Use YOLO v5+DeepSORT to Create a Real-time Multi-target Tracking Model
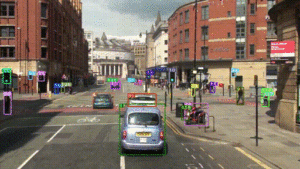
Object Tracking is an important topic in the field of machine vision.It can be divided into single object tracking (Single Object Tracking, referred to as SOT) and multi-object tracking (Multi Object Tracking, referred to as MOT).
Multi-target tracking is often prone to target loss due to the large number of tracking IDs and frequent occlusions. With the help of the tracker DeepSORT and the detector YOLO v5, a high-performance real-time multi-target tracking model can be built.
This article will introduce single target tracking and multi-target tracking respectively. At the end of the article, the implementation process and specific code of YOLO v5+DeepSORT will be explained in detail.
Detailed explanation of single target tracking
definition
Single target tracking (SOT) means giving the target in the first frame of the video, locating the target in subsequent frames based on context information, and establishing a tracking model to predict the target's motion state.
Application Scenario
SOT is widely used in intelligent video surveillance, autonomous driving, robot navigation, human-computer interaction and other fields.

Research Difficulties
The three main difficulties are: changes in the target background, changes in the object itself, and changes in light intensity.
Mainstream algorithms (based on deep learning)
There are two main approaches to solving the SOT problem:Discriminative tracking and generative tracking,With the successful application of deep learning in machine vision-related tasks such as image classification and target detection,Deep learning has also begun to be widely used in target tracking algorithms.
This article mainly introduces the SOT algorithm based on deep learning.

Key algorithm: SiamFC
Different from the online learning methods used in traditional object tracking, SiamFC focuses on learning strong embeddings in the offline stage.
It combines a basic tracking algorithm with a novel fully-convolutional Siamese network trained end-to-end on the ILSVRC15 dataset.For object detection in videos.

Experiments have shown that the twin fully convolutional deep network makes more efficient use of existing data during model testing and training.
SiamFC pioneered the application of twin network structures in the field of target tracking.The tracking speed of the deep learning method tracker is significantly improved, with a simple structure and excellent performance.
Related derivative algorithms
1. StructSiam
A local structure learning method is proposed, which considers both the local pattern and structural relationship of the target.To this end, the authors designed a local pattern detection module to automatically identify the discriminative areas of the target object.
The model can be trained in an end-to-end manner.
2. SiamFC-tri
The author proposed a new triplet loss.Used to extract expressive deep features of tracked objects. Without increasing the input, this method can use more elements for training and achieve more powerful features by combining original samples.
3. DSiam
The authors proposed a dynamic twin network,Through a fast conversion learning model, it is possible to effectively learn the appearance changes of the target and the background suppression of the previous frame online. At the same time, the author also proposed element multi-layer fusion, using multi-layer deep features to adaptively integrate network outputs.
DSiam allows the use of any feasible general or specially trained features, such as SiamFC and VGG, and the dynamic twin network can be integrated and trained directly on labeled video sequences, making full use of the rich spatiotemporal information of moving objects.
Detailed explanation of multi-target tracking
definition
Multi-object tracking (MOT) refers to assigning an ID to each object in each frame of the video and drawing the behavior trajectory of each ID.

Application Scenario
MOT is widely used in smart security, autonomous driving, medical scenarios and other fields.
Research Difficulties
The biggest challenge MOT currently faces is occlusion, which is the occlusion between targets or the occlusion of targets by the environment.
Mainstream Algorithms
1. SORT
Simple Online and Realtime Tracking (SORT) is a multi-target tracking method that focuses on simple and efficient algorithms.It is very practical and can effectively associate targets for online and real-time applications.

Due to the simplicity of the tracking method, the tracker can update at a rate of 260 Hz, which is 20 times faster than the most advanced trackers at the time.
2. DeepSORT
DeepSORT is an upgraded version of SORT, which integrates appearance information to improve the performance of SORT.This allows us to track the target normally even when encountering long periods of occlusion, and effectively reduces the number of ID transitions.

The authors put most of the computational complexity into the offline pre-training phase, where they use a large-scale person re-identification dataset to learn a deep association metric.
In the online application phase, neighbor queries in the visual appearance space are used to establish measurement-to-track associations.
Experiments show that DeepSORT reduces the number of ID conversions by 45% and has excellent overall performance at high frame rates.
In addition, DeepSORT is a very general tracker that can be connected to any detector.
3. Towards Real-Time MOT
The authors proposed a MOT system,This enables object detection and appearance embedding to be learned in a shared model.That is to say, the appearance embedding model is incorporated into a single-shot detector so that the model can output the detection and the corresponding embedding at the same time.
The authors further propose a simple and fast association method that can be run together with the joint model.

The computational cost of both components is significantly reduced compared to previous MOT systems, providing a clean and fast baseline for subsequent work on real-time MOT algorithm design.
This is the industry's first near real-time MOT system.It runs faster, has higher accuracy, and its code is open source, making it very worthwhile for reference.
Multi-object tracking with YOLOv5 and DeepSORT
This tutorial is in OpenBayes.com run. OpenBayes is an out-of-the-box machine learning computing cloud platform that provides mainstream frameworks such as PyTorch and TensorFlow, as well as various types of computing solutions such as vGPU, T4, and V100. The pricing model is flexible and simple, and charges are based on usage time.
This tutorial uses vGPU to run in the PyTorch 1.8.1 environment.

This project consists of two parts. First, the YOLO v5 detector is used to detect a series of objects; then DeepSORT is used for tracking.
The first step is to prepare the code environment
%cd Yolov5_DeepSort_Pytorch
%pip install -qr requirements.txt # 安装依赖
import torch
from IPython.display import Image, clear_output # 显示结果
clear_output()
print(f"Setup complete. Using torch {torch.__version__} ({torch.cuda.get_device_properties(0).name if torch.cuda.is_available() else 'CPU'})")
Step 2 Preprocess the video to be tested
!y | ffmpeg -ss 00:00:00 -i test.avi -t 00:00:03 -c copy out.avi -y
Step 3: Model inference
!python track.py --yolo_weights /openbayes/input/input1/crowdhuman_yolov5m.pt --source out.avi --save-vid
Step 4: Format conversion
!ffmpeg -i /openbayes/home/Yolov5_DeepSort_Pytorch/inference/output/out.avi output.mp4 -y
Step 5: Display the results
from IPython.display import HTML
from base64 import b64encode
mp4 = open('output.mp4','rb').read()
data_url = "data:video/mp4;base64," + b64encode(mp4).decode()
HTML("""
<video controls>
<source src="%s" type="video/mp4">
</video>
""" % data_url)

About OpenBayes
OpenBayes It is a leading machine intelligence research institution in China.Provides a number of basic services related to AI development, including computing power containers, automatic modeling, and automatic parameter adjustment.
At the same time, OpenBayes has also launched many mainstream public resources such as data sets, tutorials, and models.For developers to quickly learn and create ideal machine learning models.
Visit Now openbayes.com and register
Enjoy now
600 minutes/week of vGPU
And 300 minutes/week of free CPU computing time
You can also input a video and get the corresponding detection and tracking results.