Bioinformatics | Start Research More Efficiently With AI
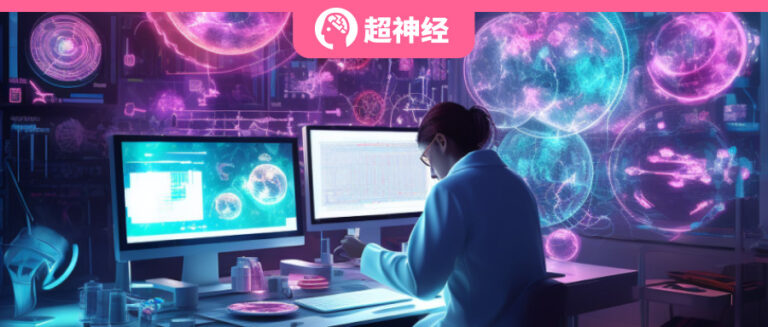
Bioinformatics refers to the study of biological problems using methods from applied mathematics, informatics, statistics and computer science.
With the development of computer science and technology, AI has shown great advantages in solving complex and challenging biological research problems, further accelerating the transformation and upgrading of traditional research paradigms.
Author | Sanyang
Editor | Xuecai
When it comes to bioinformatics, the most well-known one is AlphaFold.
AlphaFold is a deep learning system first released by DeepMind in 2018, mainly used to predict protein structure.It has been described by many industry insiders as "completely changing biology."
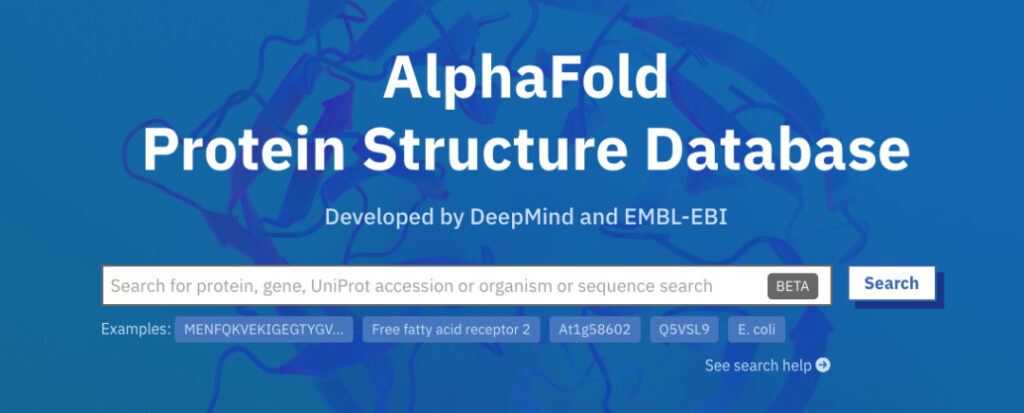
In fact, apart from well-known bioinformatics advances such as AlphaFold,AI has abundant application cases in biological fields such as homology search, multiple alignment and phylogeny construction, genome sequence analysis, and gene discovery.
Taking nanotoxicology as an example,The image-based analysis of human cells is a lengthy and error-prone process, requiring a great deal of time to manually analyze images and compare scans of each cell one by one.
With the free open source software CellProfiler,Biologists with no programming experience can use image analysis algorithms out of the box to explore the effects of silver nanoparticles (AgNPs) on liver cells.
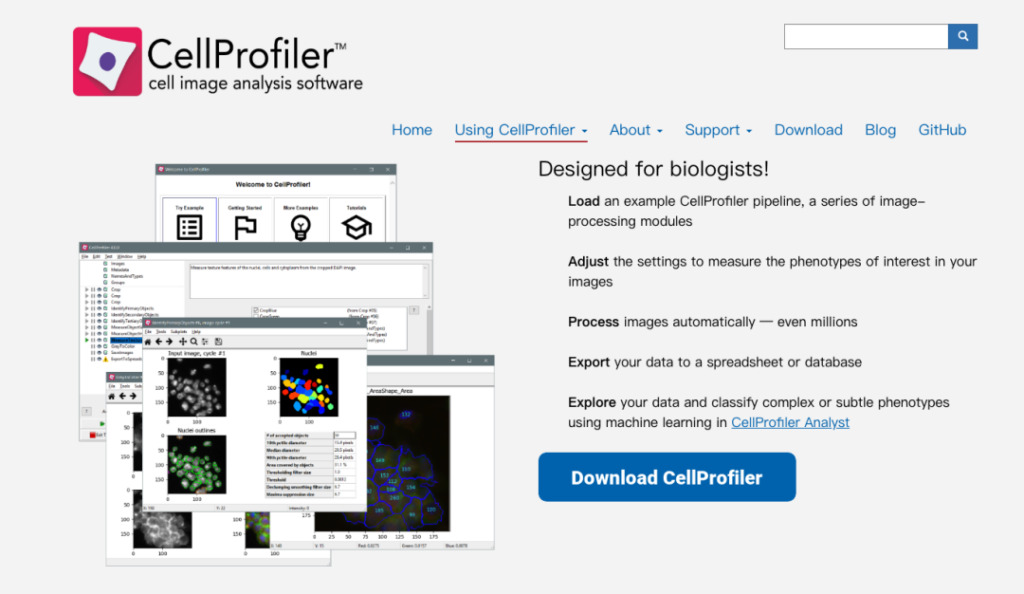
Bioinformatics skills have become essential for contemporary life science research. As a biological researcher,Ability to skillfully integrate machine learning tools into data analysis,It will definitely accelerate scientific discovery and improve scientific research efficiency.
This article will briefly describe how biologists use AI to get rid of a large number of repetitive experiments and accelerate the traditional scientific research process from the perspectives of tool resources, methods and approaches, and peer communication.
Read the original article:
Follow the official account and reply "Bioinformatics" to get the full PDF of the paper

Familiar with common AI tools
AI plays an important role in improving scientific research efficiency.Image analysis algorithms can help researchers compare cell characteristics more quickly and quantitatively, freeing them from massive repetitive work, and adaptive learning can further accelerate this process.
In addition, AI can often detect differences or comparison patterns that users would not expect.By converting what the AI “sees” into numerical data, complex biological images can be transformed into a relatively straightforward mathematical problem and ultimately a data science problem.
Taking CellProfiler as an example, this online open source tool has a simple interface and is ready to use. It can run a large number of machine learning and deep learning algorithms, allowing users to customize pipelines and perform automated analysis of quantitative shapes, characteristics, and patterns.
In addition, there are open source AI tools such as ilastik, QuPath, and CDeep3M.No strong programming background is required, and with practice you can use these tools to solve cell and image analysis problems.

Improve professional skills and narrow the gap between peers
Contemporary bioinformaticians need to improve their professional skills from the following aspects:
- Have certain programming skills, master general programming languages such as Python, and be proficient in using Python for text processing, scientific computing, web services and other tasks;
- Consciously cultivate your basic mathematical and statistical abilities, which will be of great help to your career development;
- Be good at using tools, whether it is the scikit-learn machine learning library or ChatGPT, these tools can lower the learning threshold of AI-related knowledge;
- Self-study high-quality online courses, you can refer to the high-quality courses on online platforms such as Coursera, edX, and Udacity;
- Participate in online and offline seminars to deepen communication with peers and share cutting-edge learning methods

Pursue long-term value and focus on actual results
For traditional scientific research, it is not necessary to pursue the latest technology.
AI technology is changing with each passing day, but science does not change every week. If researchers are busy integrating the latest tools and keeping up with the progress of the literature every day, they will inevitably fall into a state of exhaustion.It is better to stop and think about which methods and advances are most useful for your own scientific research.
Although computers have advantages such as high efficiency and the ability to customize rules when processing biological image analysis tasks, it should be noted that the introduction of AI in the scientific research processPay special attention to risks and challenges such as uncertainty and human bias.We strive to make the results neutral, credible and explainable.
At the same time, data management is also a big challenge for AIForScience.Some projects will generate hundreds of megabytes of image and measurement data. Most contemporary scientific research projects are interdisciplinary and require more professionals with high-dimensional data processing capabilities and sufficient data science knowledge to join the project team.

Enhance global perspective and learn from the community
There are some very active online communities and high-quality project addresses in the field of biological sciences.These groups bring together users from all over the world at the intersection of AI and biology, and some members are very willing to share.
These resources include:
* forum.image.sc:Scientific Imaging Software Discussion Group, a collaboration between the Broad Institute and the University of Wisconsin-Madison
* BioStars.org:Online discussion group focusing on bioinformatics, computational genomics, and biological data analysis
* GitHub.com:Bioinformatics related project examples and code
also,The best way to improve your AI skills is to practice.In addition to participating in discussions and learning from others’ experiences, bioinformaticians can also try out a series of competition projects on Kaggle to play with AI programs and tools in the process of practice.
The trend of interdisciplinary studies is unstoppable. The rational and appropriate use of AI will surely become an important driving force for the development of biological sciences.I hope every biologist can start from now and use AI to accelerate scientific research progress and innovate thinking.