Interview With UIUC Li Bo | From Usability to Trustworthiness, the Ultimate Thinking of the Academic Community on AI
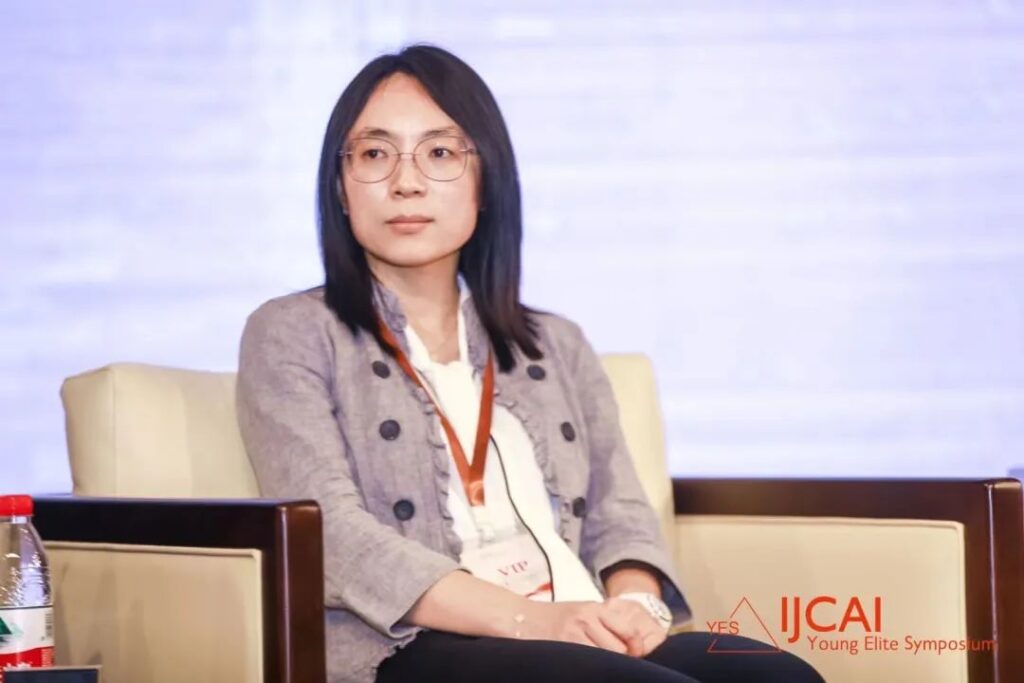
This article was first published on HyperAI WeChat public platform~
The emergence of ChatGPT has once again caused a stir in the technology circle over AI, which has far-reaching impacts and has divided the technology community into two camps. One camp believes that the rapid development of AI may replace humans in the near future. Although this "threat theory" is not without reason, the other camp has also put forward different views.The intelligence level of AI has not yet surpassed that of humans and is even "not as good as that of dogs", and it is still a long way from endangering the future of mankind.
Admittedly, this debate deserves early warning, but as Professor Zhang Chengqi and other experts and scholars pointed out at the 2023 WAIC Summit Forum,Human expectations of AI are always a useful tool.Since it is just a tool, compared to the "threat theory",The more important issue is whether it is credible and how to improve its credibility.After all, once AI becomes untrustworthy, what about its future development?
So what is the standard of credibility, and where is the field today?HyperAI was fortunate to have an in-depth discussion with Li Bo, a cutting-edge scholar in this field, an associate professor at the University of Illinois, who has won many awards including the IJCAI-2022 Computers and Thought Award, the Sloan Research Award, the National Science Foundation CAREER Award, AI's 10 to Watch, the MIT Technology Review TR-35 Award, and the Intel Rising Star. Following her research and introduction, this article sorted out the development context of the field of AI security.
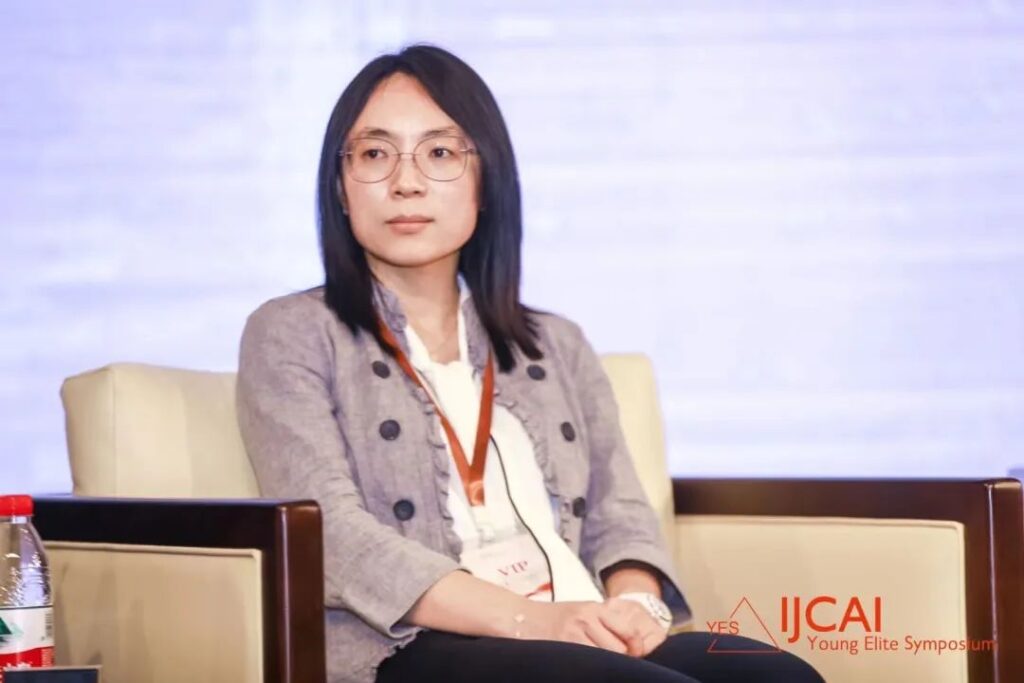
Li Bo at 2023 IJCAI YES
Machine learning is a double-edged sword
Looking at the timeline in a longer term, Li Bo’s research journey is also a microcosm of the development of trusted AI.
In 2007, Li Bo entered the undergraduate program of information security. During that period, although the domestic market had already awakened to the importance of network security and began to develop firewalls, intrusion detection, security assessment and other products and services, overall, the field was still in its development stage. Looking back now, this choice was risky, but it was the right start.Li Bo started his own security research journey in such a still "new" field, and at the same time, laid the groundwork for subsequent research.

Li Bo studied information security at Tongji University
At the doctoral level,Li Bo will further focus on AI security.The reason why she chose this not-so-mainstream field was not only because of her interest, but also largely due to the encouragement and guidance of her mentor. This major was not particularly mainstream at the time, and Li Bo's choice was quite risky. However, even so, she relied on her undergraduate experience in information security to keenly grasp that the combination of AI and security would be very bright.
At that time, Li Bo and his supervisor were mainly engaged in research from the perspective of game theory.Model the attack and defense of AI as a game, such as using the Stackelberg game for analysis.
Stackelberg games are often used to describe the interaction between a strategic leader and a follower. In the field of AI security, they are used to model the relationship between attackers and defenders. For example, in adversarial machine learning, attackers try to trick machine learning models into producing incorrect outputs, while defenders work to discover and prevent such attacks. By analyzing and studying Stackelberg games,Researchers such as Li Bo can design effective defense mechanisms and strategies to enhance the security and robustness of machine learning models.

Stackelberg game model
In 2012-2013, the popularity of deep learning accelerated the penetration of machine learning into all walks of life. However, even though machine learning is an important force driving the development and transformation of AI technology, it is difficult to hide the fact that it is a double-edged sword.
On the one hand, machine learning can learn and extract patterns from large amounts of data, achieving outstanding performance and results in many fields.For example, in the medical field, it can assist in diagnosing and predicting diseases, providing more accurate results and personalized medical advice;On the other hand, machine learning also faces some risks.First of all, the performance of machine learning is highly dependent on the quality and representativeness of the training data. Once the data has problems such as bias and noise, it is very easy for the model to produce erroneous or discriminatory results.
In addition, the model may also rely on private information, which may lead to the risk of privacy leakage. In addition, adversarial attacks cannot be ignored. Malicious users can intentionally deceive the model by changing the input data, resulting in incorrect output.
Against this backdrop, Trusted AI came into being and developed into a global consensus in the following years. In 2016, the Legal Affairs Committee of the European Parliament (JURI) issued a draft report on legislative recommendations to the European Commission on civil law rules for robots, advocating that the European Commission should assess the risks of artificial intelligence technology as soon as possible. In 2017, the European Economic and Social Committee issued an opinion on AI, arguing that a standard system of AI ethics and monitoring certification should be established. In 2019, the EU issued the Ethical Guidelines for Trustworthy AI and the Algorithmic Responsibility and Transparency Governance Framework.
In China, Academician He Jifeng first proposed the concept of trusted AI in 2017. In December 2017, the Ministry of Industry and Information Technology issued the "Three-Year Action Plan to Promote the Development of the New Generation of Artificial Intelligence Industry". In 2021, the China Academy of Information and Communications Technology and JD Discovery Research Institute jointly released the first "Trusted Artificial Intelligence White Paper" in China.

"Trustworthy Artificial Intelligence White Paper" press conference
The rise of the field of trusted AI has made AI move in a more reliable direction and also confirmed Li Bo’s personal judgment.Devoted to scientific research and focused on machine learning confrontation, she followed her own judgment and became an assistant professor at UIUC. Her research results in the field of autonomous driving, "Robust physical-world attacks on deep learning visual classification", were permanently collected by the Science Museum in London, UK.
With the development of AI, the field of trusted AI will undoubtedly usher in more opportunities and challenges. “I personally think that security is an eternal topic. With the development of applications and algorithms, new security risks and solutions will emerge. This is the most interesting point of security. AI security will keep pace with the development of AI and society.” Li Bo said.
Exploring the current status of the field from the credibility of large models
The emergence of GPT-4 has become the focus of everyone's attention. Some people think it has set off the fourth industrial revolution, some people think it is the turning point of AGI, and some people have a negative attitude towards it. For example, Turing Award winner Yann Le Cun once publicly stated that "ChatGPT does not understand the real world, and no one will use it in five years."
In this regard, Li Bo said that she was very excited about this wave of big model enthusiasm, because this wave of enthusiasm has undoubtedly really promoted the development of AI, and such a trend will also put higher demands on the field of trusted AI, especially in some fields with high security requirements and high complexity such as autonomous driving, smart medical care, biopharmaceuticals, etc.
At the same time, more new application scenarios of trusted AI and more new algorithms will emerge. However, Li Bo also fully agrees with the latter point of view.The current models have not yet truly understood the real world. The latest research results of her and her team show that large models still have many loopholes in terms of trustworthiness and security.
Li Bo and his team's research mainly targets GPT-4 and GPT-3.5. They discovered new threat vulnerabilities from eight different angles, including toxicity, stereotype bias, adversarial robustness, out-of-distribution robustnes, robustness of generating demonstration samples in in-context learning, privacy, machine ethics, and fairness in different environments.

Paper address:
https://decodingtrust.github.io/
Specifically, Li Bo and his team found that the GPT model is easily misled, producing abusive language and biased responses, and it may also leak private information in training data and conversation history. At the same time, they also found that although GPT-4 performed more trustworthy than GPT-3.5 in standard benchmark tests, GPT-4 is more vulnerable to attacks due to the adversarial jailbreak system and user prompts, which is due to the fact that GPT-4 follows instructions more accurately, including misleading instructions.
Therefore, from the perspective of reasoning ability, Li Bo believes that the arrival of AGI is still a long way off, and the primary problem that lies ahead is to solve the credibility of the model.In the past, Li Bo's research team has also focused on developing a logical reasoning framework based on data-driven learning and knowledge enhancement, hoping to use knowledge bases and reasoning models to make up for the shortcomings of the credibility of large data-driven models. Looking to the future, she also believes that there will be more new and excellent frameworks that can better stimulate the reasoning ability of machine learning and make up for the threat vulnerabilities of the model.
So can we get a glimpse of the general direction of the field of trusted AI from the current state of trust in large models? As we all know,Stability, generalization ability (explainability), fairness, and privacy protection are the foundation of trustworthy AI and are also four important sub-directions.Li Bo believes that the emergence of large models and new capabilities will inevitably bring new credibility limitations, such as robustness to adversarial or out-of-distribution examples in contextual learning. In this context, several sub-directions will promote each other, thereby providing new information or solutions to the essential relationship between them. "For example, our previous research has proved that the generalization and robustness of machine learning can be two-way indicators in federated learning, and the robustness of the model can be regarded as a function of privacy, etc."
Looking forward to the future of trusted AI
Looking back at the past and present of the field of trusted AI, we can see that the academic community represented by Li Bo, the industry represented by technology giants, and the government are all exploring in different directions and have achieved a series of results. Looking to the future,Li Bo said, "The development of AI is unstoppable. Only by ensuring safe and reliable AI can we safely apply it to different fields."
How to build trustworthy AI? To answer this question, we must first think about what it means to be “trustworthy”. “I think establishing a unified and trustworthy AI evaluation standard is one of the most critical issues at present.”It can be seen that at the just-concluded Zhiyuan Conference and the World Artificial Intelligence Conference, the discussion of trusted AI was unprecedentedly high, but most of the discussions remained at the discussion level, lacking a systematic methodological guidance. The same is true in the industry. Although some companies have launched relevant toolkits or architecture systems, the patch-style solution can only solve a single problem. Therefore, many experts have repeatedly mentioned the same point of view - there is still a lack of a trusted AI evaluation specification in the field.
Li Bo was deeply touched by this."The prerequisite for a guaranteed trustworthy AI system is to have a trustworthy AI evaluation specification."She further said that her recent research "DecodingTrust" is aimed at providing a comprehensive model credibility assessment from different perspectives. Expanding to the industry, the application scenarios are becoming more complex, which brings more challenges and opportunities to the trusted AI evaluation. Because more trusted vulnerabilities may appear in different scenarios, this can further improve the trusted AI evaluation standards.
In summary,Li Bo believes that the future of the trusted AI field should focus on forming a comprehensive and real-time updated trusted AI evaluation system, and on this basis, improving the credibility of the model."This goal requires close collaboration between academia and industry to form a larger community to accomplish it together."
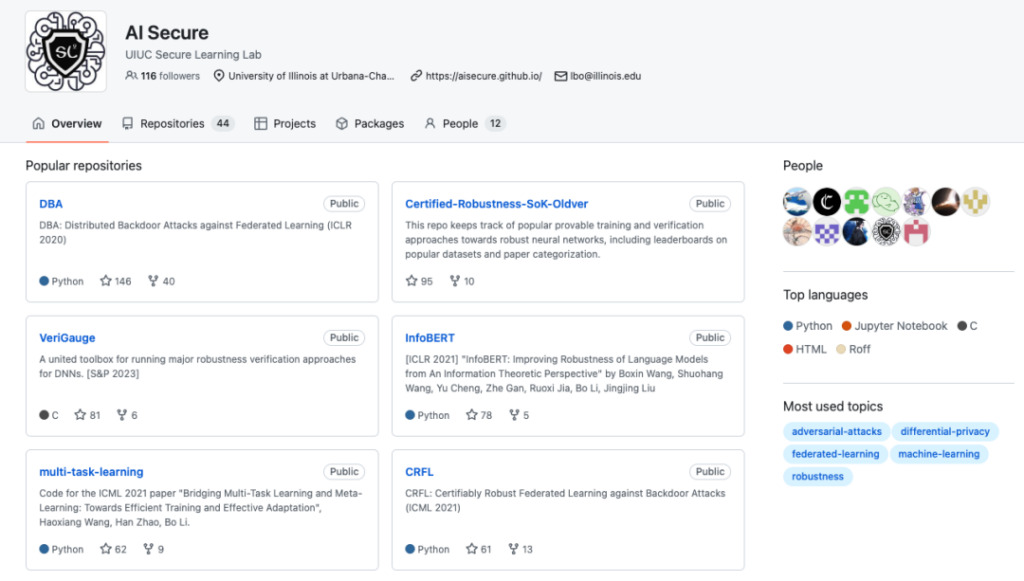
UIUC Secure Learning Lab GitHub homepage
GitHub project address:
At the same time, the Security Learning Laboratory where Li Bo works is also working towards this goal.Their latest research results are mainly distributed in the following directions:
1. A verifiable and robust knowledge-enhanced logical reasoning framework based on data-driven learning is designed to combine data-driven models with knowledge-enhanced logical reasoning, thereby making full use of the scalability and generalization capabilities of data-driven models and improving the error correction capabilities of the model through logical reasoning.
In this direction, Li Bo and his team proposed a learning-reasoning framework and proved its certification robustness. The research results show that this framework can be proven to have obvious advantages over the method that only uses a single neural network model, and analyzes enough conditions. At the same time, they also extended the learning-reasoning framework to different task areas.
Related papers:
* https://arxiv.org/abs/2003.00120
* https://arxiv.org/abs/2106.06235
* https://arxiv.org/abs/2209.05055
2. DecodingTrust: The first comprehensive model credibility assessment framework for trust assessment of language models.
Related papers:
* https://decodingtrust.github.io/
3. In the field of autonomous driving, it provides a safety-critical scenario generation and testing platform "SafeBench".
Project address:
* https://safebench.github.io/
besides,Li Bo revealed that the team plans to continue to focus on smart healthcare, finance and other fields."Breakthroughs in trusted AI algorithms and applications may appear earlier in these areas."
From assistant professor to tenured professor: if you work hard, success will come naturally
From Li Bo's introduction, it is not difficult to see thatThere are still many problems that need to be solved in the emerging field of trusted AI.Therefore, whether it is the academic community represented by Li Bo's team or the industry, all parties are exploring at this time in order to fully cope with the burst of demand in the future. Just like Li Bo's dormancy and concentrated research before the rise of the field of trusted AI - as long as you are interested and optimistic, it is only a matter of time before you achieve success.
This attitude is also reflected in Li Bo's own teaching career. She has been working at UIUC for more than 4 years.This year he was awarded the title of tenured professor.She introduced that the evaluation of professional titles has a strict process, which includes research results, academic evaluations of other senior scholars, etc. Although there are challenges,But "as long as you work hard on one thing, everything else will fall into place naturally."At the same time, she also mentioned that the tenure system in the United States provides professors with more freedom and the opportunity to carry out some more risky projects. So for Li Bo, she will work with the team to try some new, high-risk projects in the future, "hoping to make further breakthroughs in theory and practice."
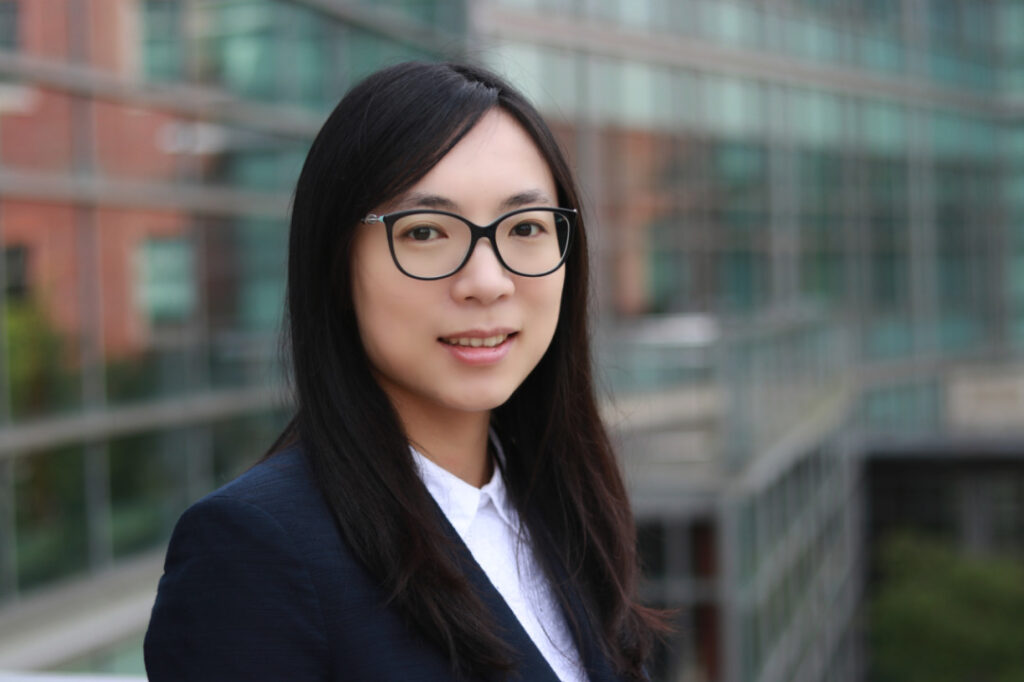
Associate Professor at the University of Illinois, winner of the IJCAI-2022 Computers and Thought Award, the Sloan Research Fellowship, the NSF CAREER Award, AI's 10 to Watch, the MIT Technology Review TR-35 Award, the Dean's Award for Research Excellence, the CW Gear Outstanding Junior Faculty Award, the Intel Rising Star Award, the Symantec Research Lab Fellowship, best paper awards from Google, Intel, MSR, eBay, and IBM, as well as multiple top machine learning and security conferences.
Research interests: Theoretical and practical aspects of trustworthy machine learning, which is the intersection of machine learning, security, privacy, and game theory.
Reference Links:
[1] https://www.sohu.com/a/514688789_114778
[2] http://www.caict.ac.cn/sytj/202209/P020220913583976570870.pdf
[3] https://www.huxiu.com/article/1898260.html
This article was first published on HyperAI WeChat public platform~