Effectively Delaying Dementia: Yonsei University Found That the Gradient Boosting Machine Model Can Accurately Predict BPSD Subsyndrome
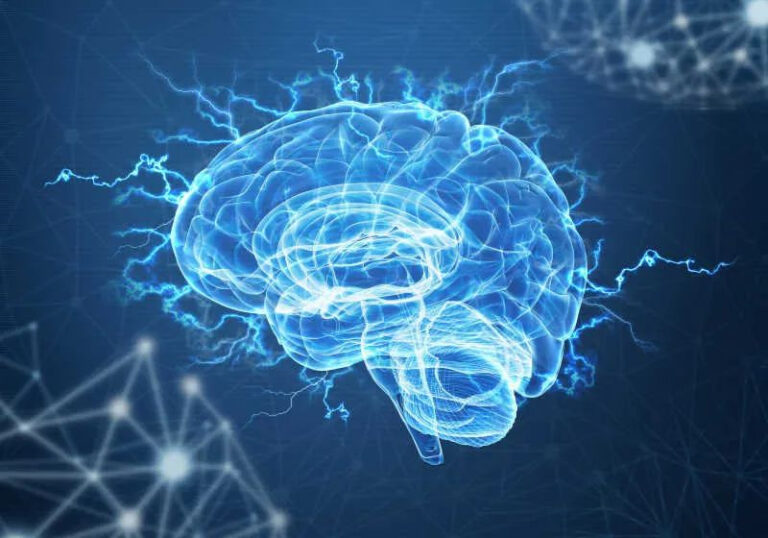
Contents at a glance:As the population ages, dementia has become a public health issue. Currently, the only way to treat the disease is through medication, and no effective cure has been found. Therefore, preventing dementia is particularly urgent. In this context, researchers at Yonsei University developed and validated several machine learning models for predicting BPSD. The experimental results show that machine learning can effectively predict BPSD subsyndromes.
Keywords:Dementia BPSD Gradient Boosting Machine
This article was first published on HyperAI WeChat public platform~
Currently, more than 550 million people worldwide suffer from dementia (Alzheimer's disease is the most common type), with nearly 10 million new cases each year.As the population ages, this number is expected to triple by 2050. Dementia is a brain disease that causes a slow decline in memory, thinking and reasoning. It primarily affects older people and is one of the leading causes of disability among them. It is the seventh leading cause of death worldwide (by total number of deaths), behind ischemic heart disease, stroke and chronic obstructive pulmonary disease.
Typically, patients with dementia display a range of behavioral and psychological symptoms (BPSD) such as agitation, aggression, apathy, and depression in addition to cognitive impairment.These symptoms are the most complex and challenging issues in dementia care. They not only prevent patients from living independently, but also place a considerable burden on caregivers.
Recently, researchers Eunhee Cho and others from Yonsei University in South Korea developed and validated several machine learning models for predicting BPSD.The study has been published in the journal Scientific Reports with the title “Machine learning‑based predictive models for the occurrence of behavioral and psychological symptoms of dementia: model development and validation”.

The research results have been published in Scientific Reports
Paper address:
https://www.nature.com/articles/s41598-023-35194-5
Dataset
This study collected data in three batches, using information from 187 dementia patients for model training, and information from another 35 patients for external validation. The second data collection was a repeated measurement of the participants in the first data collection, and the third data collection recruited new participants for measurement.The data collected in the first and second times are used as training sets, and the data set collected in the third time is used as the testing set.
In order to collect comprehensive characteristic information of the participants,The researchers first investigated their health data (age, gender, marital status, etc.) and personality types before the onset of the disease (Korean Big Five Personality Inventory BFI-K).Second, an actigraph was used to monitor nighttime sleep and activity levels, and finally a symptom diary was used to record the triggers of symptoms perceived by the caregivers (hunger/thirst, urination/defecation, pain, insomnia, noise, etc.) and the 12 BPSDs that occurred daily in the patients.These symptoms are also divided into 7 sub-syndromes.The figure below provides a visual representation of the recording of physical activity recorder and symptom diary data.
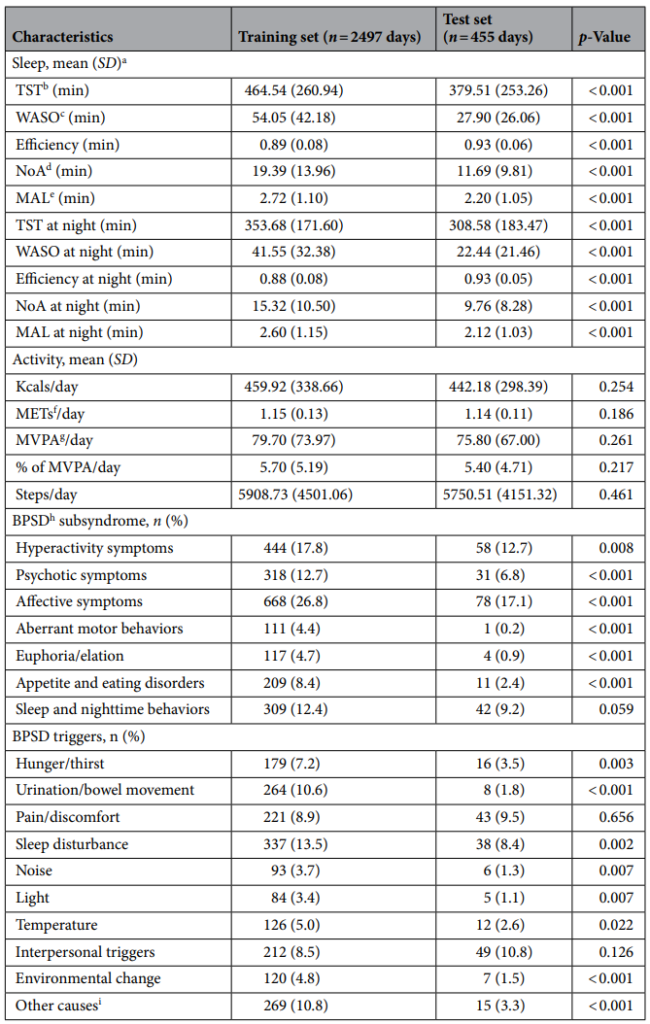
Table 1:Statistics of physical activity recorders and symptom diaries
SD:Standard Deviation
TST:Total sleep time
WASO:Wake-up time after falling asleep
NoA:Number of wake-ups
MAL:Wake up time
METs:Metabolic equivalent
MVPA:Moderate to vigorous physical activity
BPSD:Behavioral and psychological symptoms of dementia
Other reasons:Other caregiver-perceived BPSD triggers (treatment, nightmares, etc.)
However, due to reasons such as participants’ non-compliance or improper wearing of the device, the activity recorder data was missing. According to statistics, the number of participants missing data accounted for 36% of the total number of participants, with an average of 0.9 days of data missing per person.Multivariate imputation was applied using chained equations to handle this missing data.
Experimental procedures
The researchers trained four models to determine the best model for predicting each subsyndrome. Based on the results, the researchers can apply these models to clinical monitoring and prediction of BPSD subsyndromes.At the same time, potential BPSD influencing factors can be intervened to achieve patient-centered dementia care services. In addition, machine learning algorithms can also be embedded in smartphone applications to further enhance their value.
Model performance
The researchers used four machine learning algorithms, including logistic regression, random forest, gradient boosting machine, and support vector machine.The model performance was evaluated by their respective unique learning algorithms, and the best model for predicting BPSD subsyndromes was selected.Here, the logistic regression model is the most common and mature, so it is used as a benchmark model to judge the performance improvement of machine learning.
Based on the training set, through five-fold cross validation,The performance of different models in predicting BPSD subsyndromes is shown in the following figure:
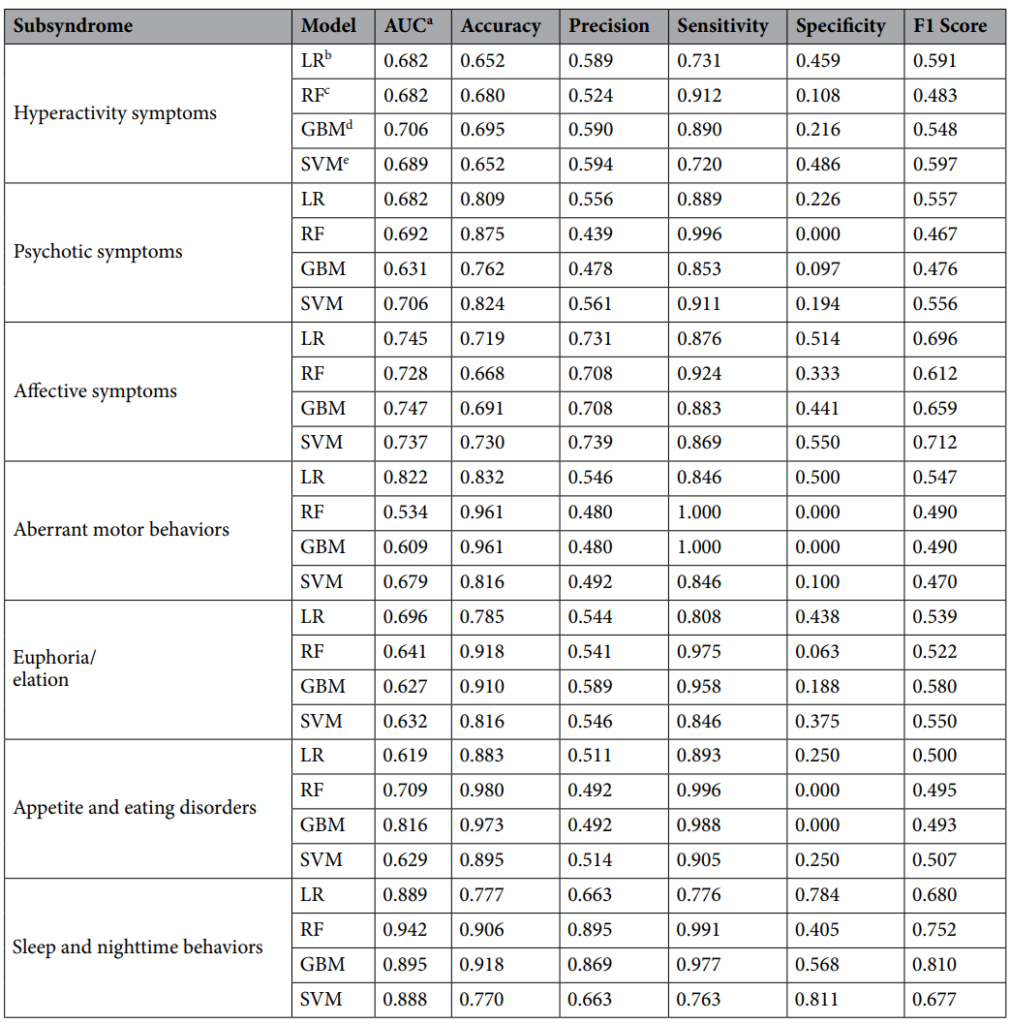
Table 2: Performance of different models in predicting BPSD subsyndromes based on the training set
AUC:Area under the ROC curve
LR:Logistic regression model
RF:Random Forest Model
GBM:Gradient boosting machine model
SVM:Support Vector Machine Model
ROC Curve:The ROC (Receiver Operating Characteristic Curve) curve is a graphical tool for depicting the performance of a classifier.
AUC value:The AUC (Area Under the Curve) value represents the area under the ROC curve and is used to measure the performance of the classifier. The closer the AUC value is to 1, the better the classifier performance is.
Table 2 shows thatThe gradient boosting machine model had higher AUC values in predicting ADHD (0.706), affective symptoms (0.747), and eating disorders (0.816);The support vector machine model had the highest AUC value (0.706) in predicting psychiatric symptoms; the random forest model had the highest AUC value (0.942) in predicting sleep and nighttime behavior; and the logistic regression model had the highest AUC value in predicting abnormal activity behavior (0.822) and pathological euphoria (Euphoria/elation, 0.696).
Model Validation
The researchers used an external validation method to verify the model on the dataset collected for the third time.Based on the test set, the performance of different models in predicting BPSD sub-syndromes is shown in the following figure:
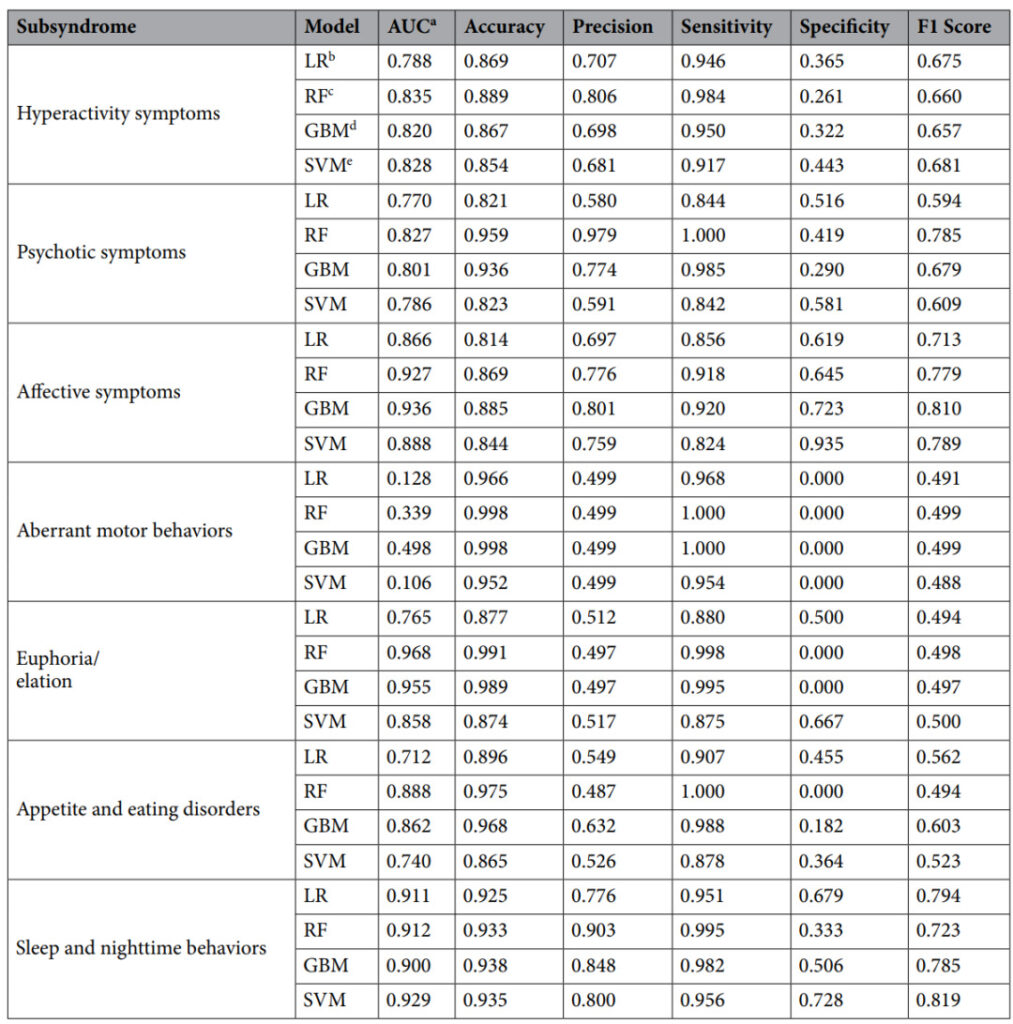
Table 3: Performance of different models in predicting BPSD sub-syndrome based on the test dataset
AUC:Area under the ROC curve
LR:Logistic regression model
RF:Random Forest Model
GBM:Gradient boosting machine model
SVM:Support Vector Machine Model
Table 3 shows thatCompared with the logistic regression model, the machine learning model performs better.Specifically, for most sub-syndromes, the performance of the random forest and gradient boosting machine models is better than that of the logistic regression and support vector machine models; the random forest model has a higher AUC value than other prediction models in predicting ADHD (0.835), pathological euphoria (0.968) and eating disorders (0.888); the gradient boosting machine model has a higher AUC value than other prediction models in predicting psychiatric symptoms (0.801); the support vector machine model has the highest AUC value in sleep and nighttime behavior (0.929).
Combining the information from the two charts, the researchers found that in terms of predicting 7 sub-syndromes,The gradient boosting machine model has the highest average AUC value, which means it performs best.At the same time, the researchers also reminded that when the sample size of the test data set is small, the results of the prediction performance need to be inferred with caution.It is suggested that repeated experiments with larger sample sizes should be conducted in the future to obtain more accurate prediction results.
Domestic achievements: Predicting the onset of dementia ten years in advance
In terms of dementia prediction, in addition to foreign countries, China has also achieved remarkable results.Last September, the clinical research team of Yu Jintai, chief physician of the Department of Neurology at Huashan Hospital Affiliated to Fudan University, in collaboration with the algorithm team of Professor Feng Jianfeng and Young Researcher Cheng Wei from the Institute of Brain-Inspired Intelligence Science and Technology of Fudan University, developed the UKB-DRP dementia prediction model.
The model can predict whether an individual will develop the disease in the next five, ten or even longer years.Screening out people in the early stages of dementia, including all-cause dementia and its major subtypes (such as Alzheimer's disease). The research results have been published in The Lancet's subsidiary journal Electronic Clinical Medicine.
Paper address:
https://www.thelancet.com/journals/eclinm/article/PIIS2589-5370(22)00395-9/fulltext
This research result also demonstrates China's innovative strength and scientific research level in the field of dementia prediction. In the future, with the participation of more institutions and research teams, and the accumulation of more comprehensive and diversified data, we are expected to see more cooperation and progress at home and abroad.With the power of artificial intelligence and big data analysis, we can make greater contributions to the prevention, treatment and management of dementia, and bring more hope and well-being to patients and their families.