AI "anti-corruption", Germany's Max Planck Institute Combines NLP and DNN to Develop Corrosion-resistant Alloys
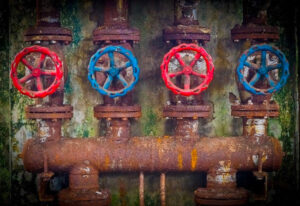
Contents at a glance:In a world surrounded by stainless steel, we may almost forget the existence of corrosion. However, corrosion exists in all aspects of life. Whether it is rusty steel nails, aging and leaking wires, or dull cars, all of these are related to corrosion. According to statistics, the economic losses caused by metal corrosion in the world exceed 2.5 trillion US dollars each year, far exceeding other natural disasters. Among them, the economic losses caused by corrosion in China are about 394.9 billion US dollars, accounting for 4.21% of China's GDP. Because of this, researchers have been exploring alloys or metal protective films with better corrosion resistance. Today, AI comes in handy in the process of optimizing the corrosion resistance of materials.
Keywords:Natural Language Processing Deep Neural Networks Corrosion
Author | Xuecai
Editor | Sanyang
This article was first published on HyperAI WeChat public platform~
According to statistics from the National Association of Corrosion Engineers (NACE), the economic losses caused by corrosion worldwide in 2013 exceeded 2.5 trillion US dollars.At the same time, China was also plagued by corrosion, with economic losses of approximately US$394.9 billion, accounting for 4.21% of its GDP that year, slightly higher than that of other developed countries.
By comparison, the economic losses caused by the 2008 Wenchuan earthquake were approximately US$110 billion.That is to say, as early as 2013, the economic losses caused by corrosion alone in our country exceeded three Wenchuan earthquakes.
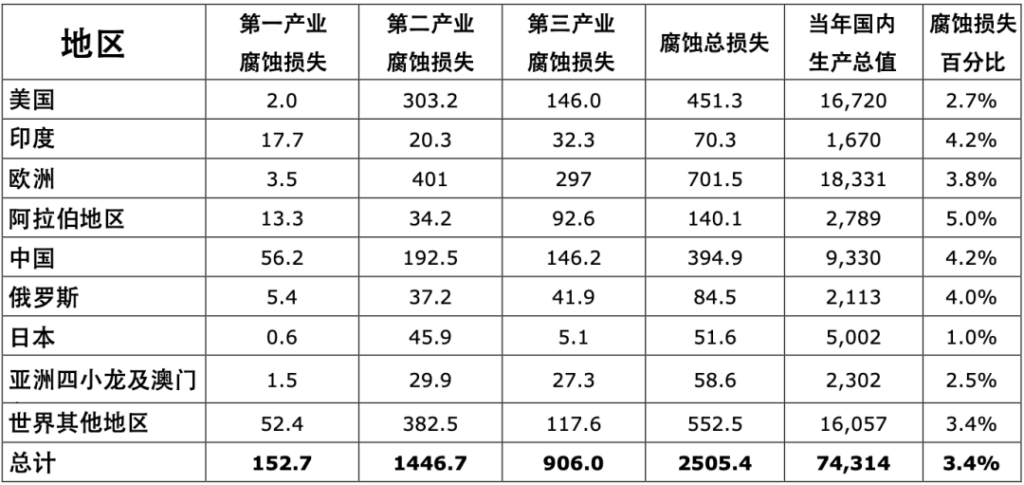
Table 1: Economic losses caused by corrosion around the world in 2013 (in billions of US dollars)
In order to solve the corrosion problem, researchers are working hard to improve the strength of materials while also constantly looking for ways to improve the corrosion resistance of materials.With the help of AI, they have made some progress, such as predicting the corrosion mechanism of alloys at high temperatures, analyzing the atmospheric corrosion rate of steel and the environmental corrosion of reinforced concrete, and being able to use convolutional neural networks (CNN) to determine the corrosion form of materials from images.
However, the input data of machine learning models are mostly numerical data. But in the processing and analysis of metal materials, in addition to numerical data such as pH value and test temperature, there are also categorical data such as material type and text data such as heat treatment process and test method.Traditional machine learning models are unable to thoroughly read and analyze all data, and their prediction accuracy is low.
To this end, the Max Planck Institute for Iron Research (MPIE, Max-Planck-Institut für Eisenforschung) in Germany Process-aware DNN was developed by combining deep neural network (DNN) and natural language processing (NLP).This model can combine numerical data and text data for processing, and its accuracy is 15% higher than other models.
At the same time, they converted the physical and chemical properties of metals into descriptors and constructed a feature transformation DNN, which can be used to predict the impact of elements not present in the training set on corrosion resistance. This research has been published in Science Advances in August 2023 with the title “Enhancing corrosion-resistant alloy design through natural language processing and deep learning”.
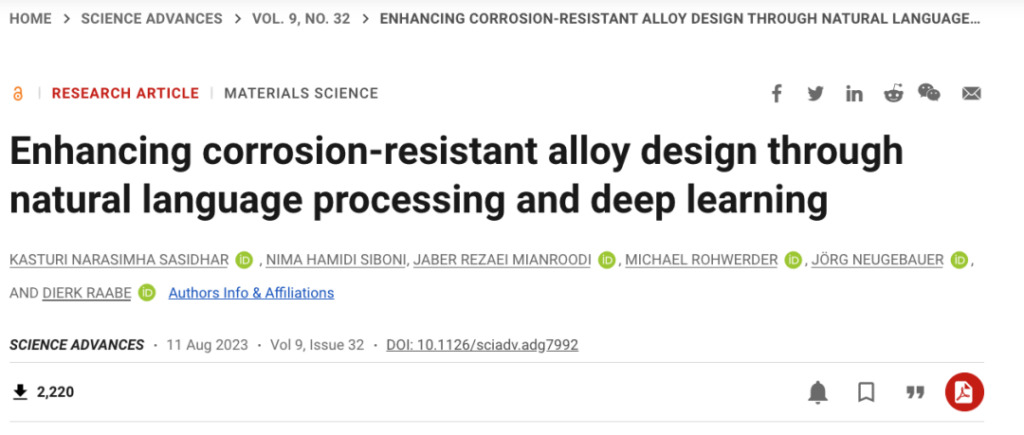
Related research has been published in Science Advances
Paper link:
https://www.science.org/doi/10.1126/sciadv.adg7992
Process-aware DNN
Model design
The data set for this study is the pitting potential of 769 alloys in 5 categories, which includes numerical data, categorical data, and text data. Among them, the numerical data is directly input into the model, and the categorical data is converted into numerical input model through sequential numbering.The text data is processed through the NLP architecture and then input into the model.
The NLP architecture is mainly divided into three parts, including vocabulary tagging, vectorization, and processing of vector sequences.
During word tagging, each word is replaced by a specific integer token.Through vocabulary tagging, a phrase or sentence is converted into an integer vector.
After word tagging, although the text data is converted into numerical values, there is no correlation between the numerical values and they cannot carry the semantics of the original text. Therefore, the integer vector is converted into an n-dimensional floating-point vector through vectorization. During the training process, the weight of each word is continuously optimized.After training, the proximity between vectors corresponds to their semantic similarity.
Finally, the n-dimensional floating-point vector is converted into a single vector through a long short-term memory recurrent neural network (LSTM) and enters the input layer. LSTM can identify long-term dependencies between words through gate functions.Therefore, LSTM can find key relevant words from a given sentence and pass the most important part of the sentence to the input layer of the DNN.
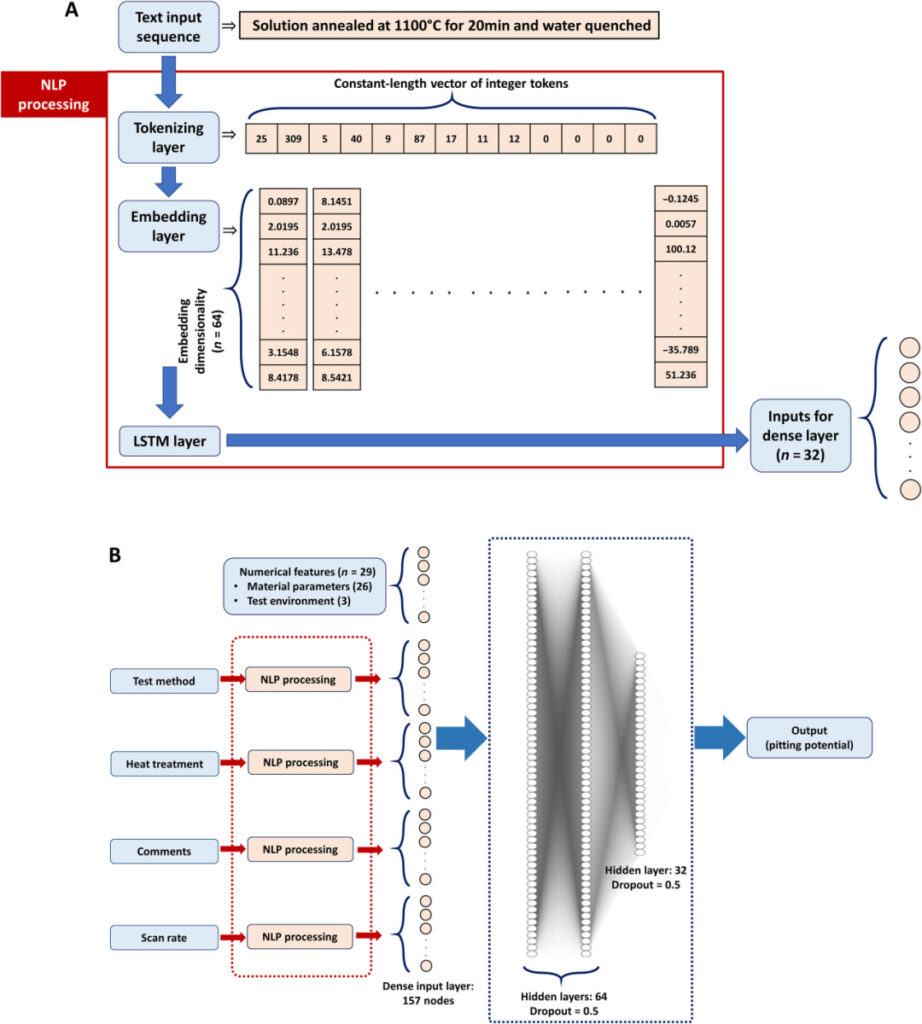
Figure 1:Process-aware DNN model structure
A: NLP data processing workflow
B: Schematic diagram of process-aware DNN model
Training and validation
After training, the researchers summarized the absolute mean error of the models. The average absolute error of the process-aware DNN was about 150 mV, which was 20 mV lower than that of the simple DNN. The R between the predicted pitting potential and the actual pitting potential2 It is 0.78 ± 0.06, which is higher than 0.61 ± 0.04 of the simpler DNN.The above results show that after analyzing text data, the performance of process-aware DNN is better than that of simple DNN model.
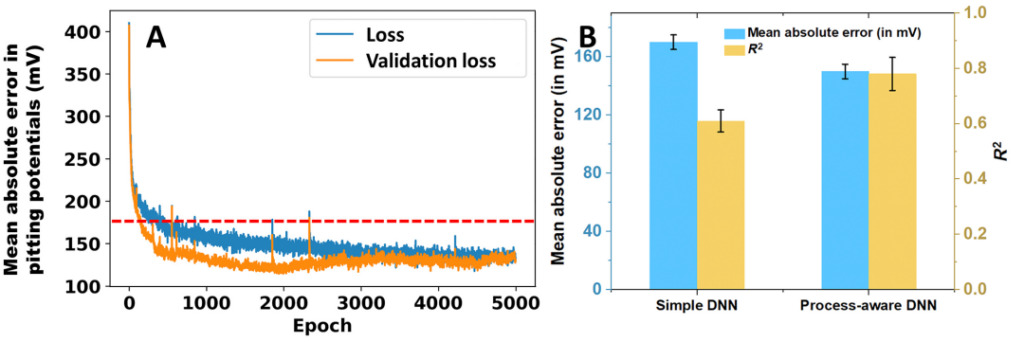
Figure 2: Process-aware DNN training results
A: The mean absolute error during training and validation, where the red line is the mean absolute error of the simple DNN model;
B: Comparison of results between process-aware DNN and simple DNN models.
Alloy composition optimization
In order to compare the differences between process-aware DNN and simple DNN in the alloy composition optimization process, the researchers started from similar alloy compositions, used the same learning rate, and optimized the alloy compositions using both models.
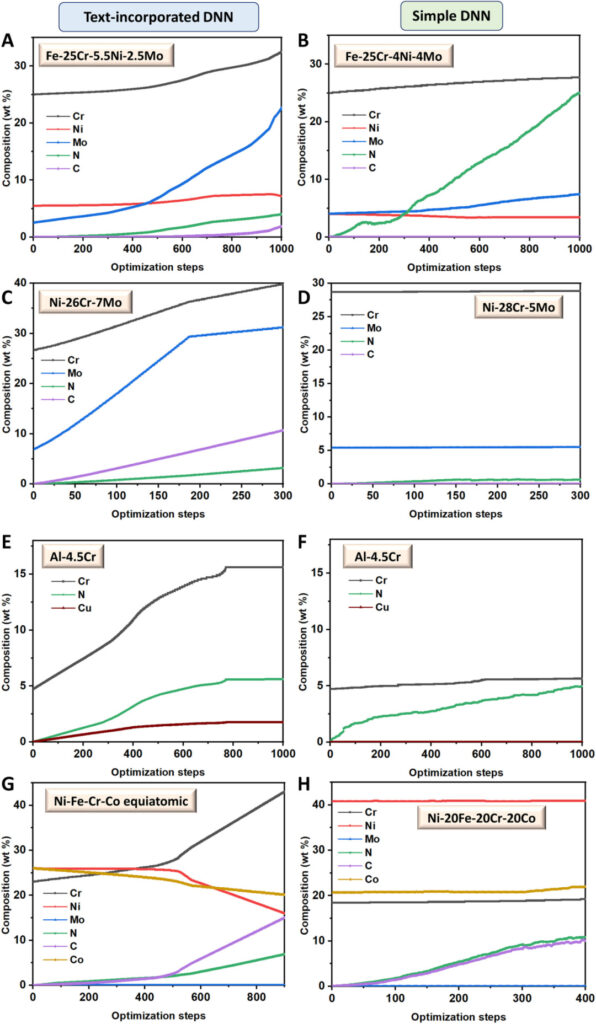
Figure 3: Component optimization results
A&B: Optimization results of iron-based alloys;
C&D: Ni-Cr-Mo alloy optimization results;
E&F: Al-Cr alloy optimization results;
G&H: Optimization results for high-moisture alloy.
As can be seen from the figure, the optimization results of the two models for iron-based alloys and FeCrNiCo high-moisture alloys are partially similar, but the optimization results for the other two alloys are very different.First, the process-aware DNN predicts that an increase in the Mo content will significantly increase the pitting potential of the Fe-based alloy and the Ni-Cr-Mo alloy. Second, the process-aware DNN believes that in the Ni-Cr-Mo alloy, interstitial nitrogen and interstitial carbon can increase the pitting potential of the alloy. Finally, in the Al-Cr alloy, the Cu element is also conducive to the improvement of the pitting potential. These are all ignored by the simple DNN.
Feature Transformation DNN
Model design
Through the alloy composition characterization function "WenAlloys", the alloy composition information can also be decomposed into a series of atomic, physical and chemical properties, and transformed into different descriptors as input values of the DNN model.
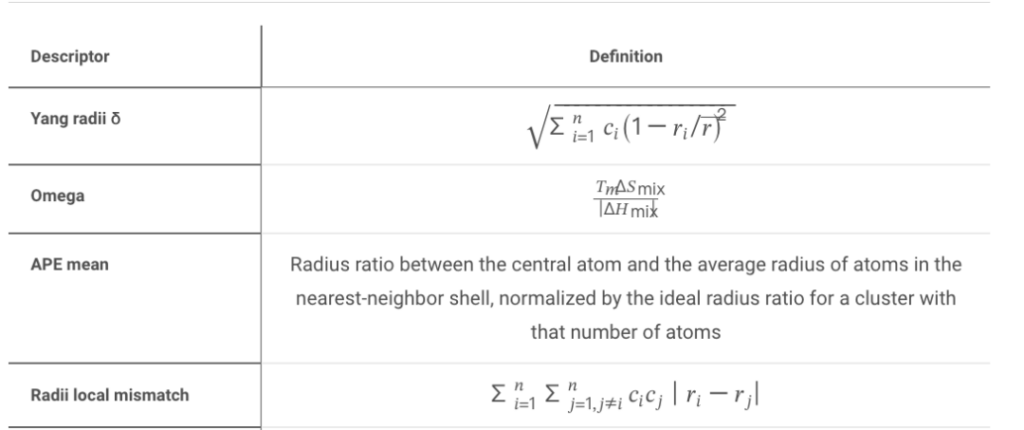
Table 2: Transformation results of some features
where ci , ri , Xi and Ec,i They represent atomic fraction, atomic radius, Pauli electronegativity, and element binding energy respectively.
Training and validation
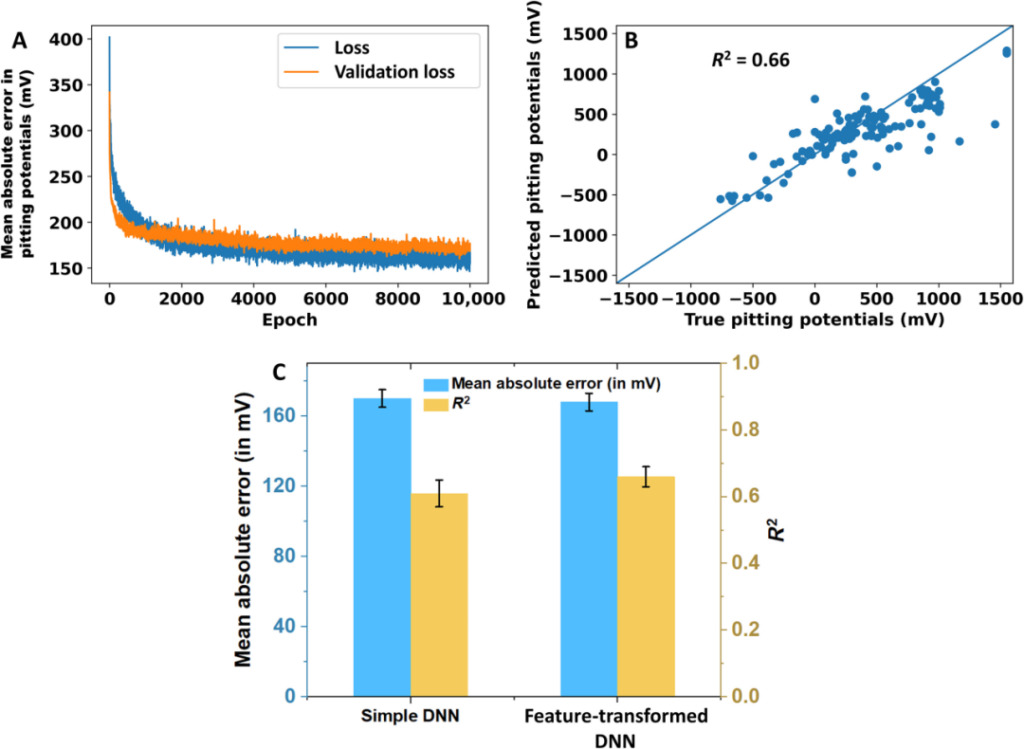
Figure 4: Training results of feature transformation DNN
A: Error curve during model training and verification;
B: Regression curve of predicted pitting potential and actual pitting potential after training;
C: Comparison of results between feature transformation DNN and simple DNN.
After training, the mean absolute error of the feature transformation DNN is about 168 mV, R2 It is 0.66, and the performance is slightly improved compared with the simple DNN model.
Analysis of feature transformation DNN on anti-corrosion mechanism
One of the five types of alloys was selected for feature transformation and then input into the model for optimization. Based on the optimization curve, the input features can be divided into two categories.One type of feature curve changes significantly during the optimization process, beyond the expectations in the training set; the other type of feature has only slight changes during the optimization process.
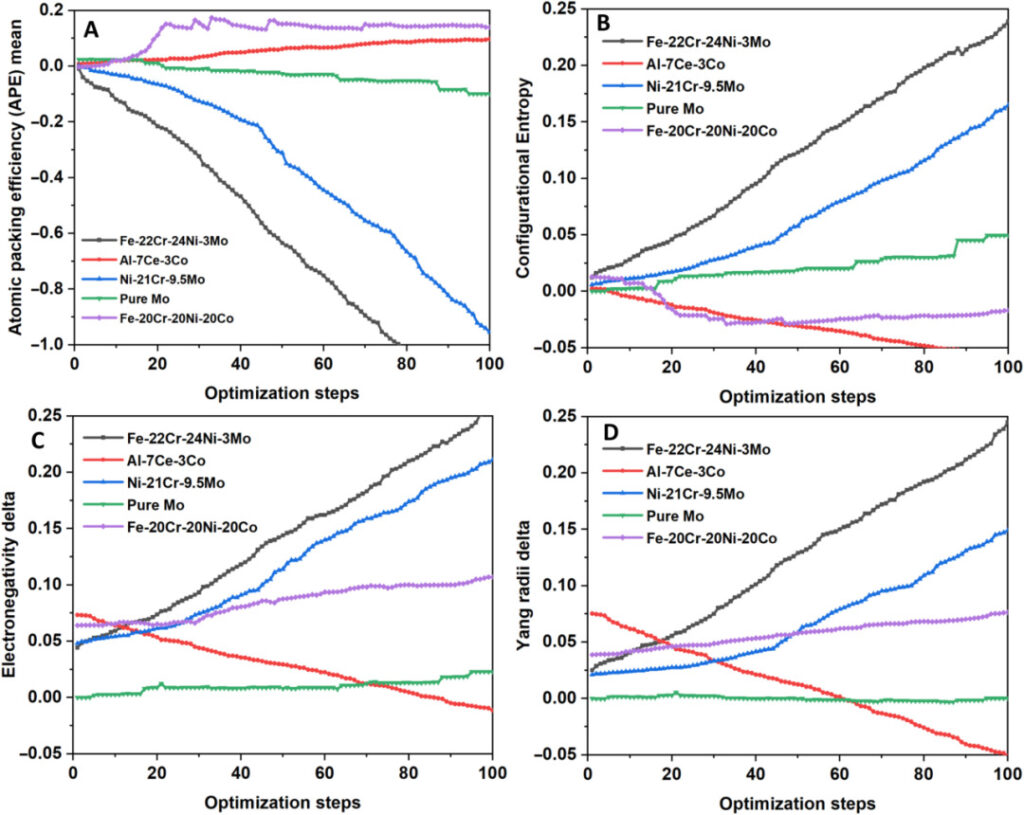
Figure 5: Optimization curves for different input features
The figure shows four features that changed significantly during the optimization process, which means that these features may be important parameters for improving the pitting potential of the alloy.
Prediction of Al-Cu-Sc-Zr alloy using feature transformation DNN
Since the input of feature transformation DNN only contains atomic, physical and chemical features of components,Therefore it can make predictions for elements that are not present in the training set.
In various alloys, Sc and Zr elements have shown improved corrosion resistance. Therefore, the research team used feature transformation DNN to analyze the effects of these two elements on Al-Cu alloys.
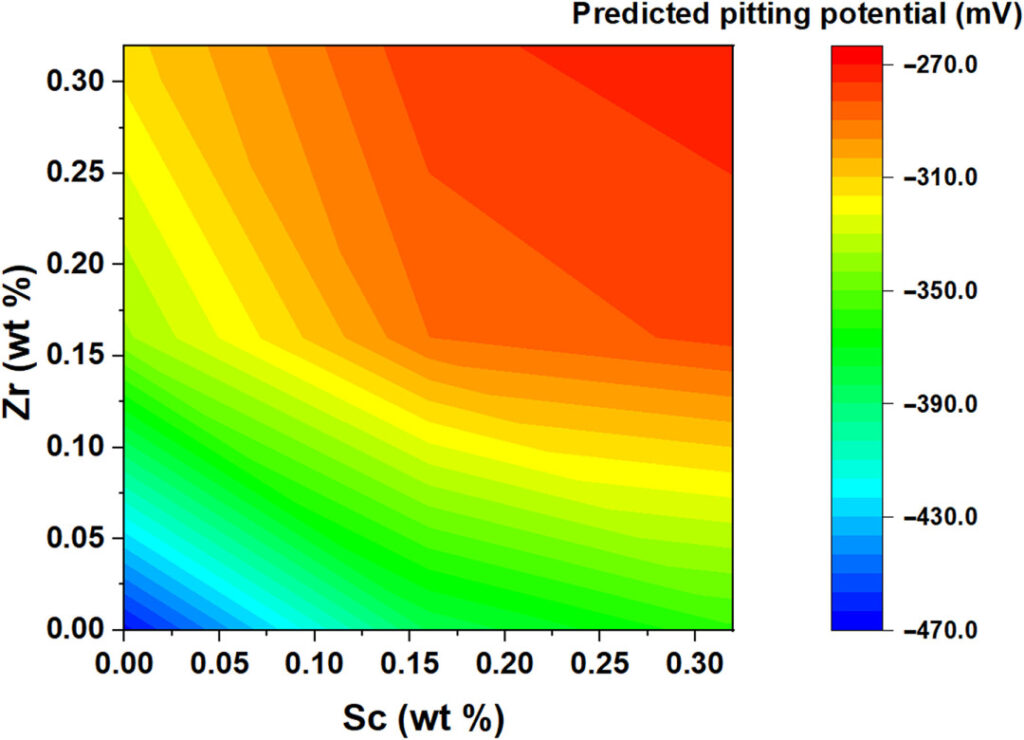
Figure 6: Feature transformation DNN prediction results for pitting potential of Al-Cu-Sc-Zr alloy
As shown in the figure, with the increase of Zr and Sc element content, the pitting potential of the alloy continues to increase, indicating that the corrosion resistance of the alloy has been improved.This result verifies the predictive power of feature transformation DNN for new elements.
The above results show thatCombining NLP with DNN, the model is able to read text data about alloy processing and testing methods, so it performs better than the traditional DNN model and can discover the influence of elements ignored by simple DNN on the corrosion resistance of alloys.The feature transformation DNN can predict the performance of elements that do not exist in the training set based on the atomic, physical and chemical properties of the alloy.
Corrosion: The Silent Killer of Metals
In 2009, the World Corrosion Organization (WCO) established April 24th of each year as World Corrosion Day to enhance public awareness of corrosion. As a common chemical phenomenon, corrosion exists in every corner of our lives. Whether it is various kitchen utensils, various household appliances, transportation across land, sea and air, or even various buildings with unique designs, they are all plagued by corrosion.It can be said that wherever there is metal, there is corrosion.
Metal corrosion includes chemical corrosion and electrochemical corrosion, among which electrochemical corrosion is more common and more harmful. Electrochemical corrosion refers to the phenomenon that two metals form a circuit in an electrolyte solution, forming a galvanic cell, which causes the active metal to corrode. Common electrochemical corrosion includes uniform corrosion, pitting corrosion, stress corrosion, crevice corrosion, etc.Among them, non-uniform corrosion, especially pitting corrosion and other forms of corrosion that are not easy to detect, are more harmful to metals and can easily cause accidents.

Figure 7: Common types of electrochemical corrosion
On November 22, 2013, the oil pipeline in Qingdao, Shandong Province, was exposed to high chlorine and alternating dry and wet environments for a long time, which led to corrosion and thinning of the pipe wall, and eventually ruptured, causing crude oil leakage. During the subsequent cleanup and repair process, due to improper on-site operations, the crude oil exploded, eventually causing 62 deaths and 163 injuries.
Corrosion is often difficult to detect, so avoiding corrosion accidents requires regular manual inspections and repairs, which consumes a lot of manpower and material resources.Now, with the help of AI, we can optimize the composition of alloys and find materials with better corrosion resistance.At the same time, digital corrosion monitoring systems are also being put into use to help us quickly locate corrosion potentials, so that the "silent killer" is no longer silent.
Reference Links:
[1] http://impact.nace.org/documents/Nace-International-Report.pdf
[2] https://whatispiping.com/corrosion/?expand_article=1
[3] https://www.gov.cn/govweb/jrzg/2014-01/11/content_2564654.htm#:
This article was first published on HyperAI WeChat public platform~