AI Fights Superbugs: McMaster University Uses Deep Learning to Discover a New Antibiotic Abaucin
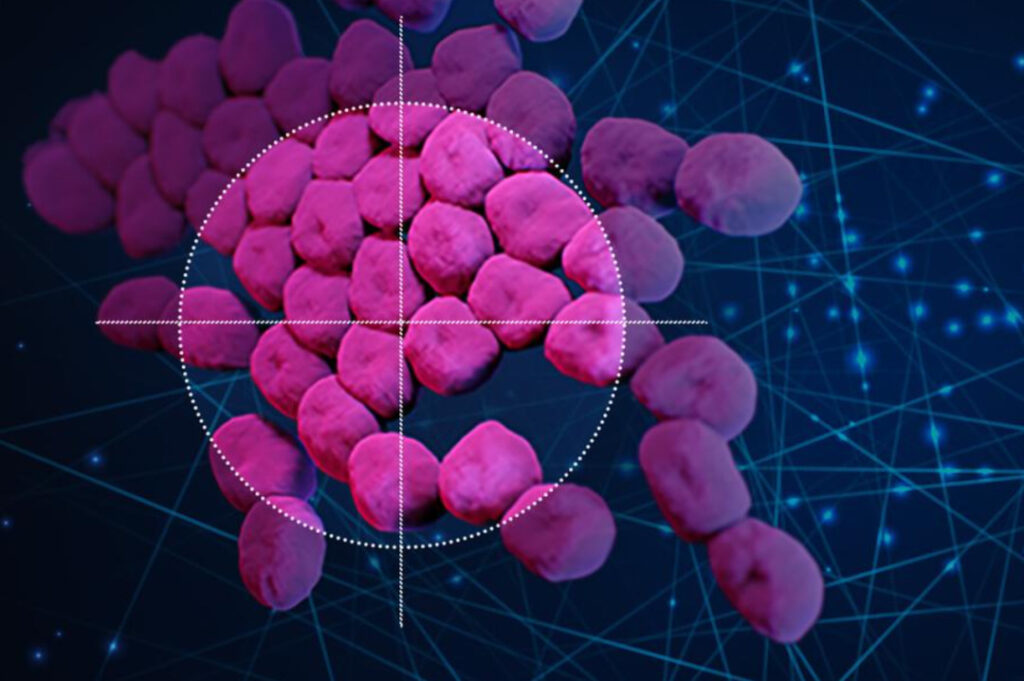
Contents at a glance:Acinetobacter baumannii is a common hospital-acquired Gram-negative pathogen that often exhibits multidrug resistance. Using traditional methods, it is difficult to discover new antibiotics that inhibit this bacterium. However, machine learning can quickly explore chemical space, thereby increasing the possibility of discovering new antibacterial molecules. Recently, a research result was published in the international journal Nature Chemical Biology. Researchers found that abaucin can effectively inhibit Acinetobacter baumannii.
Keywords:Acinetobacter baumannii abaucin deep learning
This article was first published on HyperAI WeChat public platform~
Acinetobacter baumannii (scientific name: Acinetobacter baumannii, commonly known as: AB bacteria) is the most common Gram-negative bacillus in the genus Acinetobacter. It is widely found in water and soil in nature, and also in normal human skin, respiratory tract, digestive tract and urogenital tract. This bacterium is common in hospital infections and is also a pathogen in aquaculture animals.Commonly causes bacteremia, pneumonia, meningitis, peritonitis, endocarditis, and urinary tract and skin infections.
Acinetobacter baumannii has strong resistance to heat, humidity, ultraviolet rays, chemical disinfectants, and low temperature. It can survive on dry surfaces for more than 25 days. Conventional disinfectants can only inhibit its growth but cannot kill it. It is also resistant to soap and is the most commonly isolated Gram-negative bacillus on the hands of medical staff, medical equipment, and surfaces.Due to the abuse of antibiotics, Acinetobacter baumannii has developed multiple drug resistance and is identified by the World Health Organization as one of the most dangerous antibiotic-resistant bacteria in the world.
Recently, researchers including Gary Liu from McMaster University and Denise B. Catacutan from the Massachusetts Institute of Technology used deep learning to screen approximately 7,500 molecules to identify new antibiotics that inhibit Acinetobacter baumannii.Currently, the research has been published in the journal Nature Chemical Biology with the title "Deep learning-guided discovery of an antibiotic targeting Acinetobacter baumannii".

The research results have been published in the journal Nature Chemical Biology
ArgumentAddress:
https://www.nature.com/articles/s41589-023-01349-8#access-options
Experimental procedures
Dataset
The researchers screened 7,684 small molecules based on the growth of Acinetobacter baumannii ATCC 17978 in LB medium.These small molecules included 2,341 generic drugs and 5,343 synthetic chemicals, all of which were provided by the Broad Institute's high-throughput screening sub-library. Based on the traditional hit rate cutoff (i.e., molecules with a growth inhibition rate less than one standard deviation compared to the average growth inhibition rate of the entire data set), they determined that 480 molecules were "active" and 7,204 molecules were "inactive."
Model Training
To enhance the model structure, the researchers connected the molecular representation with 200 additional molecular-level features calculated using RDCit. In addition, to further improve the performance of the model, the researchers used an ensemble of 10 models to build and train the model on a training dataset of 7,684 molecules (with ~6.2% of valid examples) and applied the model to the updated Drug Repurposing Hub.The researchers randomly divided the dataset into 80% of training data, 10% of validation data, and 10% of test data.
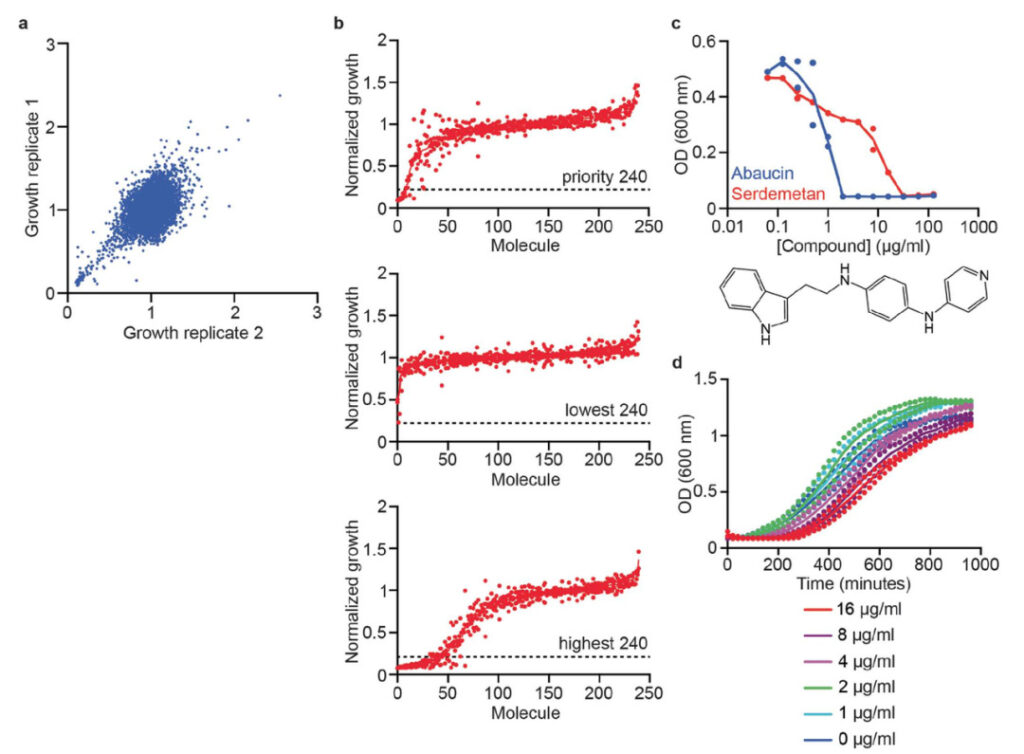
Figure 1: Model training data
a. Initial screening data for 7,684 small molecules that inhibit the growth of Acinetobacter baumannii ATCC 17978 in LB medium at 50 μM.
b. Ranked growth inhibition data of 240 priority molecules selected for validation from the prediction set (top); Ranked growth inhibition data of 240 predicted molecules with the lowest prediction scores (middle); Ranked growth inhibition data of 240 predicted molecules with the highest prediction scores that were not found in the training dataset (bottom). The horizontal dashed line represents the stringent hit cutoff of >80% growth inhibition at 50 μM.
c. The growth of Acinetobacter baumannii in LB medium was inhibited by abaucin (blue) and serdemetan (red).
d. Growth kinetics of A. baumannii cells after treatment with different concentrations of abaucin for 6 h.
Code acquisition:
https://github.com/chemprop/chemprop
Mouse model experiments
The researchers pretreated 6- to 8-week-old female C57BL/6N mice with 150 mg/kg and 100 mg/kg of cyclophosphamide, respectively, four days and one day before the start of the experiment to reduce neutropenia. On day 0, the mice were anesthetized with isoflurane and 0.1 mg/kg of buprenorphine was injected intraperitoneally as an analgesic. The researchers used approximately 30-35 pieces of high-pressure tape to create a 2 cm2 abrasion on the back of the mice, which was peeled off to the basal layer of the epidermis and then infected with ~6.5 x 106 CFU A. baumannii ATCC 17978.Mice (n = 5/6) were treated with ~10-20 μl of Glaxal Base with abaucin (experimental group) or DMSO (control group) at 1, 2, 3, 4, 6, 10, 21, and 24 h after infection.
Experimental Results
Researchers discovered an antibacterial compound called abaucin with the help of machine learning.It has a narrow spectrum of activity against Acinetobacter baumannii and can interfere with the transport of lipoproteins by interfering with the mechanism of LolE. The figure below shows the process of deep learning-guided discovery of abaucin.
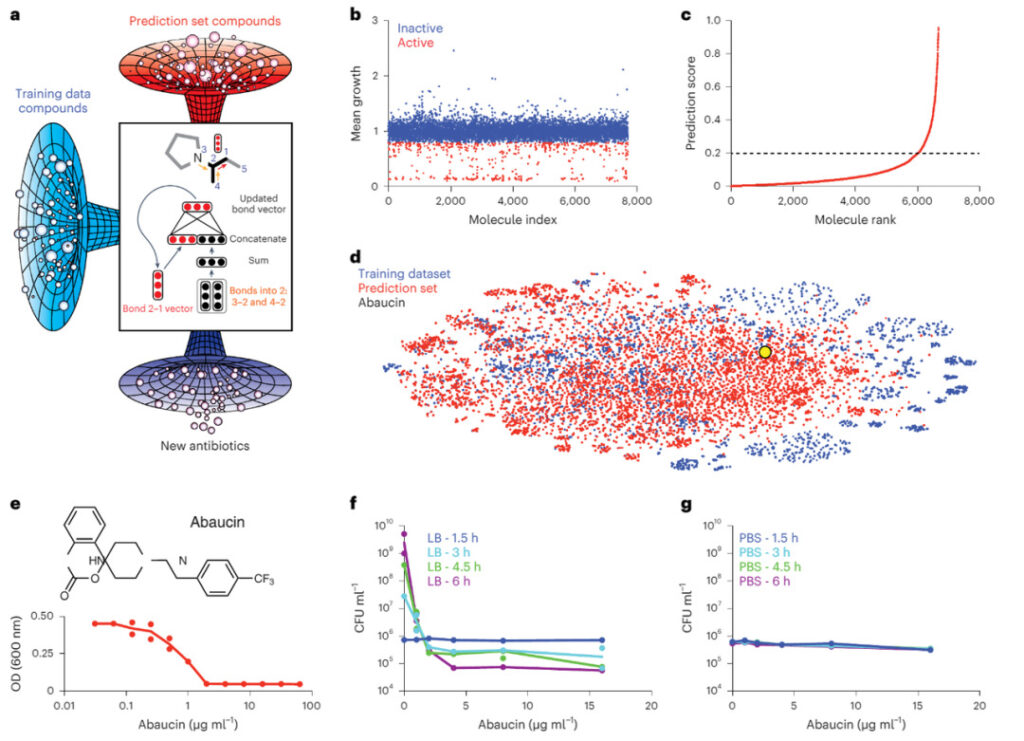
Figure 2: Machine learning guided the discovery of abaucin
a. ~7,500 molecules screened (blue); a deep neural network for directed information transfer trained with this growth inhibition dataset and predicted in the Drug Repurposing Hub (red); new molecules that can inhibit Acinetobacter baumannii (purple).
b. Growth inhibition of Acinetobacter baumannii ATCC 17978 at 50 μM by a collection of 7,684 small molecules.
c. The trained model ranks the prediction scores of the molecules in the drug repurposing center. Molecules with prediction scores greater than 0.2 are preliminary candidate molecules.
d. A t-SNE plot shows the chemical relationships between the training dataset (blue), prediction set (red), and abaucin (yellow).
e. Growth inhibition of Acinetobacter baumannii by abaucin in LB medium.
f. The killing effect of abaucin on Acinetobacter baumannii at different concentrations after 1.5 h (blue), 3 h (cyan), 4.5 h (green), and 6 h (purple) of incubation under nutrient-rich conditions.
g. The killing effect of abaucin on Acinetobacter baumannii after 1.5 h (blue), 3 h (cyan), 4.5 h (green), and 6 h (purple) of incubation under nutrient-deficient conditions.
As shown in Figure 2, the researchers screened approximately 7,500 molecules, trained a neural network using this growth inhibition dataset, and predicted novel molecules that could inhibit the activity of Acinetobacter baumannii.Collectively, these data suggest that abaucin exerts its antimicrobial potency by inhibiting a biological process that is most active during growth and division, consistent with most known antibiotics.
In addition, to test the in vivo efficacy of abaucin,The researchers established wound infections on the backs of mice.
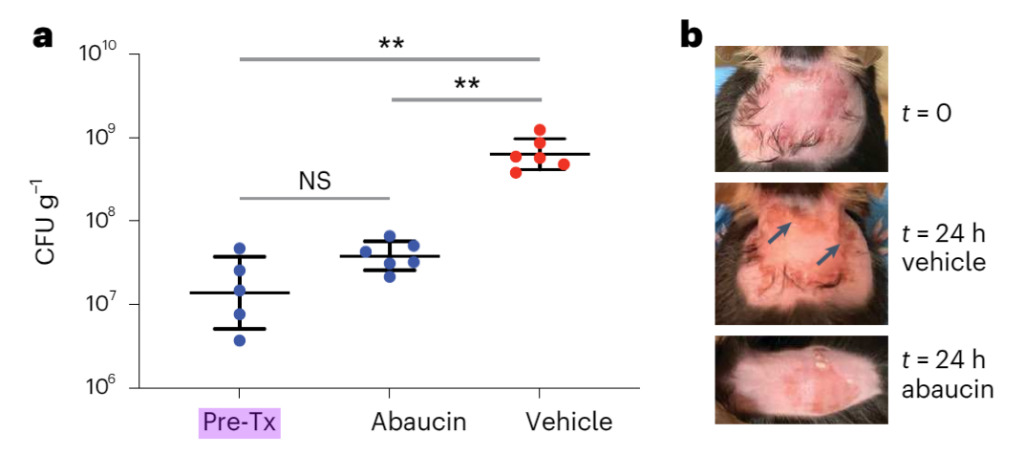
Figure 3: Abaucin can inhibit Acinetobacter baumannii infection in the wound model
a. In the dorsal wound infection model, mice were infected with Acinetobacter baumannii ATCC 17978 (~6.5 x 106 CFU). One hour after infection, mice were treated with DMSO (red; n = 6) or 4% abaucin (blue; n = 6) for more than 24 hours. The vertical axis is the bacterial burden of wound tissue 25 hours after infection. Pre-Tx represents the bacterial burden at the time of initial treatment (n = 5). Black lines represent mean ± sd. NS indicates not statistically significant; ** P < 0.005 using unpaired two-sided t-test with Welch correction (Pre-Tx vs. 4% abaucin, P = 0.0704; Pre-Tx vs. DMSO, P = 0.0034; DMSO vs. 4% abaucin, P = 0.0039).
ps Generally, one asterisk means the p value is less than 0.05, two asterisks mean the p value is less than 0.01, and three asterisks mean the p value is less than 0.001. The smaller the p value, the stronger the significance.
b. Images of the dorsal surface of mice before infection (t = 0), after 24 hours of DMSO treatment, and after 24 hours of abaucin treatment. The inflammation observed in DMSO (arrows) was absent in mice treated with abaucin.
Figure 3 shows that the bacterial load in wounds treated with abaucin was significantly lower than that in the DMSO group, and the wound inflammation that was still present after 24 hours of DMSO treatment disappeared in abaucin.Abaucin can effectively control infections caused by Acinetobacter baumannii.
In summary, researchers used deep learning to find that abaucin can effectively control Acinetobacter baumannii infection.This study highlights the role of machine learning in discovering new antibiotics and guiding the study of challenging Gram-negative pathogens.
AI changes the traditional way of discovering new drugs
It is worth noting that this research was conducted by Jon Stokes, an assistant professor at McMaster University, James J. Collins, a professor of medical engineering and science at MIT, and McMaster University graduate students Gary Liu and Denise Catacutan. Jon Stokes, Gary Liu and Denise Catacutan are all members of the Stokes Laboratory.
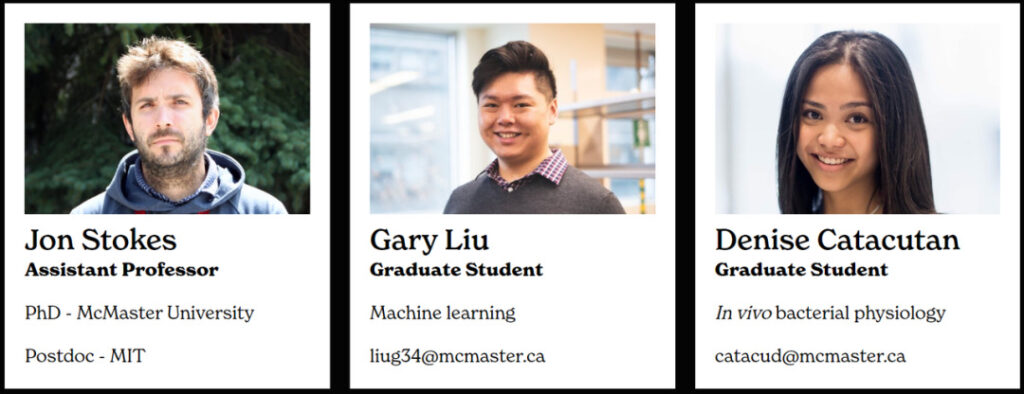
Stokes Laboratory Address:
https://www.thestokeslab.com/projects
The laboratory discovers novel antibiotics by utilizing state-of-the-art high-throughput biology and contemporary artificial intelligence approaches.Specifically, the researchers trained deep learning models to predict new antibiotics from a large computer simulation database and also built models to predict the mechanism of action and in vivo properties of new antibiotics. They used machine learning to accelerate the discovery of new antibiotics while reducing research and development costs.
Professor Stokes once said in an interview that "artificial intelligence will fundamentally change the way new drugs are discovered."The results of the study show that artificial intelligence has great significance for discovering new antibiotics against a variety of challenging pathogens, and this method also has potential in finding other antimicrobial therapies. Although artificial intelligence cannot solve all problems, as a powerful tool, it can help us find new drugs and bring hope to people.
Reference articles:
[1]http://ccm.dxy.cn/article/805579
[2]https://zh.wikipedia.org/wiki/%E9%AE%91%E6%B0%8F%E4%B8%8D%E5%8B%95%E6%A1%BF%E8%8F%8C
[3]https://www.ctvnews.ca/health/scientists-use-ai-to-discover-antibiotic-for-very-difficult-to-treat-bacteria-1.6411927
-- over--