DeepMind Uses Unsupervised Learning to Develop AlphaMissense, Predicting 71 Million Gene Mutations
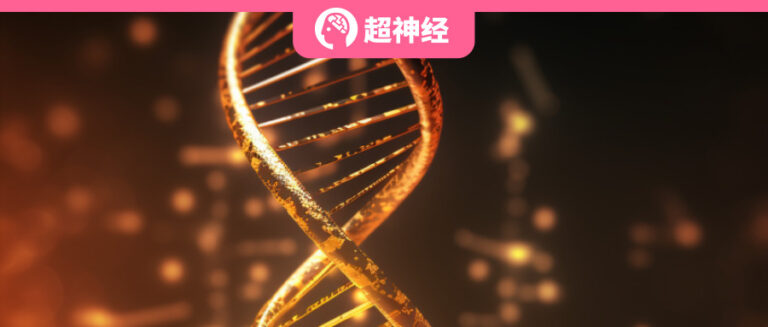
The human genome has a total of 3.16 billion base pairs, which are constantly undergoing replication, transcription and translation, and are at risk of errors and mutations at any time.
Missense mutation is a common form of gene mutation, but humans have only observed a small part of it so far, and only 0.1% can be interpreted.
Accurately predicting the effects of missense mutations plays an important role in the research and prevention of rare diseases and genetic diseases. This time, DeepMind has taken action again.
Author | Xuecai
Editor | Three Sheep, Iron Tower
This article was first published on HyperAI WeChat public platform~
The human genome has a total of 3.16 billion base pairs. These base pairs undergo replication, transcription, and translation every day, and are ultimately expressed as proteins that regulate human daily physiological activities.
With such a huge workload, even the delicate human body would find it difficult to achieve perfect errors.If you are not careful, the base pairs may be misaligned, leading to gene mutations, and even cancer over time.
Missense mutation is a common form of gene mutation.Due to base mutations in DNA, the translated amino acids change, ultimately leading to the destruction of the entire protein function.
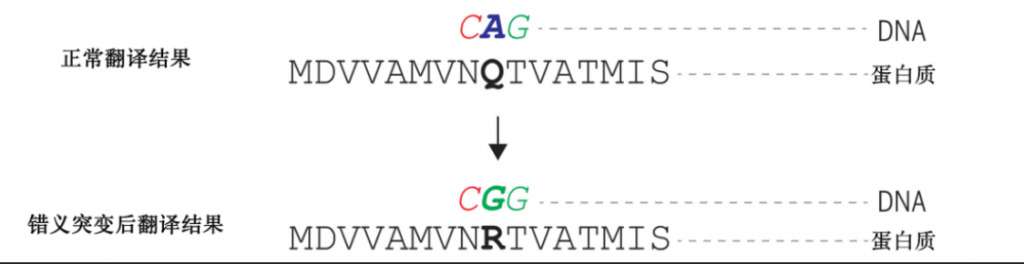
Figure 1: Schematic diagram of missense mutation.Due to the mutation of adenine nucleotides to guanine nucleotides in DNA,The amino acid converted from glutamine to serine
Currently, more than 4 million missense mutations have been observed in humans, but only the missense mutations of 2% can be classified as pathogenic mutations or benign mutations.
Accurately predicting the effects of missense mutations can deepen our understanding of rare diseases and prevent and treat potential genetic diseases.Although multiplex analysis of variant effects (MAVEs) can systematically analyze protein mutations and accurately predict their clinical effects,But this method requires a lot of manpower and material resources.It is difficult to perform a comprehensive analysis of all possible missense mutations.
To this end, DeepMind analyzed the overall structure of the protein through AlphaFold.We developed AlphaMissense by combining weak label learning and unsupervised learning.A systematic analysis of the consequences of missense mutations was performed. AlphaMissense was validated using the ClinVar dataset.The prediction accuracy reached 90%.
Then,AlphaMissense predicts 71 million possible missense mutations in humans, among which 32% may be a pathogenic mutation and 57% may be a benign mutation.These results will greatly promote the development of disciplines such as molecular biology, genomics, and clinical medicine.This result has been published in "Science".

Figure 2: AlphaMissense's prediction results for 71 million missense mutations (top) and the results currently observed and confirmed by humans (bottom)
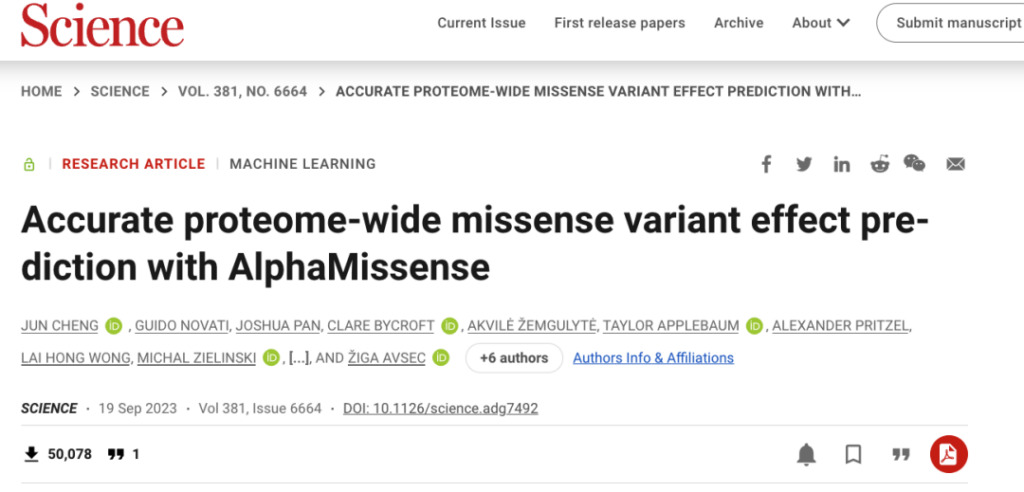
Related results have been published in "Science"
Paper link:
https://www.science.org/doi/10.1126/science.adg7492
Experimental procedures
AlphaMissense:AlphaFold + Fine-tuning
When an amino acid sequence is input into AlphaMissense, it predicts the pathogenicity of any amino acid change in the sequence. The implementation of AlphaMissense is very similar to AlphaFold, with only minor adjustments to the architecture.
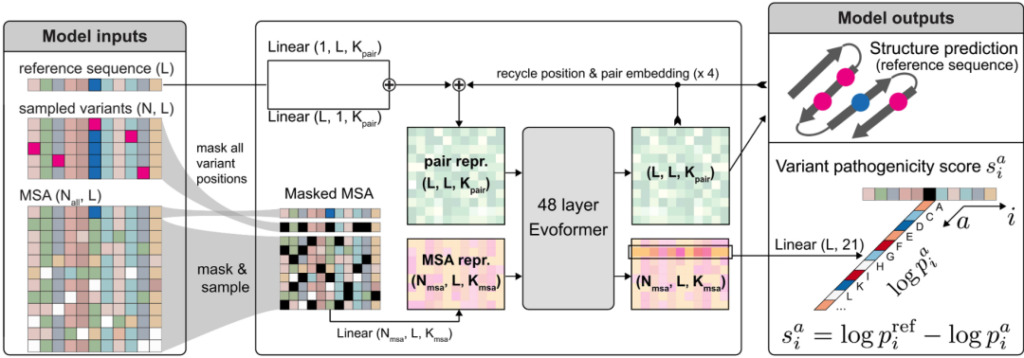
Figure 3: AlphaMissense structure diagram
AlphaMissense’s training data comes from a wide range of sources, but primarily from humans and non-human primates.Among them, there are 1,248,533 benign missense mutations originating from humans, and pathogenic missense mutations are extracted from 65,314,044 mutations that may occur but have not yet been observed.
The training of AlphaMissense consists of two steps. First, like AlphaFold, AlphaMissense needs to predict the randomly masked amino acids in multiple sequence alignments.Then predict the structure of single-chain proteins and perform protein language modeling.
Then,Researchers fine-tuned AlphaMissense using human proteins.And the output target of the model was set, namely the pathogenicity of the missense mutation.
Since there are a considerable number of benign mutations among the unobserved missense mutations, but they are classified as pathogenic mutations during training, the AlphaMissense training set is very noisy.In order to improve the quantity and quality of the training set, the researchers filtered the data using self-distillation.
Clinical data verification:Performance in different datasets
After training is completed,AlphaMissense was validated using annotated clinical data (ClinVar dataset), de novo variants in patients with rare developmental disorders, and MAVE results in ProteinGym.
First, the researchers evaluated the performance of AlphaMissense in the ClinVar dataset. After analyzing 18,924 mutation sites,The auROC of AlphaMissense is 0.940, which is an improvement over the previous state-of-the-art evolutionary model (EVE) (0.911).
When evaluating missense mutations clinically, people generally focus on genes associated with specific diseases. Therefore, it is particularly important to distinguish between benign and pathogenic missense mutations in these genes. The researchers used AlphaMissense to analyze 612 genes in ClinVar.Its auROC is 0.950, which is better than EVE's 0.921.
Finally, the researchers analyzed the prediction results of AlphaMissense in the Deciphering Developmental Disorders (DDD) dataset. The auROC of AlphaMissense is 0.809, which is comparable to 0.797 of PrimateAI.
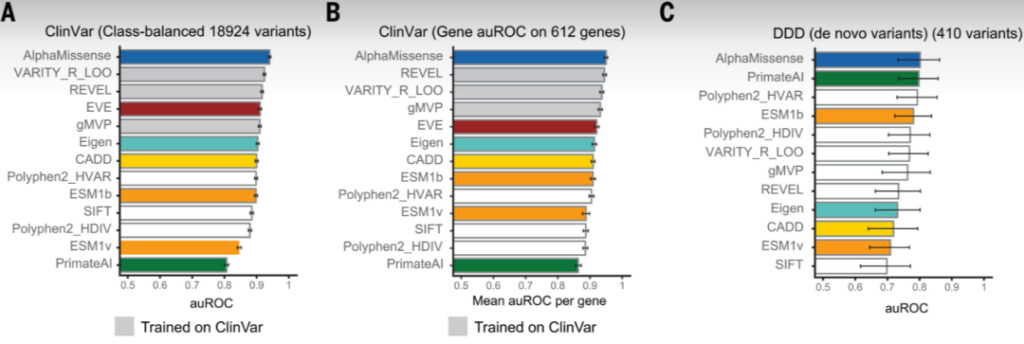
Figure 4: Performance comparison of AlphaMissense and other models in different datasets
A: Analysis of mutation sites in ClinVar;
B: Analysis of genes in ClinVar;
C: Analysis of the DDD dataset.
At the same time, AlphaMissense's prediction results for Cancer Hotspots, ACMG (American College of Medical Genetics) and other MAVE data are better than other models.The above results show that AlphaMissense outperforms existing models in multiple datasets.
Overall prediction performance:Reflecting protein mutation trends
After verifying AlphaMissense with clinical data,The researchers used AlphaMissense to predict possible mutations of 216 million amino acids in 19,233 common proteins in humans, and ultimately obtained predictions for 71 million missense mutations.
AlphaMissense's pathogenicity prediction results are between 0 and 1, and the closer to 1, the higher the possibility of pathogenicity. Since most of the prediction results are close to 0 and 1, the data between 0.2 and 0.8 may not be very accurate. In the end, they divided the prediction results into three categories:Possibly pathogenic, possibly benign, and undetermined.
To evaluate the predictive performance of AlphaMissense as a whole, the researchers calculated the pathogenicity of individual amino acids for all proteins.Mutations in aromatic amino acids and cysteine are more likely to cause disease, which is consistent with the actual results.Because these two amino acids play a role in maintaining the structure of protein.

Figure 5: AlphaMissense prediction results heat map,The color blocks represent the average pathogenicity of 216 million amino acid changes in the proteome
After visualizing the prediction results of AlphaMissense and the protein structures predicted by AlphaFold, we can see the mutation trends of these proteins.For example, regions with disordered protein structure correspond to regions where benign mutations occur, which is consistent with the prediction results of proteomics.
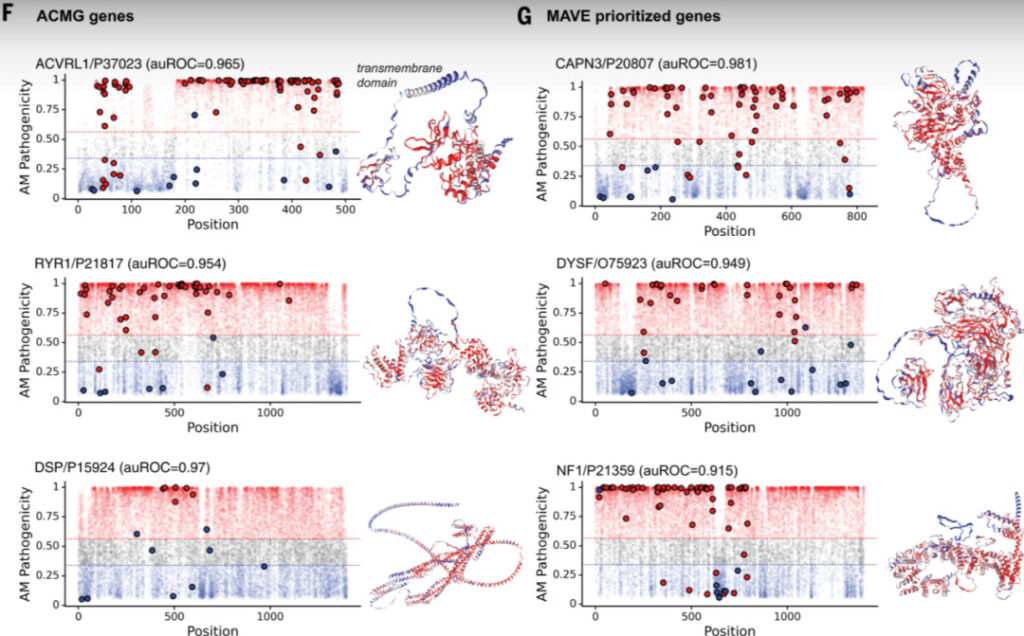
Figure 6: Visualization results of some proteins in ACMG and MAVE datasets
On the left is the pathogenicity predicted by AlphaMissense, with possible pathogenic missense mutations in red and possible benign missense mutations in blue. Mutations that have been included in the ClinVar dataset are marked with solid circles. On the right is the protein structure predicted by AlphaFold, with different colors indicating the pathogenicity of mutations in this region, corresponding to AlphaMissense.
Prediction accuracy:Consistency with MAVE results
To investigate the consistency between AlphaMissense and MAVE results, the researchers analyzed two sets of MAVE data using AlphaMissense.Compared with other prediction methods, AlphaMissense is closest to the MAVE data.

Figure 7: Spearman correlation coefficient of AlphaMissense and other models with MAVE prediction results,Among them, AlphaMissense has the best result
They then compared AlphaMissense's prediction data with the pathogenicity of missense mutations verified by experiments. SHOC2 protein can form a complex with MRAS and PP1C proteins to activate the Ras-MAPK cancer pathway. AlphaMissense and MAVE predicted the correlation between this mutation and Ras cancer cells.The obtained Spearman correlation coefficient is 0.47, which is better than other models. (ESM1v: 0.41, ESM1b: 0.40, EVE: 0.32).

Figure 8: Prediction results of different models for missense mutations in the MAVE dataset
Furthermore, the researchers explored the prediction results of AlphaMissense on the pathogenicity of amino acid missense mutations in different regions of the SHOC2 protein. Among the first 80 amino acids of SHOC2, MAVE predicted that mutations in amino acids 63-74 are pathogenic because this region binds to the PP1C protein through RVxF. AlphaMissense is the only model that identifies this important region.
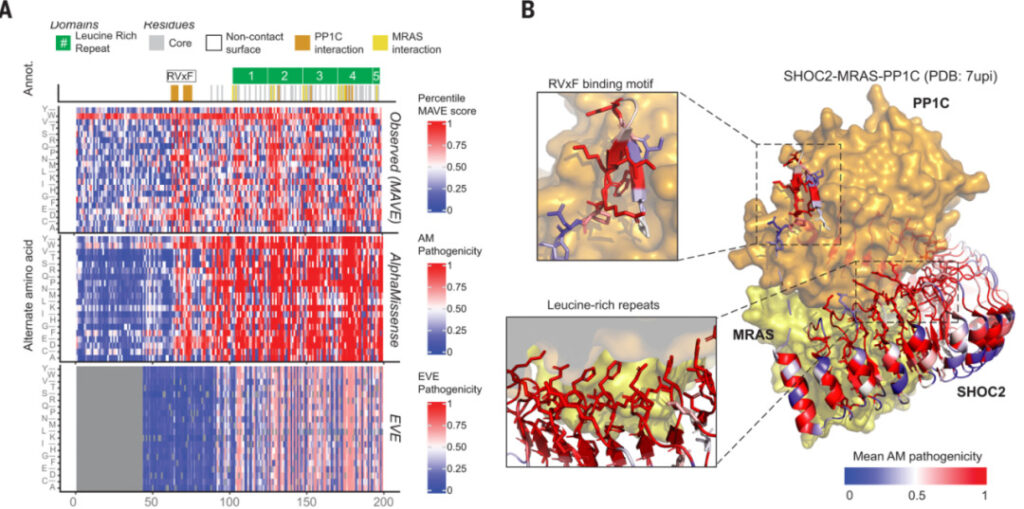
Figure 9: AlphaMissense prediction results for SHOC2 protein
A: The prediction results of different models for the pathogenicity of the first 200 amino acid mutations of SHOC2 protein. From top to bottom, they are actual situation (MAVE), AlphaMissense and EVE;
B: The structural diagram of the complex composed of SHOC2 protein (red and blue) and MRAS (yellow) and PP1C (gold) proteins.
Moreover, AlphaMissense can reflect the results of different types of amino acid missense mutations.For SHOC2 protein, the prediction results of AlphaMissense are closest to the actual results.

Figure 10: Correlation between different models for the prediction of pathogenicity of amino acid mutations in SHOC2 and MAVE results
The above results collectively indicate that the prediction results of AlphaMissense are comparable to those of MAVE and can accurately predict the outcomes of gene missense mutations.
Finally, Deepmind made the model and prediction results open source to the community, hoping that the conclusions could help research in other disciplines.
Model link:
https://github.com/deepmind/alphamissense
Gene mutation: out of reach yet always there
When it comes to gene mutation, we tend to think of dangerous elements such as X-rays, nuclear radiation, nitrite, or scenes from the movies Resident Evil and The Hulk, and feel that these are too far away from us. It is true that we are exposed to very little radiation in our daily lives.But gene mutations still happen every moment in our lives and actually change our lives.
In life, we are inevitably exposed to radiation sources., such as sunlight. The radiation in sunlight comes from ultraviolet rays, which are one of the carcinogenic factors. Therefore, long-term exposure to the sun will increase the risk of skin cancer.
Even without exposure to radiation sources,DNA inevitably makes some mistakes during replication, transcription, and translation, causing gene mutations., but these mutations may be benign or cleared in time by the immune mechanism.
But at the same time, gene mutations also provide convenience for our lives.Especially in agricultural productionCrop mutants can increase crop yields, improve crop tolerance to salt and alkali, and even help control pests. After breeding and screening these mutants, these excellent characteristics can be retained and food production can be increased.
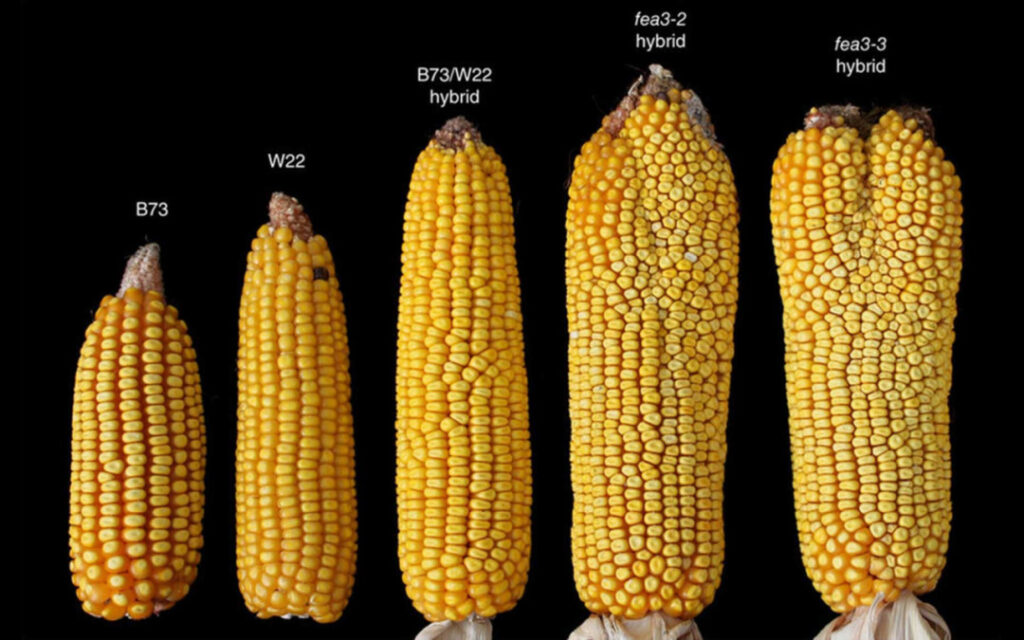
Figure 11: Different varieties of corn mutants
However, there are too many possibilities for human gene mutations, and what we know so far is just a drop in the ocean. With AlphaMissense, we can make relatively reliable predictions about the results of gene mutations, and then infer them in reverse.Perhaps we can find the mechanisms behind genetic diseases and rare diseases and provide new methods for disease prevention and treatment.
At the same time, AlphaMissense also provides materials for research in other fields. Perhaps in the near future, we will be able to see AlphaMissense's interpretation of gene mutations in other species.We can then make rational use of gene mutations and let genetic engineering bring more benefits to our lives.
Reference Links:
[1]https://www.science.org/doi/10.1126/science.abj6987
[2]https://www.cshl.edu/discovery-of-new-stem-cell-pathway-indicates-route-to-much-higher-yields-in-maize-staple-crops/
This article was first published on HyperAI WeChat public platform~