OBIA: 900+ Patients, 193w+ Images, the Chinese Academy of Sciences Institute of Genomics Released My Country's First Biological Image Sharing Database
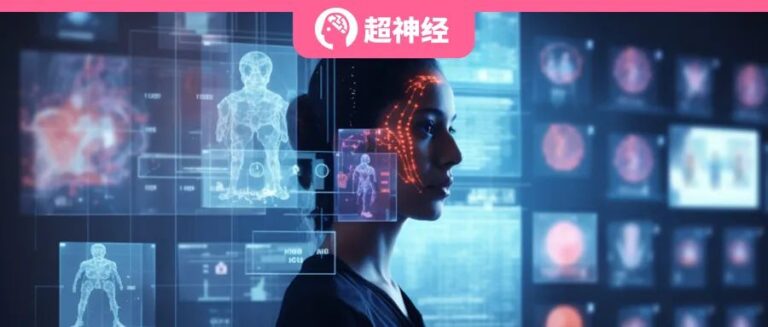
Taking X-rays is a common practice when seeing a doctor. CT, MRI, X-ray and other imaging data can be used non-invasively to penetrate the human body, making the internal organs and tissue conditions clearly visible, providing a reliable basis for clinical diagnosis and disease treatment.
With the widespread development of medical imaging technology, imaging data has accounted for more than 80% of domestic medical data.Pain points such as the shortage of radiologists, differences in diagnostic results among hospitals at all levels, and uneven distribution of medical resources are becoming increasingly prominent.
AI combined with medical imaging has great potential for imagination. Sensory cognition and deep learning technologies have incomparable advantages over humans in identifying medical imaging diagnostic results, and can assist doctors in reducing misdiagnosis rates and improving work efficiency.
However,High-quality AI algorithms require sufficiently large and representative image datasets.These medical images often involve a large amount of sensitive privacy information. In addition, there are "data islands" between hospitals at all levels, and the incomplete sharing system makes the available resources for medical imaging AI limited.
Author | Tower
Editor | Sanyang, Xuecai
Many countries around the world have built various medical imaging data sharing databases. my country still lags behind the international community in this field. In order to promote the sharing of high-quality medical biological imaging data,The Institute of Genomics, Chinese Academy of Sciences (National Center for Bioinformation, China) has established the Open Biomedical Imaging Archive (OBIA).
As the first open repository of biomedical imaging data and related clinical data in China,OBIA is open to medical practitioners and related scholars around the world free of charge. The preprint of the relevant results was published on "bioRxiv" on September 25, 2023.
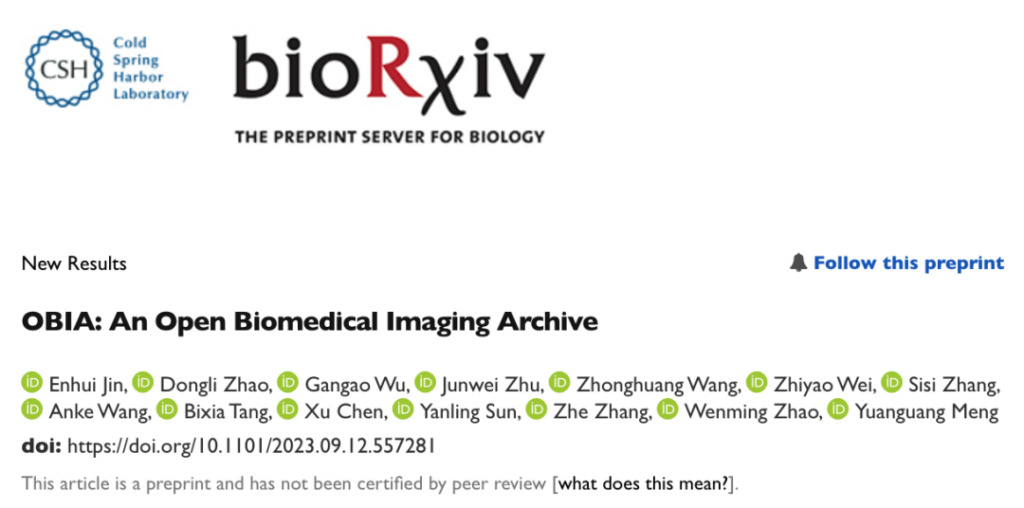
Paper link:https://www.nature.com/articles/s42256-023-00704-7
Follow the "HyperAI Super Neural" public account and reply "OBIA" to get the full PDF of the paper
OBIA database construction and implementation process
As the core database resource of the China National Center for Bioinformation, OBIA accepts image submissions from all over the world and provides free open access to all public data.It supports the de-identification, management and quality control of image data.Providing data services such as browsing, retrieval and downloading can promote the reuse of existing image data and clinical data.
OBIA uses five types of data objects (Collection, Individual, Study, Series, Image) to organize data.Accepts submissions of multi-modality, multi-organ, and multi-disease biomedical images.
To protect your privacy,OBIA has developed a unified de-identification and quality control process.It also provides an intuitive and friendly web interface for data submission, browsing and retrieval, as well as image retrieval. Overall, OBIA provides a reliable platform for domestic biomedical imaging data management and helps support global biomedical research.
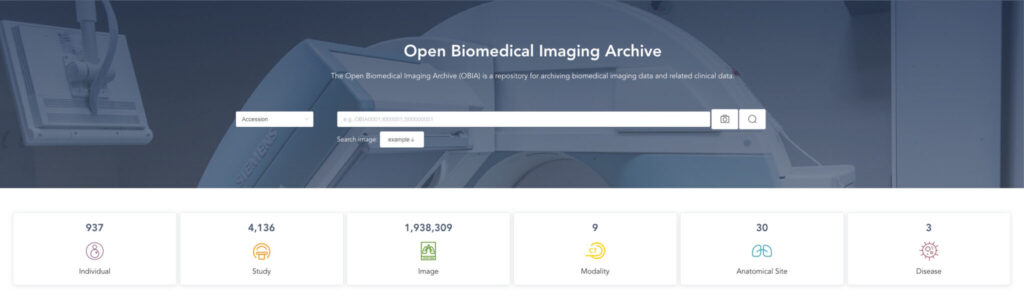
Figure 1: OBIA access interface
Visit URL:https://ngdc.cncb.ac.cn/obia
Implementation details——Image Retrieval
Deep neural networks are good at extracting advantageous features.It can be used to retrieve multimodal medical images of various organs of the human body and improve the ranking performance in the case of small samples. Compared with traditional methods, deep learning-based methods such as scale-invariant feature transform (SIFT), local binary pattern (LBP) and histogram of oriented gradients (HOG) can show better performance.
In OBIA, researchers used EfficientNet as a feature extractor based on multimodal cancer data from the cancer imaging database TCIA, trained the model using a triplet network and an attention module, and compressed the image into a discrete hash value (Figure 2). Subsequently, to speed up inference performance and reduce inference latency, the trained model was converted to the TensorRT format and Faiss was used to store the hash code.
The researchers used the Hamming distance to calculate the image similarity and returned the most similar image.The results show that the mean average precision (MAP) value of the proposed model exceeds the performance of existing advanced image retrieval models on the TCIA dataset.
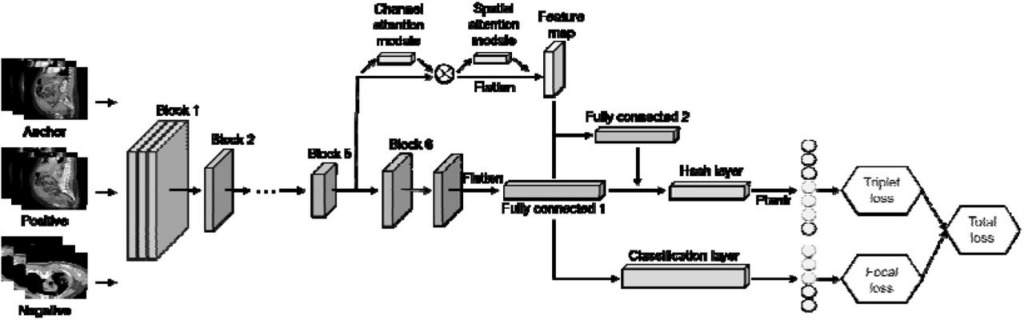
Figure 2: Deep triplet hashing based on attention and layer fusion modules
The model uses EfficientNet-B6 as the main network and uses the CBAM attention module in Block5 to obtain feature maps. Layer fusion is used in the fully connected layer, and the focus loss and triplet loss are used to generate hash codes and class embeddings.
Note:
● CBAM: convolutional block attention module
● EfficientNet: A new type of CNN network proposed by Google in 2019, which has extremely high parameter efficiency and speed and performs well in the field of image classification
● Faiss: A high-performance similarity search library developed by Facebook AI Research, commonly used in deep learning
Database Content and Usage - Data Model
As shown in Figure 3,Imaging data in OBIA is divided into five object types:Collection, Individual, Study, Series, Image, respectively refer to:
• Collections:Prefixed with "OBIA" to provide an overall description of the complete submission;
• Individual:Registration numbers are prefixed with an "I" and define the characteristics of the human or non-human organism receiving or registered to receive health care services;
• Study:The accession number is prefixed with "S" and contains descriptive information about the individual's radiological examination;
• Series:The study can be divided into one or more series according to different logics (such as body part or direction);
• Image:Describes the pixel data of a single DICOM file (Digital Imaging and Communications in Medicine). An Image is associated with a single Series in a single Study.
Note: DICOM is an international standard widely used in the field of medical imaging. It defines a set of specifications and protocols for storing, transmitting, sharing and printing medical imaging data, so that medical equipment and software produced by different manufacturers can be compatible and communicate with each other.
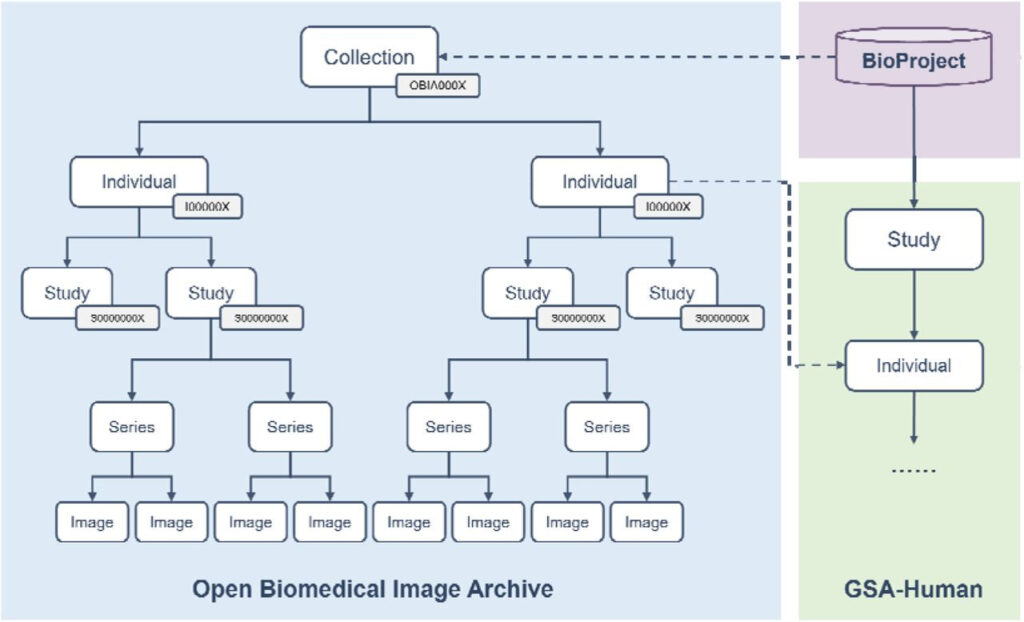
Figure 3: OBIA data model
Based on these standardized data objects,OBIA connects the image structure defined by the DICOM standard with actual research projects.Data sharing and exchange are realized.
In addition, each Collection in OBIA is linked to BioProject to provide descriptive metadata about the research project;
Where available, OBIA's Individual can be linked via the Individual accession number to GSA-Human, which links imaging data with genomic data for researchers to perform multi-omics analyses.
BioProject URL:
https://ngdc.cncb.ac.cn/bioproject/
GSA-Human link address:
https://ngdc.cncb.ac.cn/gsa-human/
Database Content and Use——De-identification and quality control
Biomedical images may contain protected health information (PHI) and need to be properly processed to minimize the risk of violating personal privacy. In order to retain as much valuable scientific information as possible while removing PHI,OBIA provides a de-identification and quality control mechanism that complies with the DICOM standard (Figure 4).
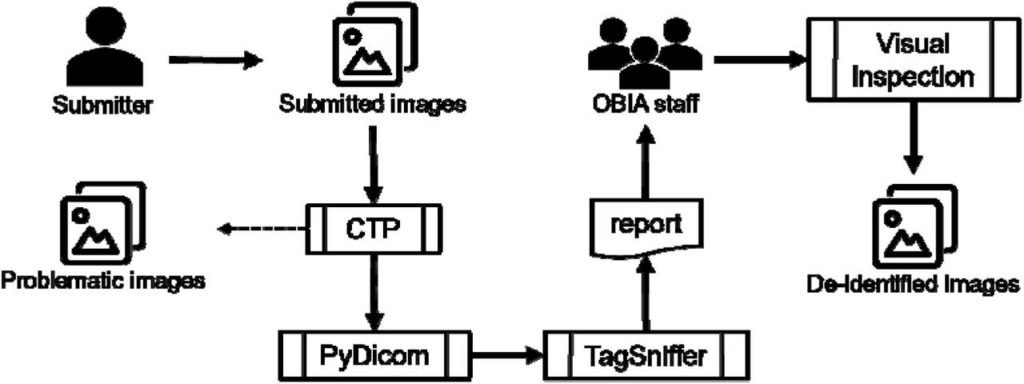
Figure 4: OBIA de-identification and quality control mechanisms
OBIA uses the Radiological Society of North America (RSNA) MIRC Clinical Trial Processor (CTP) to perform much of the de-identification work:
• For standard tags,The researchers built a CTP and developed a universal base de-identification script to remove or anonymize certain standard markers that contain or may contain PHI;
• For private tags,Use PyDicom to process it, preserving its purely digital nature.
After the de-identification process is complete, OBIA begins running quality control procedures:
• The image in question:Isolate images, where submitters can provide relevant information to repair the image or discard it completely (such images are those with blank titles or missing patient IDs, damaged, mixed with other patient images, etc.);
• Duplicate image:Keep only one.
OBIA then uses TagSniffer to generate a report for all images where all DICOM elements are carefully reviewed to ensure they do not contain PHI and that certain values (eg, patient ID, study date) are modified as expected.
also,OBIA staff also perform visual inspections of image pixels.to ensure that no PHI is included in the pixel values and that the image is visible and uncorrupted.
Database Content and Use——Statistics
As of September 2023, OBIA has collected 937 "Individuals", 4,136 "Studies", 24,701 "Series" and 1,938,309 "Images", covering 9 modalities and 30 anatomical parts.
Representative imaging modalities include X-ray computed tomography (CT), magnetic resonance (MR), and digital radiography (DX), and anatomical sites include abdomen, chest, thorax, head, liver, pelvis, etc.
The first batch of data submitted to OBIA came from 301 Hospital.Includes imaging data for 3 major gynecological tumors (endometrial cancer, ovarian cancer, and cervical cancer).
As shown in Table 1, these data are divided into four "Collections", listing the number of "Individual", "Study", "Series" and "Image". In addition,OBIA also collects relevant clinical metadata.Such as demographic data, medical history, family history, diagnosis, pathology type and treatment method.

Table 1: First batch of information submitted to OBIA
Breaking down data barriers,Building medical data sharing platforms at home and abroad
Data will only generate value when it is circulated. In order to improve the level of sharing of biological imaging data,Many countries around the world are committed to building open medical databases:
• National Institutes of Health (NIH):Sponsored several knowledge bases, such as MIDRC, an open access platform for COVID-19-related medical images and data, IDA, NITRC-IR, FITBIR, OpenNeuro and NDA, which collect neural and brain imaging, and TCIA and IDC, cancer imaging databases (TCIA provides images locally and IDC provides images in a cancer research data sharing cloud environment);
• Cancer Research UK:sponsored the OPTIMAM Mammography Image Database (OMI-DB);
• University of Porto, Portugal:sponsored the Breast Cancer Digital Repository (BCDR), which provides annotated breast cancer images and clinical details;
In the above repositories, except NITRC-IR and IDC,Most others support data de-identification and quality control.In addition, some universities or institutions also provide open source data sets, such as OASIS, EchoNet-Dynamic, CAMUS project, etc.
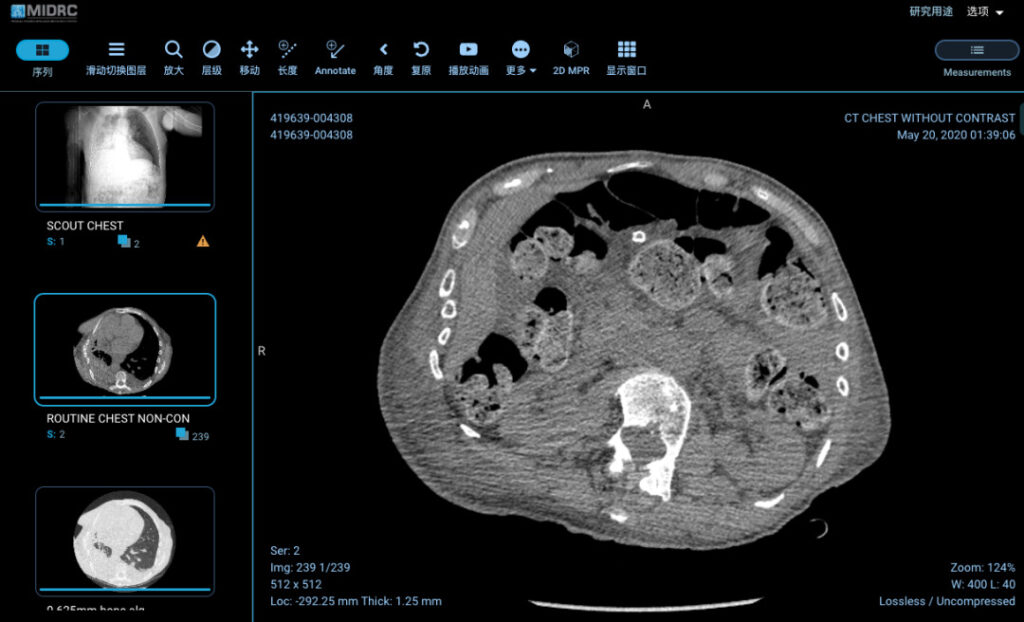
Figure 5: Chest CT of a 79-year-old patient in the MIDRC database
in the country,Huazhong University of Science and Technology provides open resources of integrated CT images and CFs of COVID-19.It includes CT images and clinical characteristics of patients with pneumonia (including COVID-19), but is limited to a single disease and available research resources are limited. Therefore, there is still a lack of databases in China that specialize in storing and accepting submissions of various diseases and modality data.
OBIA established by the Chinese Academy of Sciences fills the gap in the open sharing of domestic biomedical imaging data, which facilitates researchers from different institutions to share clinically relevant imaging data and can effectively bridge the gap in China's biomedical imaging database field.
The researchers stated in the paper that they will continue to upgrade OBIA's infrastructure and increase security measures in the future. They will also collect more types of biomedical imaging data and expand data sources.We are taking multiple measures to move towards the goal of "retaining as much valid image metadata as possible and providing high-quality imaging data for scientific researchers."
-- over--