Drone + AI Image Analysis: University of Lisbon Efficiently Detects Forest Pests
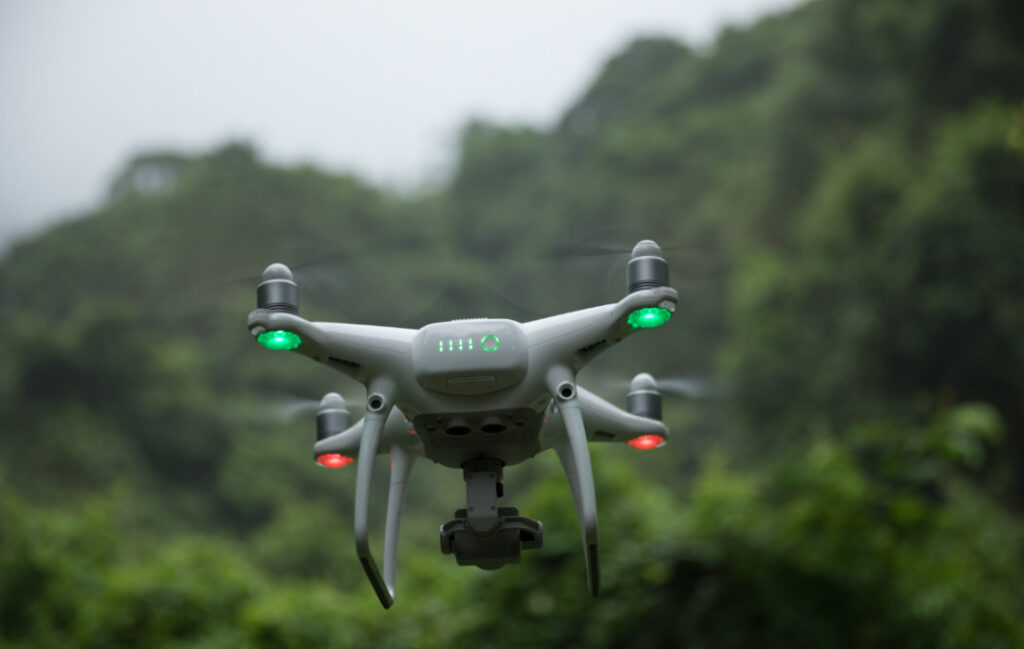
Contents at a glance:Early detection of pests is essential for taking preventive measures tailored to local conditions. Although remote sensing technology can be used to quickly scan large areas, it is not as effective when faced with low-intensity signals or difficult-to-detect objects. Therefore, researchers at the University of Lisbon combined drones with AI image analysis and tested two deep learning methods, FRCNN and YOLO, to detect early nests of pine moths, with significant results.
Keywords:AI Algorithm Pest Detection YOLO
This article was first published on HyperAI WeChat public platform~
Nowadays, with the decline of forest resources and environmental degradation becoming more and more serious, forest pests have become one of the major challenges to global forest protection.Among them, destructive pests such as the pine moth (Thaumetopoea pityocampa) have attracted widespread attention.The pine moth is mainly distributed in southern Europe, the Mediterranean and North Africa. Its larvae will drill holes in the trunks and branches of pine trees and eat, destroying the growth and development of the pine trees.
For early detection and control of the pine moth, researchers from the University of Lisbon compared two deep learning algorithms to tackle the challenge of nest identification in drone imagery.The research has been published in the journal NeoBiota with the title “Testing early detection of pine processionary moth Thaumetopoea pityocampa nests using UAV-based methods”.
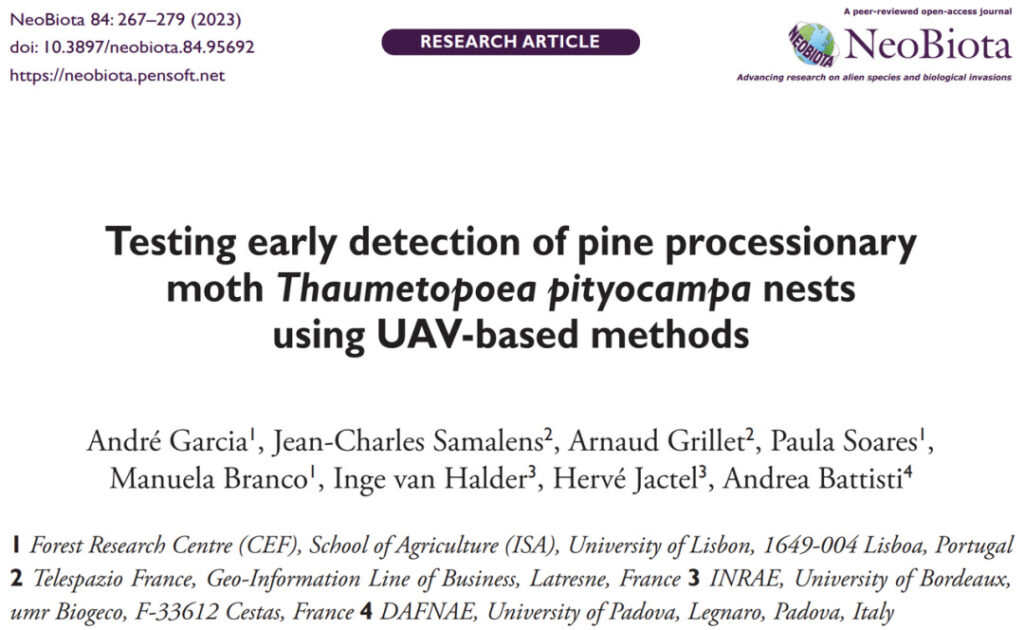
The research results have been published in NeoBiota
Paper address:
https://neobiota.pensoft.net/article/95692/
Experiment Overview
In the past, researchers usually used remote sensing technology (satellites, etc.) combined with multispectral cameras to obtain forest cover images in a certain area and judge the overall pest situation from information such as tree crown color and dead tree location. However, due to the low resolution of the images, it is impossible to detect the pest situation of individual trees.Therefore, in this experiment, the researchers proposed a method of collecting images by drones. In this way, drones can get close to individual trees and scan and collect them in more detail.
The researchers used images captured by drones toTwo deep learning methods, Faster R-CNN (FRNN) and YOLO, were tested to detect early nests of P. pine moth (hereinafter referred to as nests). The specific experimental process is as follows:
Research site selection
The researchers selected 1 study site each in France, Italy, and Portugal.As shown in Figure 1 , characteristics such as tree age and density were different among the three sites.
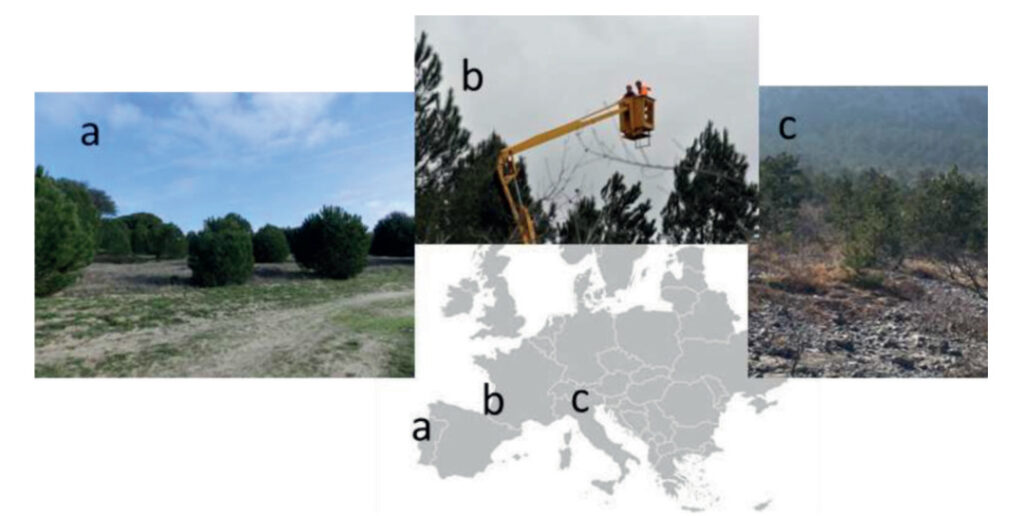
Figure 1: Study location
a: Portuguese pine forest
b: French pine forest
c: Italian black pine forest
In all three sites, researchers used ground counts (two observers visually inspected both sides of the trees) to detect the number of nests.In addition, in the French pine forest shown in Figure 1b, the researchers also stood on a mobile platform 2 m above the tree canopy to detect the number of nests.
Dataset
The researchers used drones and high-definition cameras to collect images of three sample sites.The best application performance solution for the high-definition (HD) camera (RGB HD SONY Alpha 7R) was determined to be: using an RGB HD sensor with a focal length of 35mm and a resolution of at least 36 Mpix, while the drone was selected as the DJI Matrice 300 multi-rotor drone platform, and the overlap within and across the track of 80% was formulated.
In the end, the researchers obtained 22,904 images collected by drones as a dataset, and manipulated the drone images through data augmentation techniques, such as changing brightness, hue, noise, and image compression, to generate a new dataset that enables the model to learn and generalize better.Among them, 80% of this dataset is used for model training, and 20% is used for testing..
Experimental procedures
Drone Model
Considering that some nests can only be seen from the side, the researchers used the model detection mainly for a single drone orthophoto rather than a global orthophoto.,Because the global image is in vertical perspective, it is easy to cause omissions.Drone orthophotos refer to images collected by drones that have been processed so that their position and scale on the map are consistent with their position and scale in the real world.
The research team trained two deep learning models based on FRCNN and YOLO.To evaluate the model's detection results on drone images, an observer was also assigned to visually assess the number of nests in each image.
The researchers used the F1 score to measure the performance of the model compared to human eye detection on drone and ground images..The F1 score calculation formula is as follows:

Figure 2: F1 calculation formula
The F1 score is the harmonic mean of precision and recall and can be used to assess the accuracy and completeness of a model.Its value range is from 0 to 1, and the closer it is to 1, the better the performance of the model.
Experimental Results
The researchers compared the FRCNN and YOLO models with human eye detection.The performance of the model in detecting the presence of nests on trees (% infested trees) and the number of nests (No. PPM nests) was tested.
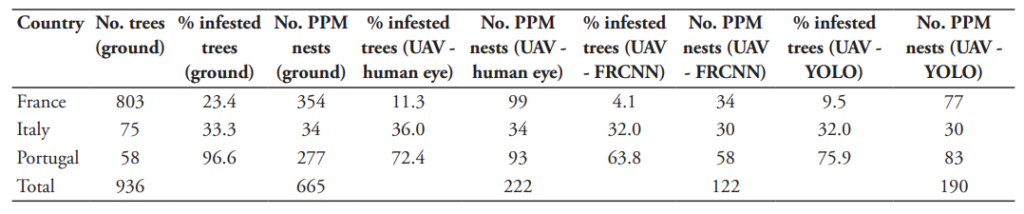
Table 1: Detection of pine moth nests by different methods
As shown in Table 1, a total of 665 nests were visually observed by human eyes in the trees throughout the study area through ground counting, while 222 nests were detected through visual inspection of drone images. The researchers believe that the reason for the difference between the two is that ground visual inspection has a multi-dimensional observation angle, while drones are limited to shooting from above.However, drone imagery has its own advantages as detailed ground inspections are costly and drones can inform people of the risks and take further action to conduct detailed ground inspections.
The figure below shows the F1 scores of the two models for detecting the presence of nests and the number of nests per tree on drone images of three sample plots.
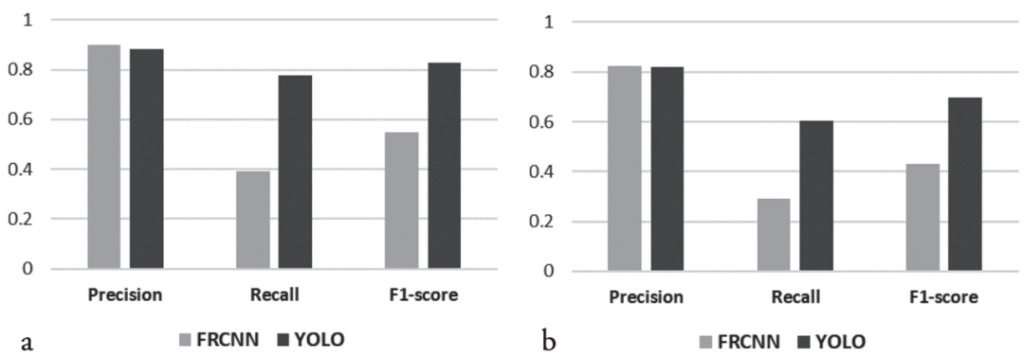
Figure 3: F1 scores of two models for drone image detection
a: Detect the presence of nests on drone images
b: Detect the number of nests on each tree
As shown in Figure 3, the F1 score of the YOLO model for detecting nests in drone images is as high as 0.826, and the F1 score of the YOLO model for detecting the number of nests on each tree is as high as 0.696.At the same time, the researchers found that the detection performance of the YOLO model was higher than that of FRCNN.The figure below shows the F1 scores of the two models when detecting drone images at different study locations (different pine species).
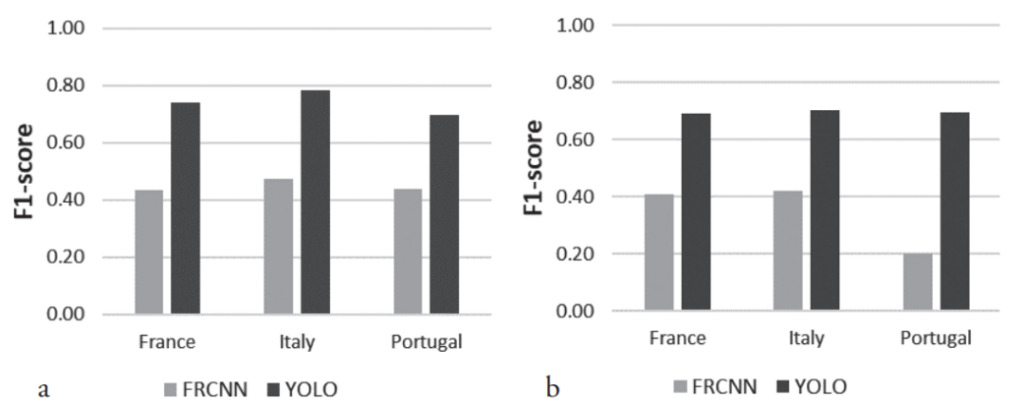
Figure 4: F1 scores of the two models at different study locations
a: Detecting the presence of nests on drone images
b: Detect the number of nests on each tree
As shown in Figure 4, in the three plots, whether detecting the presence of nests or the number of nests on each tree,The F1 score of the YOLO model is better than that of the FRCNN model.
In summary, the researchers proposed thatThe combination of drones and AI models can effectively detect pine moth nests at an early stage.Among them, drones have the following advantages:
- Efficiency: Drones can cover large areas quickly and collect large amounts of data.
- High precision: The high-resolution cameras carried by drones can capture very detailed images and videos, allowing drones to provide high-precision data.
The YOLO model performs well in both nest detection and nest quantity detection on drone images.This shows that the combination of relevant technologies is of great significance in monitoring and managing pests and diseases in forests, and also provides new ideas for protecting forest ecosystems.
Drones + AI: Important trends in technology
At present, drones + AI have become a consensus in the development of forest protection at home and abroad.Through the aerial perspective of drones and the analysis of artificial intelligence, researchers can perform tasks more efficiently, accurately and automatically, thereby improving the efficiency of forest protection.
Focusing on China, the report "Remote Sensing Monitoring of Forest Pests and Diseases - From Satellites to UAVs" released by the Chinese Academy of Sciences details the types, development stages and detection methods of forest pests and diseases., and proposed that one of the important directions for future forest protection work is to develop prediction models and achieve seamless integration of prediction and detection methods, which coincides with the research results of this paper.
Report address:
It can be seen that drones + AI have brought new opportunities and challenges to forest protection, and have played an important role in improving efficiency and protecting forest resources.However, the integration of drones and artificial intelligence also faces a series of challenges.On the one hand, it is necessary to continuously promote the development of drones and artificial intelligence to improve performance and stability. On the other hand, in terms of data security and privacy protection, relevant policies and regulations are needed to ensure that drones and artificial intelligence applications can safely process and store data.
This article was first published on HyperAI WeChat public platform~