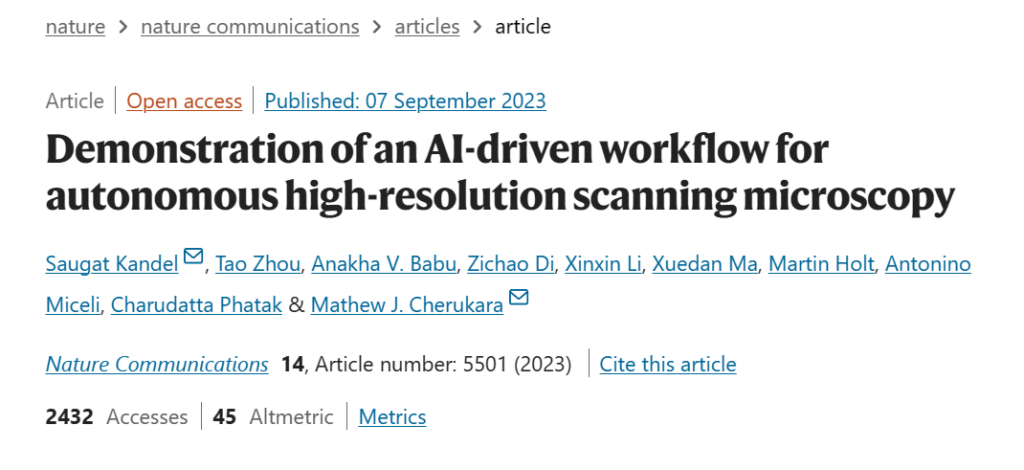
Related results have been published in "Advanced Science"
In simulations and dark-field X-ray microscopy experiments, FAST researchers performed FAST scans on WSe2 films. The results showed thatA FAST scan of <25% is sufficient to accurately image and analyze the sample.
Paper link:
https://www.nature.com/articles/s41467-023-40339-1
Experimental procedures
Training Data:Using a general image training algorithm
The algorithm used by FAST does not need to be trained using large data sets, and the AI can use generic images to identify areas of interest.
The training data was generated from publicly available images from the MIT Libraries, USC-SIPI Image Database, and Scikit-image software package.

Examples of test images used
FAST:Rapid Autonomous Scanning Tool Kit
FAST stands for Fast Autonomous Scanning Toolkit.It combines the SLADS-Net method, path optimization technology, and efficient and modular hardware control.is a suite of fast autonomous scanning tools for real-time sampling and scan path selection in synchrotron-based scanning microscopy.

FAST Workflow
A:A set of quasi-random initial measurements are transmitted to the edge device, which in turn generates an initial sample estimate, calculates the next candidate points to be measured, and calculates the measurement path. The new measurements are combined with the existing measurements to calculate the new estimate, and the process is repeated until the completion criteria is met.
B:At the beginning of the candidate point operation, each unmeasured point is checked. PThe local neighborhood (radius r) of the measured points is highlighted, thus generating a 6-dimensional feature vector. The feature vector is converted into a 50-dimensional vector using the radial basis kernel function (RBF) kernel and used as the input of a multi-layer neural network (NN). Next, the neural network (NN) measures point P The expected improvement of the image is predicted (ERD). The group of unmeasured pixels with the highest ERD are selected as candidates for the next measurement.
FAST training does not rely on specific sample data.A complex (non-binary) sample can be dynamically measured and reconstructed, which is different from existing SLADS-based workflows. Moreover, its computational cost is negligible compared to the acquisition time, even when running on a low-power edge computing device (placed on a synchrotron beamline), which is a significant advantage for more general autonomous experimental techniques.
These features enable FAST to be applied to the existing high-precision nanoscale scanning X-ray microscope instrument at the APS hard X-ray nanoprobe beamline.
Performance Verification
FAST:Better than static sampling methods
To verify the performance of FAST, the researchers conducted comparative experiments with three other static sampling techniques.
Subjects:That is, the test data set is a 600 × 400 pixel dark-field image, representing 240,000 possible measurement positions, covering a physical area of 900 × 600 μm, and containing multiple WSe2 slices of different thicknesses;
Comparison method:The three static sampling techniques are raster grid sampling (RG), uniform random sampling (UR), and low-discrepancy quasi-random sampling (LDR).
Experimental process:At the same scanning coverage, FAST, RG, UR and LDR sampling reconstruction images are generated.
Comparison 1:

Comparison of FAST and static sampling reconstruction images
A:Ground truth image, the color scale represents the normalized intensity
BD:RG, LDR and FAST reconstruction images under 10% scanning coverage
The results show:FAST sampling is able to reproduce boundaries, bubbles, and transition areas between different thickness levels in the experimental objects with high fidelity.
Comparison 2:

Performance comparison of FAST and static sampling methods at different scanning coverage
A:Normalized squared mean error (NRMSE) varies with scan coverage, and lower values indicate better performance;
B:The structural similarity measure (SSIM) varies with the scan coverage, and the higher the value, the better the performance.
The results show:FAST has high sampling efficiency and can achieve stable reconstruction when the scanning coverage reaches 27%; the three static sampling methods require longer time to achieve the same effect.
Contrast three:
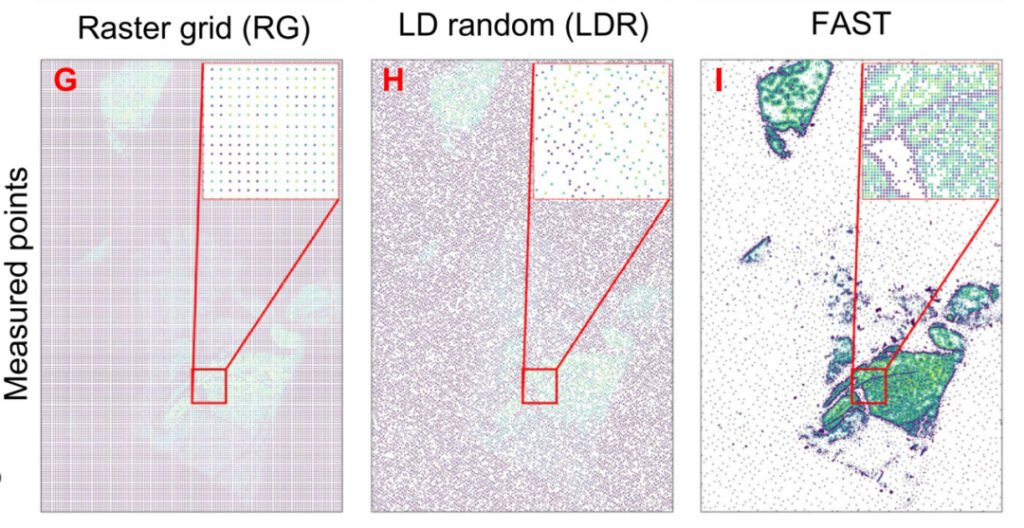
Actual measurement positions of FAST and two static sampling methods at a coverage rate of 10% under the same sampling conditions
The results show thatThe FAST reconstructed structures have high similarity and low error.
In the future, the results can be further improved by using more sophisticated inpainting techniques in the FAST method.
The comparison results of the above three groups show:
FAST is superior to static sampling techniques. FAST will preferentially select areas with significant heterogeneity for sampling rather than uniform areas. This greatly reduces the time of ineffective sampling in blank areas and is particularly effective for sparse samples.
FAST: Efficient and accurate dark field image reconstruction capability
In synchrotron beamline experiments, FAST further demonstrated its excellent performance.
The experimental process was completely self-driven by AI, and the researchers did not interfere with anything except starting the FAST script. The experimental sample was a deformed WSe2 flake with a spatial resolution of 100 nm.

The evolution of FAST scanning
A, C, E:FAST reconstructed dark-field images at 5%, 15%, and 20% scan coverage;
B, D, F:The corresponding actual measurement points;
G:Image obtained by full grid point-by-point scanning (100% coverage);
AG:The color scale shows the normalized intensity;
H:Only sampling points between 15%-20% coverage are shown.
The figure above shows that in the case of low scan coverage,The FAST method preferentially identified some heterogeneous regions.Such as the edge of bubbles; as the scanning coverage gradually increases, the reconstruction results become clearer and clearer, and the reconstructed image reaches stability between the scanning coverage of 15%-20%.
20% scanning coverage,FAST can clearly and accurately reproduce all major features in the full scan image.At the same time, it helps save about 80 minutes (≈65%) of experiment time, greatly improving the experimental efficiency.
The future of AI + microscopy
The advantage of the FAST process lies not only in improving the efficiency of microscopic data acquisition, but also in its wide range of applications.Tao Zhou, a scientist from Argonne National Laboratory in the United States, said, "From X-ray microscopes to electron microscopes to atom probe microscopes, this technology can empower any microscopy research that requires two-dimensional scanning."
In the future, AI technology will also see deeper applications in the field of microscopy. Through training, AI is gradually taking over tasks such as automated image analysis and recognition, image enhancement and reconstruction, quantitative analysis, and disease diagnosis.
AI + microscopy technology is moving towards a clearer, more efficient and more precise future, and the boundaries of scientific research will continue to expand.
Reference Links:
[1]https://www.nature.com/articles/s41467-023-40339-1
[2]https://phys.org/news/2023-10-artificial-intelligence-scientists-self-driving-microscopy.html
[3]A Supervised Learning Approach for Dynamic Sampling