Inventory | From Single-objective Optimization to Multi-objective Optimization, 3D Printing Material Development Has Entered the 100X Speed Stage
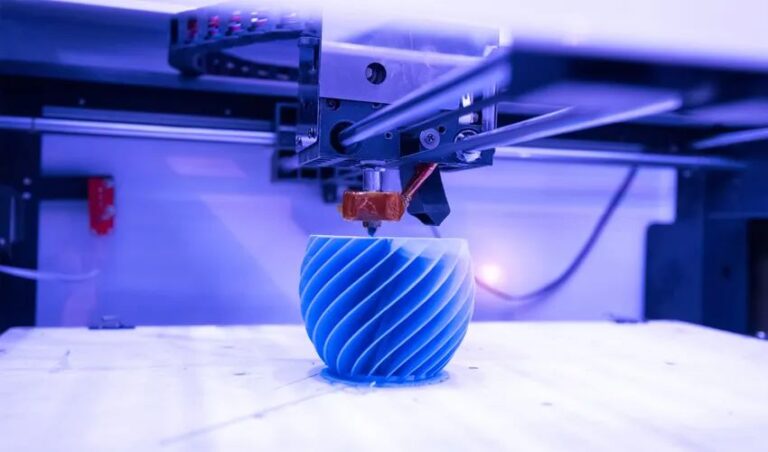
This article will focus on 3D printing material development and use four specific cases to interpret current advanced methods, in order to enable readers to have an overall understanding and grasp of the application of machine learning in material development.
This article was first published on HyperAI WeChat official account~
Represented by AlphaFold, machine learning has achieved encouraging research results in the fields of biopharmaceuticals and protein structure prediction. In particular, the great progress made by geometric deep learning in atomic structure modeling is expected to provide solutions to open problems in computational materials science.
However, compared with drug-like molecules and proteins,Material modeling also faces two main challenges:
* There is no suitable representation method for most materials.Developing a successful machine learning model requires an inductive bias for specific materials, which requires that the input must have a certain format or regularity. For example, we can use 2D graph structures to represent molecules and use sequences to represent proteins. This is not possible in the field of materials. In addition, most materials need to be represented under periodic boundary conditions, which poses great challenges to both representation learning and generative models.
* The material categories are varied and diverse.For example, inorganic crystals, polymers, catalytic surfaces, nanoporous materials, etc. Each material structure has a different representation method and requires a dedicated task/dataset.
Especially taking the development and performance optimization of 3D printing materials as an example,It also faces constraints such as over-reliance on professional knowledge, repeated experiments, and performance trade-offs.This article will focus on this topic and share the current cutting-edge scientific research results and methods.
3D printing + machine learning: impressive results
Focus on single-objective optimization
1. Boston University: A Bayesian experimental autonomous researcher for mechanical design (2020)
Read the full paper:
https://www.science.org/doi/full/10.1126/sciadv.aaz1708
The automatic 3D printing and testing platform based on Bayesian optimization can accelerate the exploration of high compressive toughness structures. Combining Bayesian optimization and high-throughput autonomous experiments,Researchers have developed a Bayesian experimental autonomous researcher (BEAR)In addition to rapid experiments, BEAR also iterates experiments based on all available results.
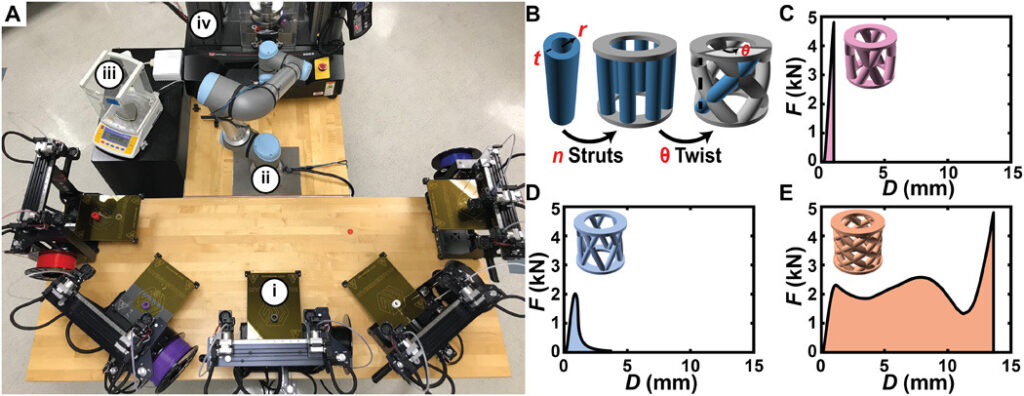
Studying the mechanics of additively manufactured components with BEAR
Using BEAR, researchers can explore the toughness of families of structure parameters while reducing the number of experiments required to identify high-performance structures by nearly 60 times compared to grid-based searches.These results demonstrate the value of machine learning in experimental domains where data is scarce.
2. New York University: Combining automated microfluidic experimentation with machine learning for efficient polymerization design (2020)
Read the full paper:
https://www.nature.com/articles/s42256-020-0166-5
Researchers have developed a microfluidic reactor for screening zirconocene (a compound) catalysts and combined it with the Latin hypercube algorithm to improve the efficiency of catalyst development.

Process flow chart of automatic thermal imaging microreactor system for zirconium metallocene catalyst
During the experiment, the researchers proposed a methodology for studying polymerization reactions using machine-learning-assisted automated microchemical reactors. Using a self-designed microreactor prototype combined with automation and in-situ infrared thermal imaging technology, they conducted efficient and rapid experiments to map the reaction space of zirconium metallocene polymerization catalysts and obtain basic kinetic parameters.
The experiments reduced chemical waste by two orders of magnitude and reduced catalyst discovery from weeks to hours.
3. University of Liverpool, UK: A mobile robotic chemist (2020)
Read the full paper:
https://www.nature.com/articles/s41586-020-2442-2
Researchers have developed a mobile robot (modular, flexible, and able to move on its own, rather than an instrument) to search for better photocatalysts for hydrogen production in water.

The robot operated autonomously for more than 8 days, driven by a series of Bayesian search algorithms, performing 688 experiments in a space of 10 variables.
The results show thatThe self-developed photocatalyst mixture is six times more active than the original formula.
Focus on multi-objective optimization
The birth of an automated quantum dot synthesis robot integrates a machine learning-based experimental selection and fluid chemistry to explore multi-target performance. However, the entire process is carried out in fluid,This also leads to a certain degree of inability to cover some more complex formulation-processing-performance relationships.
At the same time, each experiment is very costly and time-consuming, and the supply of materials is relatively limited;Collecting large amounts of data becomes difficult.In addition, for many real-world applications, multiple performance criteria must usually be met, which increases the complexity of exploring the performance space and makes it difficult to find the optimal solution.
In this case,Multi-objective optimization methods that guide design space sampling can effectively reduce the number of experiments.
1. Massachusetts Institute of Technology: Accelerated discovery of 3D printing materials using data-driven multiobjective optimization
Read the full paper:
https://www.science.org/doi/10.1126/sciadv.abf7435
Researchers use machine learning to accelerate the development of additive manufacturing materials with optimal mechanical property trade-offs. They also developed a multi-objective optimization algorithm that automatically guides experimental design by proposing mixing key recipes to create materials with better performance.
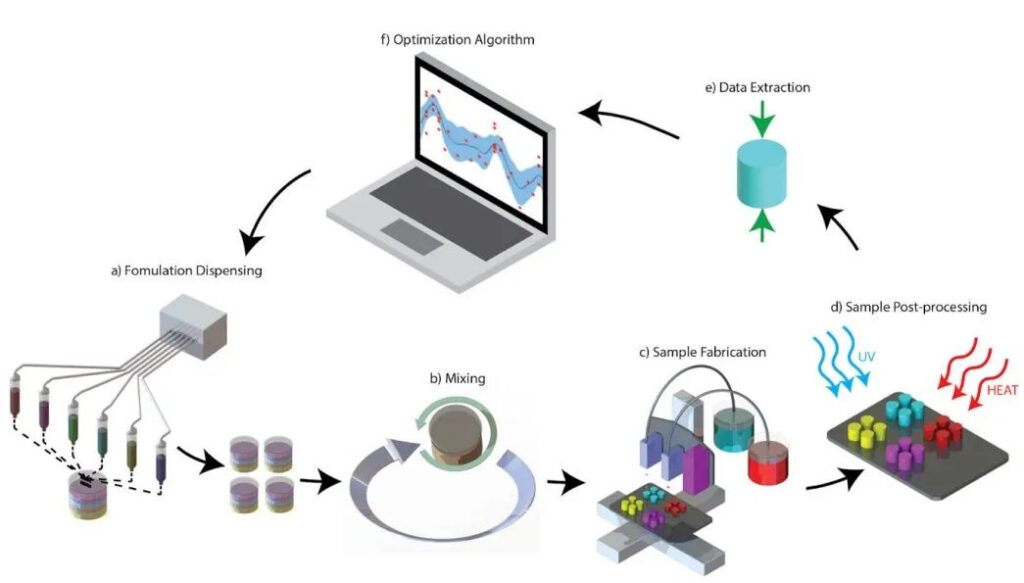
Schematic diagram of the system workflow
( A ) Recipe distribution.
( B ) Formulation mixing.
(C) 3D printer used for sample fabrication.
(D) Sample post-treatment with ultraviolet (UV) curing and heating.
( E ) Compression test of performance data extraction.
( F ) Formulation and performance evaluation of the Bayesian optimization algorithm, outputting the proposed new formulation for testing.
The algorithm, combined with a semi-autonomous manufacturing platform, can significantly reduce the number of experiments performed and the total time to solve the problem.The proposed method develops 12 optimal formulations on its own and expands the discovered performance space by 288 times after 30 experimental iterations.This approach is expected to be easily generalized to other material design systems and enable automatic material discovery.
The experimental results show thatIt only takes 6797 seconds (less than 2 hours) to complete an iterative cycle of the experiment.Only the step of transferring samples needs to be done manually, which greatly accelerates the development process of 3D printing materials.
Another blue ocean market, who will win with a smile?
Alibaba Cloud's "2021 3D Printing Industry Development Research Report" shows that in recent years, 3D printing has received widespread attention from the international community. Take the United States as an example. It has invested a lot of manpower, material and financial resources in 3D printing technology, developed more abundant printing materials, and promoted the maturity of this technology. At present, 3D printing has also become one of the fastest growing industries in the United States.
In contrast, China started late in material development, but with the country's focus, the market potential of the 3D printing industry is undoubtedly huge. An industry organization predicts that China's 3D printing market is expected to grow to 63.5 billion yuan in 2025, of which 3D printing materials account for about one-third, and the market size will reach tens of billions. It can be inferred that the 3D printing industry has become one of the core technologies leading a new round of industrial transformation. As the mid- and upper-stream parts of the industry, the importance of 3D printing materials is self-evident.
Now, in this huge blue ocean,Machine LearningWith its powerful predictive performance, it is accelerating the development of the 3D printing industry. Therefore, for many companies, who can seize the opportunity first and win in it will also meanWill successfully stand on the cusp of change.