How to Use Random Forest Algorithm to Fatten a Group of Fish in the Deep Sea
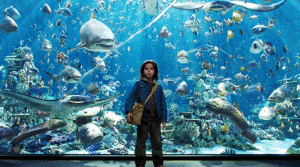
Friends who love sashimi must have heard of Yellowtail Kingfish.In restaurants, yellowtail amberjack is often referred to as yellowtail croaker or yellowtail amberjack.
This large deep-sea fish has dense meat, rich in Omega-3 and no fishy smell, and tastes first-class, and is regarded as the best sashimi. The price of wild yellowtail amberjack has always remained high due to factors such as the short fishing season and the difficulty of fishing.
How to fatten and keep marine life healthy
Yellowtail amberjack grows fast, but there are not many wild populations, so it is difficult to catch them commercially. With the development of marine aquaculture technology, yellowtail amberjack can also be cultivated to meet the growing consumer demand.
For marine aquaculture,How to fully understand the living habits and spawning characteristics of yellowtail amberjack and protect mature yellowtail amberjack in a targeted manner, becoming the key to improving breeding production efficiency and profitability.

They are extremely intelligent, and sea fishing and catching them is very difficult.
Yellowtail amberjack is a fish that migrates periodically with seasons and temperature changes. It is mainly distributed in the waters of offshore rocky reefs far from the coast of my country, Japan, the Korean Peninsula, and Australia.
In the past decade, thanks to the development of observation equipment, the field of biological tracking has made significant progress, and many breakthroughs have been made in the remote monitoring of species.More and more observations and data collection on the behavior of marine organisms are beginning to use three-axis accelerometers on a large scale.

Monitoring blacktip shark movement behavior
The three-axis accelerometer measures the acceleration of three axes.It can generate time series describing biological movements and activities, and then deduce animal behavior based on the characteristics of acceleration in the environment.
In addition, accelerator data can be combined with spatiotemporal data (depth, geographic location, season, etc.) for analysis.To determine ecologically important behaviors such as spawning and feeding.
The amount of data collected by the three-axis accelerometer is huge, even up to millions of rows (including acceleration, body position, etc.), so a semi-automatic analysis system is needed to classify biological behavior.
The movement behavior of yellowtail kingfish is also very complex, with an average speed of 30 to 40 kilometers per hour, and it can even exceed 100 kilometers per hour when chasing prey.This has hindered the development of automated analysis technology.
Machine learning comes in handy for complex biological data
Faced with a large amount of multi-dimensional and complex spatiotemporal data including movement speed, time, depth, geographic location, etc.Machine learning has become the first choice for scientific researchers.
Thomas Clarke, a PhD student in the Faculty of Science and Engineering at Flinders University,Based on 624 hours of accelerator data from six farmed yellowtail kingfish during their spawning period, a random forest machine learning algorithm was trained to identify five different behaviors of yellowtail kingfish (swimming, feeding, injury, escape, and courtship).
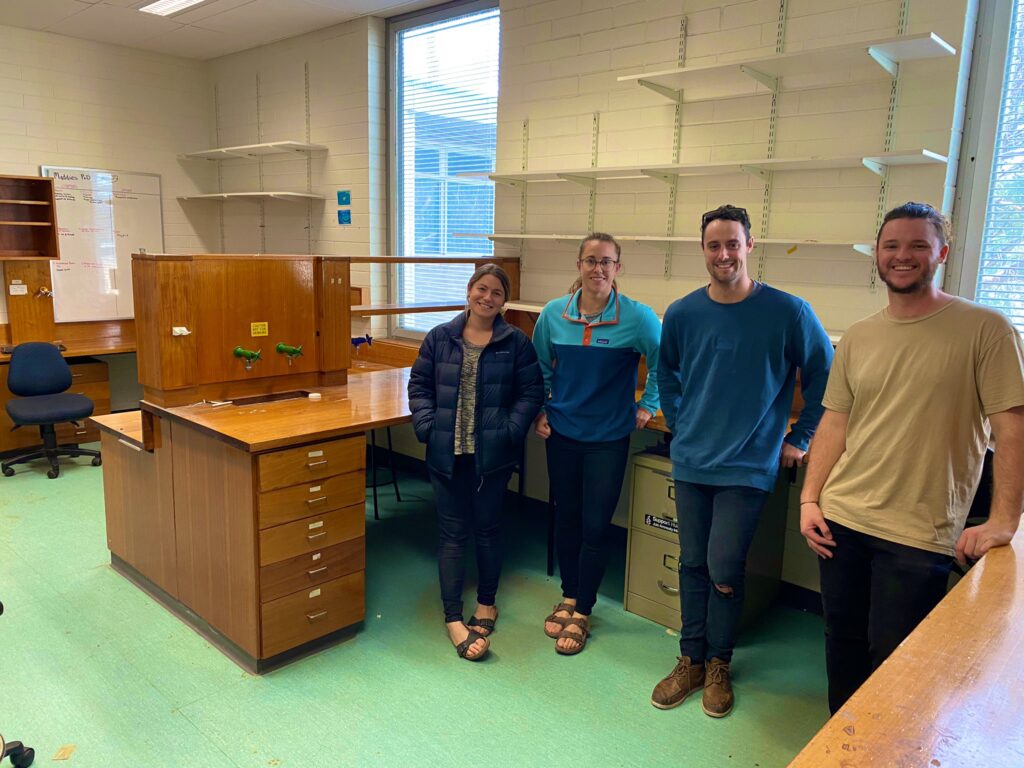
Thomas Clarke (right)
This is the first study to use machine learning to identify spawning behavior in wild yellowtail kingfish.This is of great significance for using AI to better understand the reproductive patterns of deep-sea fish.
In this study, Thomas Clarke and colleagues characterized and quantified the behavior of farmed yellowtail kingfish, building on data from the Benchmark Truth Accelerator.A supervised machine learning algorithm (RF model) was developed.
The model was then used to analyze wild yellowtail kingfish data to predict naturally occurring spawning behavior.
Farmed wild two-headed croaker, detailed test method
1. Experiment on breeding yellowtail kingfish
Sexually mature yellowtail kingfish (wild yellowtail kingfish caught in South Australia) were stocked in large containers and their spawning behavior was continuously observed.
From August 2018 to February 2019, two tracking missions were carried out.One female and two males were tracked each time. The behavioral data of six farmed yellowtail amberjacks were recorded using a three-axis accelerometer data logger.

The yellowtail kingfish in the container were taken out and placed in the device containing AQUI-S (10ppm) and marked.The recorder is fixed on the back of the yellowtail kingfish. In order to distinguish different individuals, the accelerator buttons, night mode, etc. are differentiated.Three hours after the tagging was completed, the behavioral data of yellowtail amberjack were recorded.
2. Wild yellowtail kingfish test
Between October 2015 and November 2019,The researchers captured and tagged eight wild yellowtail amberjacks of similar size to farmed yellowtail amberjacks, and used an accelerometer to record data for 2-3 days.
Wild yellowtail amberjack has the same specifications as farmed yellowtail amberjack, with a fixed accelerator. The difference is that the accelerator for wild yellowtail amberjack is a self-detachable and recoverable installation package.It includes an accelerometer, a radio transmitter, and a smart positioning and temperature transmission tag that will fall off on its own after 2-3 days.
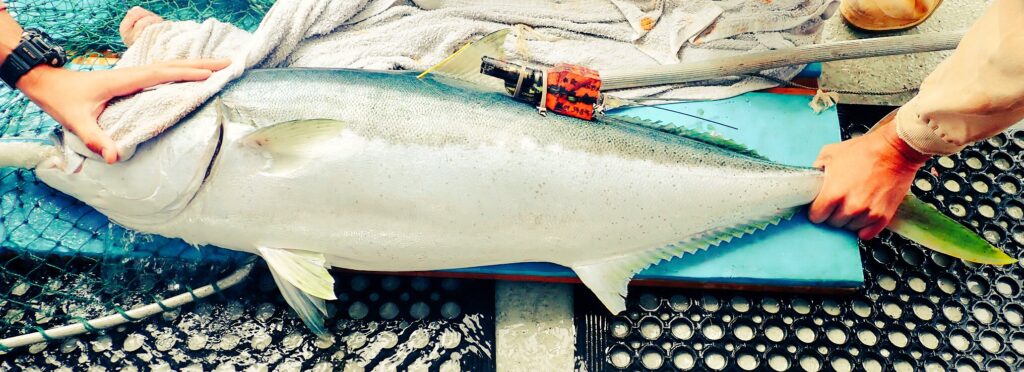
3. Data Analysis
Accelerator data can be downloaded via IGOR Pro and visualized using Ethographer software.
First, invalid data due to insufficient light or the camera not capturing the target is deleted.
Calculate the static acceleration and dynamic acceleration on the three acceleration axes,Filter out dominant signals caused by tail slapping and body posture, and isolate behaviors with high amplitude accelerations.
By observing the time series acceleration data, the potential explosive behavior of yellowtail kingfish can be determined.Five behavioral categories of yellowtail kingfish can be observed from the videos: eating, swimming, fleeing, courting, or injured.
4. Machine learning classification algorithm development
Predictions are made based on random forest classification.A single dataset was formed with the values of all predictor variables and then randomly split into two parts: 70% was used for model training and 30% was used as a validation set to evaluate model performance.
The ntree value started from 500 and gradually increased to 2000. In addition, the number of variables randomly sampled for each mtry was tested to evaluate the impact on the model error rate.
In the process of model construction, the authors used 64 predictor variables to further ensure the accuracy of the model.
5. Model performance evaluation
Performance metrics of classification models, calculated from the RF confusion matrix.The confusion matrix determines the true positive (TP), false positive (FP), and false negative (FN).A comparison table of the actual observed values of all behavioral categories of yellowtail kingfish and the model predicted values is also provided.
6. Predicting the behavior of wild yellowtail kingfish
Use RF algorithm to predict the behavior data of 8 wild yellowtail amberjacks.It was found that the overall accuracy of the model reached 94%.
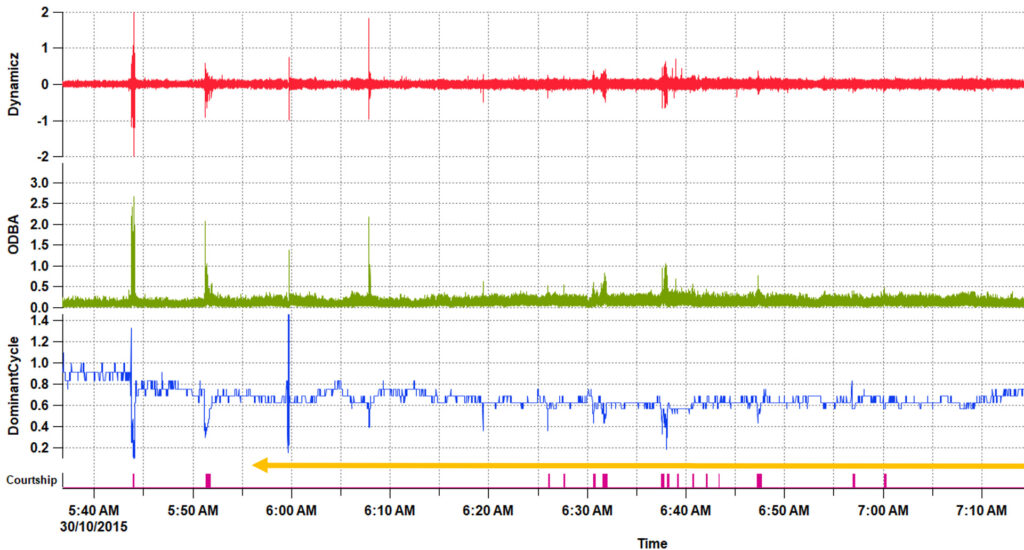
and reproductive behavior (n=1, orange arrow)
Swimming and feeding had the highest recognition accuracy, exceeding 84%, followed by courtship, escape, and injury with lower classification accuracy scores.

The grey boxes represent the number of correct classifications in the test set.
Understanding the ocean is a long way to go
The ocean is the earth's treasure trove of resources.
In addition to their economic value, marine life is also the key to the ecological balance of the ocean. Studying the ocean can help us better understand marine life, energy and other resources, and is also an important topic for the rational development and protection of marine resources.
