Peking University Develops a Pluripotent Stem Cell Differentiation System Based on Machine Learning to Efficiently and Stably Prepare Functional Cells
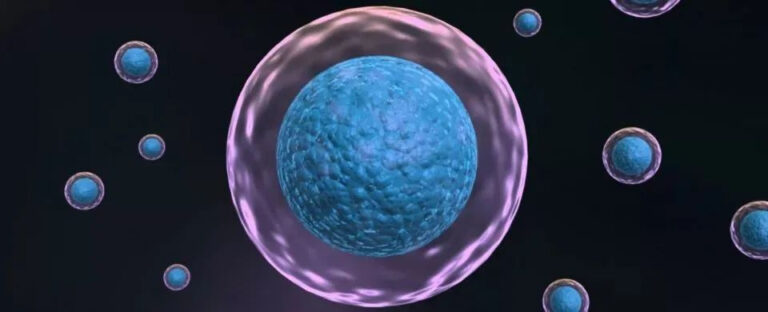
Contents at a glance:Since the 20th century, stem cell and regenerative medicine technology has been one of the hot frontiers in the international biomedical field. Today, researchers have begun to explore the transformation of stem cells into specific types of cells. However, in this process, stem cells will grow irregularly or spontaneously differentiate into different types of cells. Therefore, how to control the growth and differentiation of stem cells has become one of the challenges faced by researchers. In this article, researchers such as Zhao Yang's research group at Peking University tried to apply machine learning to the differentiation process of pluripotent stem cells, and effectively improved this situation, while bringing new directions to regenerative medicine.
Keywords:Pluripotent stem cells Image analysis Machine learning
This article was first published on HyperAI WeChat public platform~
Pluripotent stem cells (PSCs) are a type of multipotent cells that have the ability to self-renew and self-replicate. They can proliferate and differentiate into various cell types indefinitely in vitro.Replacing damaged cells and promoting the recovery of damaged tissue function have brought new hope for the treatment of eye diseases, cardiovascular diseases, and nervous system diseases.
However, the current directed differentiation process of pluripotent stem cells will result in problems such as unstable differentiation between cell lines (line-to-line) and batches (batch-to-batch), which makes the preparation of functional cells time-consuming and laborious, seriously hindering the research and development and large-scale manufacturing of pluripotent stem cell clinical application products.Therefore, it is particularly important to achieve real-time monitoring of the differentiation process of pluripotent stem cells.
Recently, the research groups of Zhao Yang and Zhang Yu from Peking University, together with the research group of Liu Yiyan from Beijing Jiaotong University, developed a differentiation system based on bright-field dynamic imaging of living cells and machine learning. The system can intelligently regulate and optimize the differentiation process of pluripotent stem cells in real time, and achieve efficient and stable production of functional cells.Currently, the research results have been published in the journal Cell Discovery, titled "A live-cell image-based machine learning strategy for reducing variability in PSC differentiation systems."
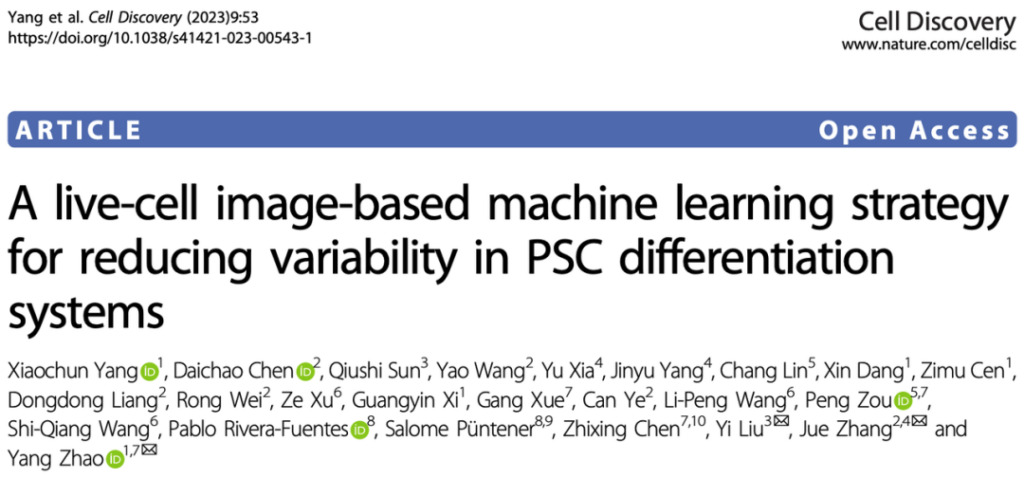
The research results have been published in the journal Cell Discovery
Paper address:
https://www.nature.com/articles/s41421-023-00543-1
Experiment Overview
Currently, microscopy technology can capture images of cells, and machine learning methods can analyze cell images.Therefore, this study used machine learning algorithms to identify and classify cells in bright-field images to determine their lineage or cellular composition, helping researchers better understand cell structure and function.
It has been proven that the research results can effectively optimize and improve the differentiation process of pluripotent stem cells into cardiomyocytes (CM) and liver and kidney chimeric cells.The whole research method and process are as follows:
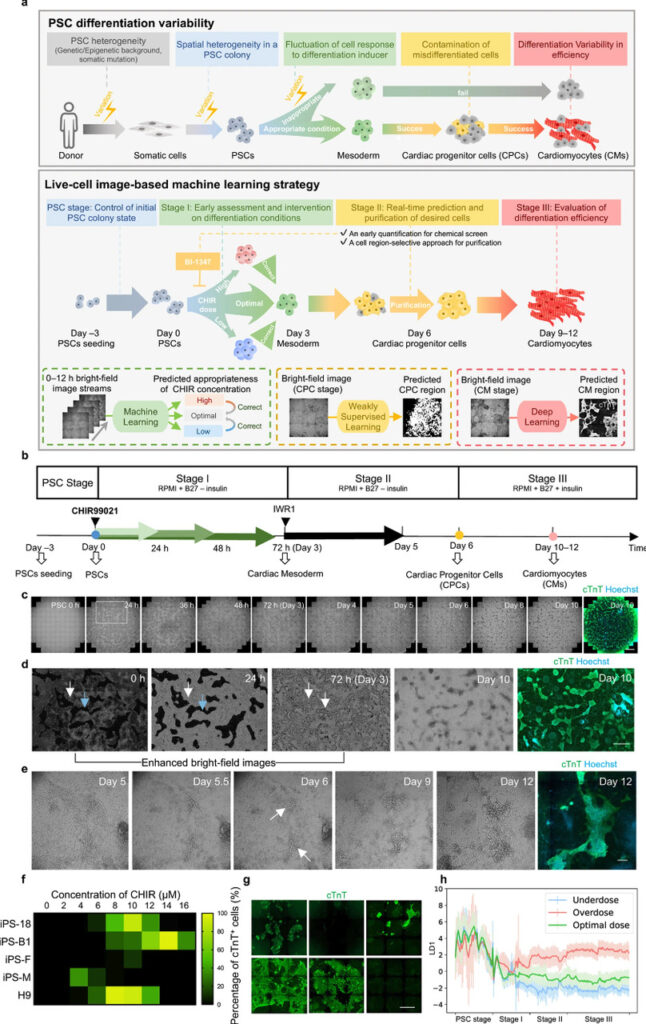
Figure 1: Machine learning optimized PSC-to-CM
a:The upper graph shows that there is variability in each PSC differentiation process, and the lower graph shows that machine learning applied to the above differentiation processes effectively reduces the variability.
b:PSC-to-CM differentiation process of canonical Wnt signaling pathway regulated by small molecule modulators. Green arrows indicate the duration and concentration of CHIR regulation in the first stage, and colored dots indicate machine learning checkpoints.
c:Delayed bright field images and cTnT fluorescence results over a 10-day period.
d:The location and morphology of cells that successfully and failed to differentiate during the entire process.
e:The texture and morphology of successfully differentiated cells change from day 5 to day 12.
f:Line-to-line variability in differentiation efficiency.
g:Differentiation variability among different batches of cells.
h:Changes in local features of differentiation images at different CHIR doses.
Experimental procedures
Experimental Dataset
The researchers used PSC-to-CM differentiation as the main example, and used the Zeiss Cell Discover 7 live cell fully automated imaging platform to capture bright field images during the differentiation process in real time and track the entire process, as shown in Figure 1b above. At the end of differentiation, successfully differentiated CMs were identified by fluorescent labeling with cTnT (a specific marker for cardiomyocytes).During this process, in order to increase the diversity of images, the researchers introduced several variables (different PSCs, initial cell density, differentiation medium, different CHIR doses), and ultimately collected more than 7.2 million images.
Experimental results
Combining live cell imaging technology and machine learning,This experiment achieved the following four results:
* Machine learning can accurately identify the state of differentiated cells and estimate differentiation efficiency.
The researchers found that on the sixth day of the differentiation process, the cells that eventually differentiated into CMs, namely CPCs (cardiac progenitor cells), began to show a spindle shape.Therefore, they used a weakly supervised model to identify such cells in bright field images and named it "Image-Recognized CPC (IR-CPC)".As shown in Figure 2 below, the researchers concluded that the correlation between the proportion of IR-CPC in total cells and the actual differentiation efficiency was 88%.

Figure 2: Correlation between IR-CPC ratio and true differentiation efficiency
At the same time, the researchers used the pix2pix deep learning model to predict the bright field images of the CM induction stage (i.e., the first stage of differentiation).As shown in the figure below, the correlation between the predicted differentiation efficiency and the actual differentiation efficiency is 93%.

Figure 3: Correlation between predicted and actual differentiation efficiency
The above experiments show that machine learning can identify the cell status at different stages of differentiation and make real-time predictions of differentiation results.
* Machine learning can predict differentiation time and induction factor concentration in real time.
During the differentiation process, the researchers found that at the mesoderm stage (0-3 days), the dose (concentration and treatment time) of the inducer CHIR99021 (CHIR) had a greater impact on the differentiation efficiency.They constructed a logistic regression model based on the CHIR-related features in the bright field images at the early stage of differentiation (0-12h) to predict the CHIR concentration in the wells (low, moderate, high).As shown in the figure below, when the CHIR treatment time is selected as 24h, the model's accuracy in judging the concentration of each well (a laboratory item with multiple small holes) reaches 93.1%.

Figure 4: Model prediction of CHIR concentration in the pore
At the same time, the researchers compared the prediction results (i.e., deviation scores) of the model under different CHIR treatment times (24h, 36h, or 48h) to obtain the optimal CHIR treatment time. As shown in Figure 5 below,The optimal CHIR treatment time was about 12 h (with the smallest deviation score). In addition, as shown in Figure 6, according to the model prediction results, the CHIR concentration can also be adjusted to improve the differentiation efficiency.

Figure 5: Model predicts optimal CHIR processing time

Figure 6: Differentiation results with and without CHIR concentration adjustment
The above experiments show that machine learning can achieve intervention in the dosage of inducers.
* Machine learning can determine the optimal state for PSC initiation differentiation in real time.
The researchers found that even at moderate CHIR concentrations, cells failed to differentiate, and they proposed that this was due to spatially variable differentiation.That is, cells at the edge of the PSC colony on day 0 of differentiation are more likely to succeed, while cells located in the center of the PSC colony are more likely to fail.
In response to this, the researchers established a machine learning model based on random forests to identify the image features of starting cells with a high differentiation success rate. The model results showed that cells with medium cell area, longer and more bumpy edges are more likely to differentiate successfully, which is consistent with actual observations.Based on this model, the researchers found that the correlation between the prediction of the starting PSC state and the actual differentiation efficiency was 76%, as shown in Figure 7 below.
Based on this, the researchers also artificially intervened and changed the initial morphology of cells.Effectively increased the differentiation efficiency from 21.6% ± 2.7% to 88.8% ± 10.5%.

Figure 7: Correlation between identification of cell starting state and prediction of differentiation efficiency
The above results show that machine learning can perform quality control on the initial state of PSC.
* Machine learning can help screen small molecule compounds and improve differentiation stability.
The researchers found that CHIR concentration is one of the important factors affecting differentiation, so they tried to conduct small molecule screening to use new compounds to offset inappropriate CHIR concentration. As shown in the figure below,Based on bright-field live cell images on day 6 of the differentiation process and the established weakly supervised model, the researchers built a small molecule screening platform and successfully screened out the compound BI-1347 from more than 3,000 small molecules.
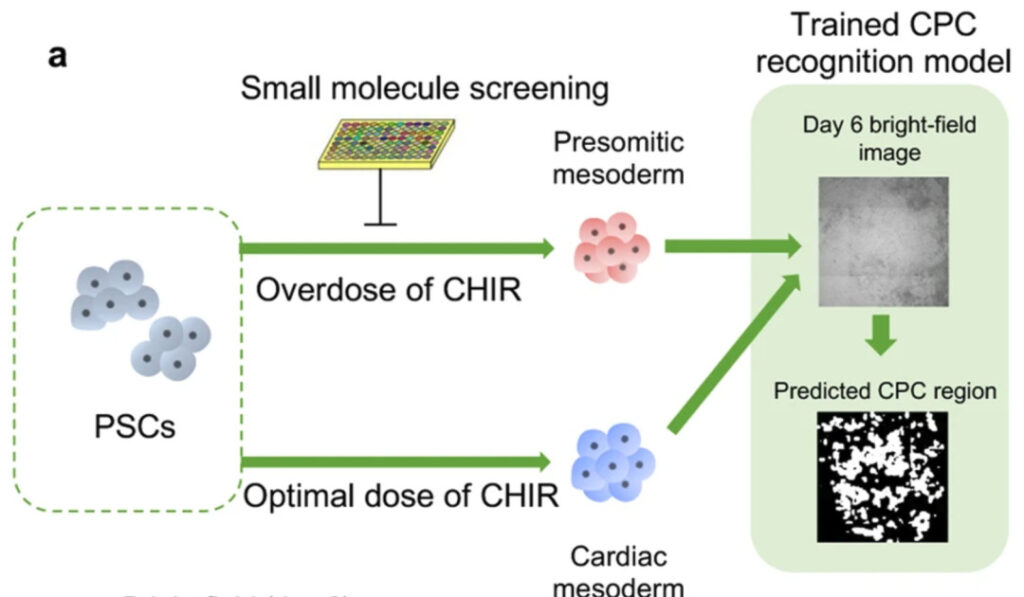
Figure 8: Machine learning screening of small molecule compounds
The above experiments show that based on the machine learning model, researchers can establish a small molecule screening platform to shorten the screening experiment cycle and reduce the screening cost.In addition, the small molecules screened by this technology have broadened the CHIR dosage range, thereby improving the overall stability of the PSC differentiation process.
Finally, in order to expand the application scenarios, the researchers applied the results of this study to the early stages of differentiation of renal progenitor cells and hepatocytes, and also achieved accurate prediction results.This research result can provide real-time guidance for the differentiation process of pluripotent stem cells.
Cell therapy: a new path for biomedicine
Cell therapy is an emerging therapy that has shown good therapeutic effects on many diseases (cancer, genetic diseases). Its main treatment methods are divided into immune cell therapy and stem cell therapy.Among them, stem cells have become one of the core research directions in this field due to their functions such as multidirectional differentiation, immune regulation and cytokine secretion.
At present, the development of cell therapy in my country is relatively short, but the future prospects are very broad.On the one hand, judging from the data, the next decade may be a period of rapid growth in this field. According to relevant data, the scale of my country's cell therapy market will increase from 1.3 billion yuan in 2021 to 58.4 billion yuan in 2030, with an average annual growth rate of 53%. Other data show that my country's cell and gene therapy market is expected to reach 2.59 billion US dollars in 2025, growing at a compound growth rate of 276%.
On the other hand, local governments have also continuously introduced relevant policies to support and encourage this field.For example, Beijing, Shanghai, Tianjin, Shenzhen and other places are vigorously developing the cell therapy industry. Shanghai has launched the "Shanghai Action Plan for Promoting Technological Innovation and Industrial Development of Cell Therapy (2022-2024)", proposing to strive to reach a scale of 10 billion yuan in Shanghai's cell therapy industry by 2024. Last year, Shenzhen successively issued documents to support the development of the biopharmaceutical industry, and will focus on supporting the high-quality development of industrial clusters including cell therapy drugs.
Dataset and code address:
https://GitHub.com/zhaoyanglab/ML-for-psc-differentiation
Reference Links:
[1]https://www.thepaper.cn/newsDetail_forward_23417694
[2]http://www.cls.edu.cn/Research/Research_Achievements6067.shtml
[3]https://stcsm.sh.gov.cn/zwgk/ghjh/20221104/f7b02ab5db40439e8d93f15b9dd206da.html
[4]http://legacy.frostchina.com/wp-content/uploads/2021/11/20211116-2.pdf
-- over--
This article was first published on HyperAI WeChat public platform~