Tomatoes Also "scream" Under Pressure. Tel Aviv University Found That the Plant Kingdom Is Not Silent
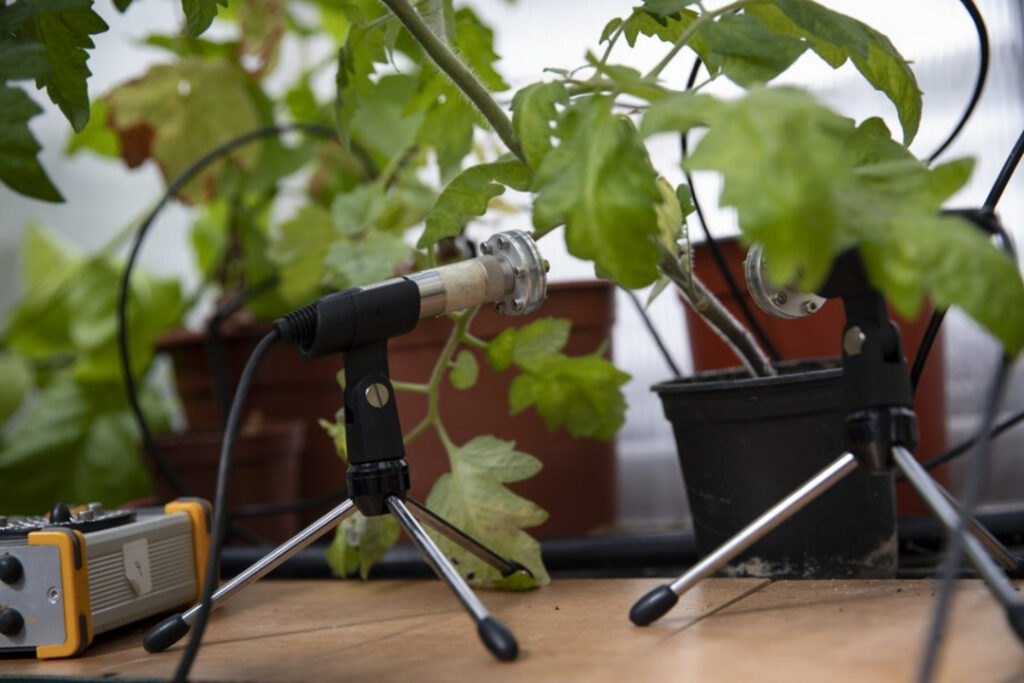
Contents at a glance:In the past, most of the research on plants' ability to sense and adapt to the outside world has been focused on the release of organic compounds and the transmission of photoelectric signals. A recent study has shown that plants can also transmit information through sound waves. Recently, researchers at Tel Aviv University in Israel have used machine learning to confirm that plants will emit high-frequency "screams" when faced with water shortages and survival pressures. This result has been published in the journal Cell.
Keywords:Agronomy Support Vector Machine Convolutional Neural Network
This article was first published on HyperAI WeChat public platform~
Adverse natural environments, including geographical location, climate conditions, and human activities, can cause harm to plants. These hazards are called adversity or stress. For example, when plants are dry and lack water, bubbles will form in the wood that is responsible for transporting water.When bubbles continue to expand and even burst, cavitation will occur.This phenomenon redistributes the stress (cyclic stress) in the plant body. When the stress is concentrated in one part, it is possible to quickly release a large amount of energy - mechanical energy is converted into sound energy. This process is called acoustic emission (AE).
However, when using acoustic emission to detect plants, it is usually necessary to connect the sensor directly to the plant being tested. Therefore, although scientists in the past were able to detect the sounds of plants, the sound waves were transmitted by the plants themselves and then received by the equipment.There is no proof that outsiders can hear it.
In the latest study, researchers from Tel-Aviv University in Israel combined laboratory observations with machine learning to prove that the ultrasonic waves emitted by tomato and tobacco plants under stress can propagate in the air, and successfully distinguished the different sounds emitted by different plants under two stressful environments by training machine learning models.The research results have been published in the journal Cell, titled "Sounds emitted by plants under stress are airborne and informative."
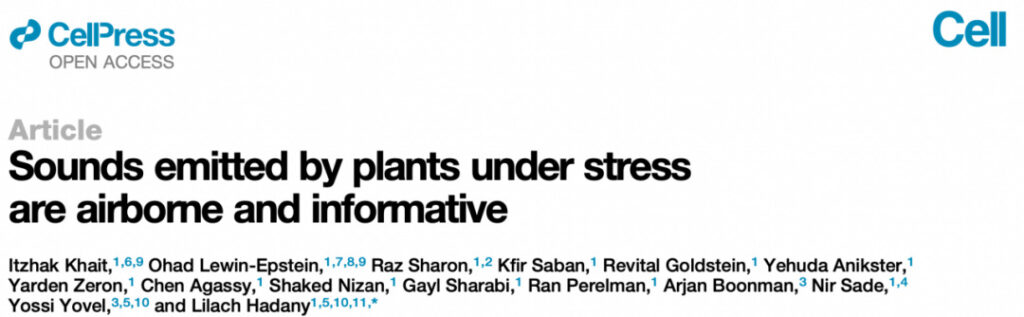
The research results have been published in Cell
Paper address:
https://doi.org/10.1016/j.cell.2023.03.009
Experiment Overview
When the author of this paper was interviewed, he introduced the experimental process and results.
The experimental subjects were tomatoes and tobacco, which were divided into three groups: drought, stem cut, and control. The control group was further divided into three conditions: normal growth, only soil in the pot without plants, and before and after control of each plant. The whole experiment was divided into three stages:
Phase I,The experimental subjects were placed in a soundproof box, and two special microphones that could receive ultrasonic waves in the range of 20 to 100 kHz were placed 10 cm away from the experimental subjects to collect the sounds of the plants under different conditions.
Phase IIThe soundproof box was placed in a noisy greenhouse environment, and the trained model was used to identify the noise and the sounds of plants in different environments.
The third stage,The relationship between plant sound production and drought severity was explored, and the sound status of other types of plants was also recorded.

Figure 1: Soundproof box for recording plant “screams”
The results showed that the plants emitted sounds with a frequency of 40 kHz to 80 kHz (the highest frequency that an adult can hear is about 16 kHz). When the plants were normal and not under any stress, they emitted no more than one sound per hour;The sound is emitted about 30-50 times per hour and can be detected even 3-5 meters away, comparable to a loud human "scream".
Plant sound dataset under stressful conditions
Publishing Agency:Tel Aviv University, Israel
Data Type:WAV format
Estimated size:10M
Release time:2023
Download address:hyper.ai/datasets/23544
Model training and results
In the first phase of the experiment, the researchers collected 5,483 audio clips recorded during the experiment into a small plant sound dataset. They developed a machine learning model specifically for this purpose.according toThe sounds that plants make identify different conditions (drought and being cut).
First, the researchers trained a Support Vector Machine (SVM) model. They divided the sounds into four groups, each containing two plant types (tomato and tobacco) and two treatments (drought or shear) for training the model. For cross-validation, the model was tested only on plants that were not involved in the training process.Three feature extraction methods were used, respectively are basic feature extraction (Basic), Mel-frequency cepstral coefficient feature extraction (MFCC) and scattering network (Scattering network).
The experimental results are shown in the figure. The SVM based on the scattering network can successfully identify drought and cut plants.And the accuracy is around 70%.
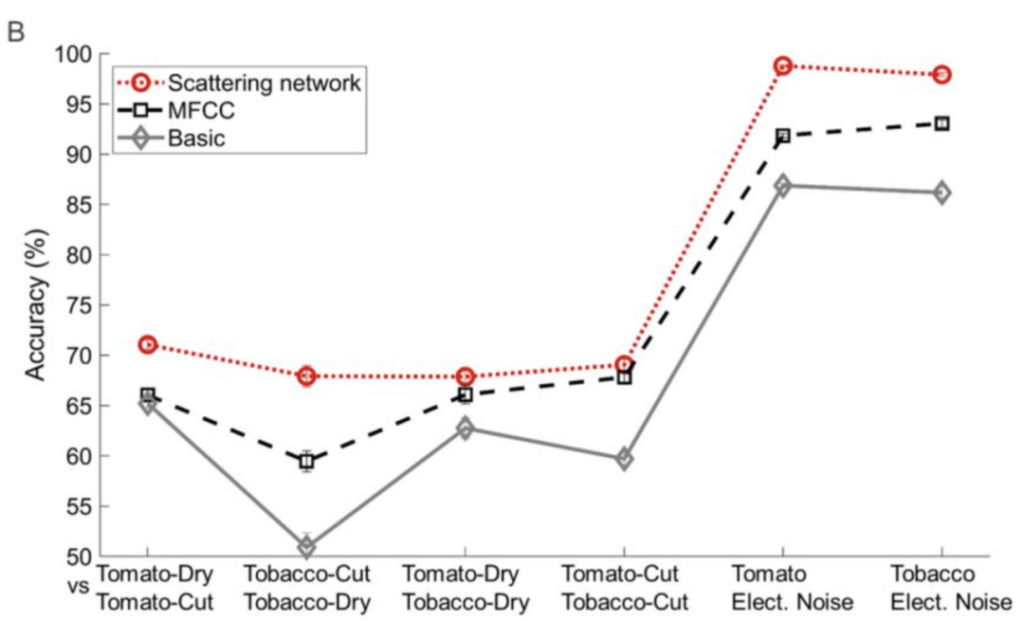
Figure 2: Accuracy of SVM classifiers based on three different feature extraction methods
In the second phase of the experiment, the researchers first collected a sample of greenhouse sounds and then trained a convolutional neural network model toDistinguish between natural noises (wind, rain, construction, etc.) and the sounds of drought-prone tomatoes.The experimental process and results are shown in Figure 3.The model's recognition and resolution accuracy is as high as 99.7%.

Figure 3: Distinguishing greenhouse noise from tomato sounds
(a) The researchers recorded the noise of an empty greenhouse without plants for several days to train the machine model.
(b) Record the sounds made by tomatoes in a greenhouse and filter them using a machine model, leaving only the sounds made by tomatoes.
(c) The confusion matrix shows that the model can successfully distinguish between tomato sounds and greenhouse noise with an accuracy of about 99.7%.
(d) The confusion matrix shows that the model can distinguish drought/non-drought tomatoes with an accuracy of about 84%.
In the validation phase, the researchers used Leave-One-Person-Out cross validation (LOPO-CV) to perform a robust evaluation of the trained model. AConfusion Matrix, from which the value of the equilibrium accuracy is obtained.
Convolutional neural network model code:
https://doi.org/10.5281/zenodo.7612742
In the third phase of the experiment, the researchers placed the watered tomatoes in a greenhouse for 10 days without watering them again.They used the above CNN model to filter out greenhouse noise.The sound of each tomato plant was counted every day. The result is shown in Figure 4. The plant made almost no sound right after watering.The screams are the loudest at 4-6 days.Then, as the drought worsened, the screams decreased.

Figure 4: Recording of tomato plants screaming due to drought in the greenhouse
However, even though the experiment revealed that certain plants can scream loudly under stress, the researchers alsoSeveral directions for further exploration in the future are proposed:
* Plant sound mechanism:The team predicts that the underlying mechanism of plant sound production may be a phenomenon called "cavitation" in the rhizomes.
* Increase research on environmental conditions, stress conditions and plant species:In the future, plant sounds could be analyzed in environments such as fields with more background noise; plant species from different families could be tested; and other stress environments could be studied, including different pathogens, cold, herbivore attack, UV radiation, and different life stages of plant species.
* Adaptive responses of plants to sound:In the future, it will be possible to explore whether other organisms can already classify and respond to these sounds.
AI is down to earth: exploring plant sounds to boost agricultural development
Regarding the research results, Lilach Hadany, an evolutionary biologist at Tel Aviv University and one of the authors of this article, said in an interview: "This research will not replace visual monitoring of plants, and the combination of the two detection methods is expected to be more conducive to studying plants. At the same time, we have applied for a patent for using acoustic information to adjust the direction of plant irrigation. The sounds made by plants can be heard by some animals, such as moths, bats and mice. But we still need to understand what happens in nature.What plants and animals respond to these sounds?We are currently researching this and hope to have an answer within a few years.”
The author further said that this research may be helpful to agriculture. According to the United Nations' forecast data on population and hunger,By 2050, the global population will have increased by a further 2 billion people, and agricultural productivity will need to increase by 60% to provide adequate food.As agriculture faces multiple challenges such as global warming, population growth, and food security, scientists have gradually turned their attention to AI and realized that it is a useful tool for improving agricultural efficiency and sustainable development.
Combined with past research, AI can be used to manage pests and diseases, optimize crop growth, accurately apply fertilizers and irrigation, etc., which can not only increase agricultural output and quality, but also protect the environment and reduce water waste and chemical pollution.
Reference Links:
[1]https://www.cas.cn/kj/202303/t20230331_4882525.shtml
[2]https://finance.sina.cn/tech/2022-02-24/detail-imcwipih5062199.d.html?fromtech=1
[3]https://www.businessinsider.com/plants-shriek-with-high-pitched-ultrasonic-clicks-when-stressed-study-2023-3
This article was first published on HyperAI WeChat public platform~