No Time to Watch Movies or TV Series, AI Turns Movies and TV Series Into Comics With One Click
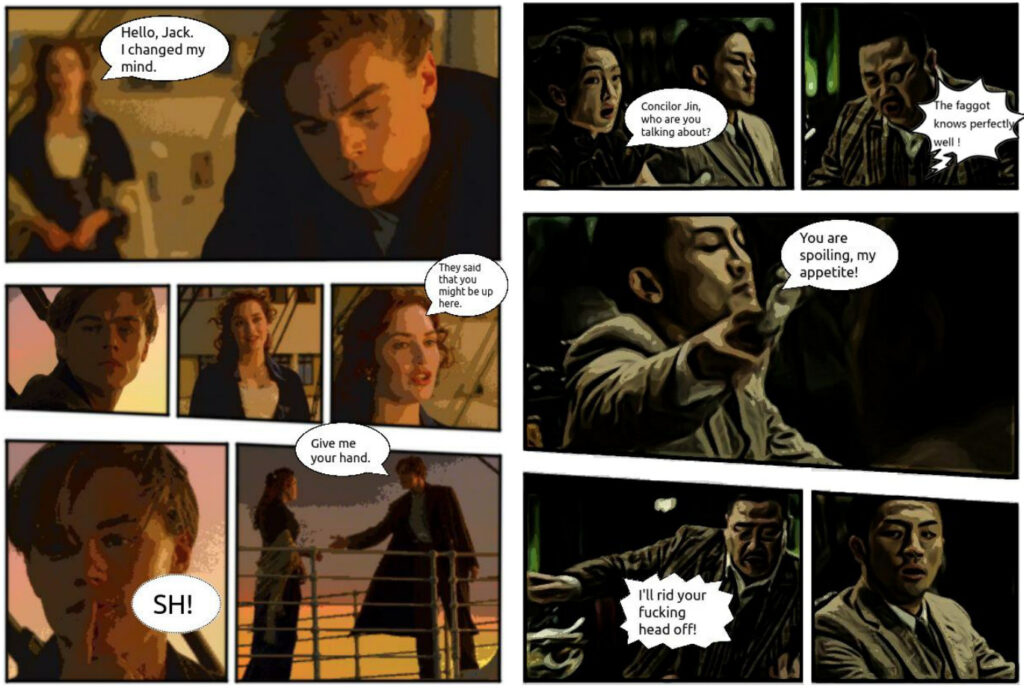
What kind of magical operation is it to turn movies and TV series into comics? A team from Dalian University of Technology and City University of Hong Kong has recently proposed an AI framework that can automatically convert movies and TV series into comics. From now on, there is another way to watch movies and TV series.
Nowadays, movies, TV series and all kinds of videos have become an indispensable part of our lives. According to reports, the total length of videos uploaded to YouTube every day would take a person more than 82 years to watch.
In order to save time in watching TV series, 2x speed playback has become the standard for binge-watching TV series.In addition to watching at double the speed, in a jumpy way, and listening to film critics' comments, there is another way to catch up quickly on TV series, which is to turn the TV series into comics.
Recently, researchers from Dalian University of Technology and City University of Hong Kong published an interesting study that can automatically generate pictures from TV series, movies or other videos into comic form and add text bubbles.
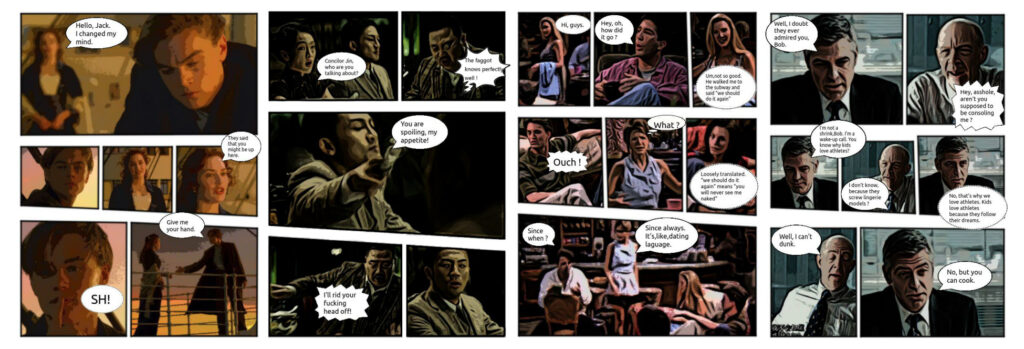
"Compared with the state-of-the-art comic generation systems, our system can synthesize more expressive and attractive comics," the researchers said in the paper. "In the future, this technology will be extended to generate comics using text information."
I’ve seen comic adaptations, but have you ever seen “adapted comics”?
Previously, there have been some similar research results in the industry, proposing automated systems for converting videos into comics, but there is still room for improvement in terms of automation, visual effects, readability, etc. Therefore, this research direction is still full of challenges.
A team from Dalian University of Technology and City University of Hong Kong recently published a paper"Automatic Comic Generation with Stylistic Multi-page Layouts and Emotion-driven Text Balloon Generation"A better method is proposed in .

Paper address: https://arxiv.org/abs/2101.11111
The paper proposes a fully automatic comic generation system.No needWith any manual adjustments by the user, any type of video (TV series, movies, cartoons) can be generated into high-quality comic pages and the character dialogues can be converted into bubble text.Moreover, the comics generated by the system have rich visual effects and are highly readable.
Three modules, turning film and television dramas into comic books
The key idea proposed in this paper is thatDesign systems in a fully automatic manner without any manually specified parameters or constraints.At the same time, the team selectively introduced user interactions to make the design more personalized and diverse.
In general, the system has three main modules:Keyframe selection and comic stylization, multi-page layout generation, text bubble generation and placement.
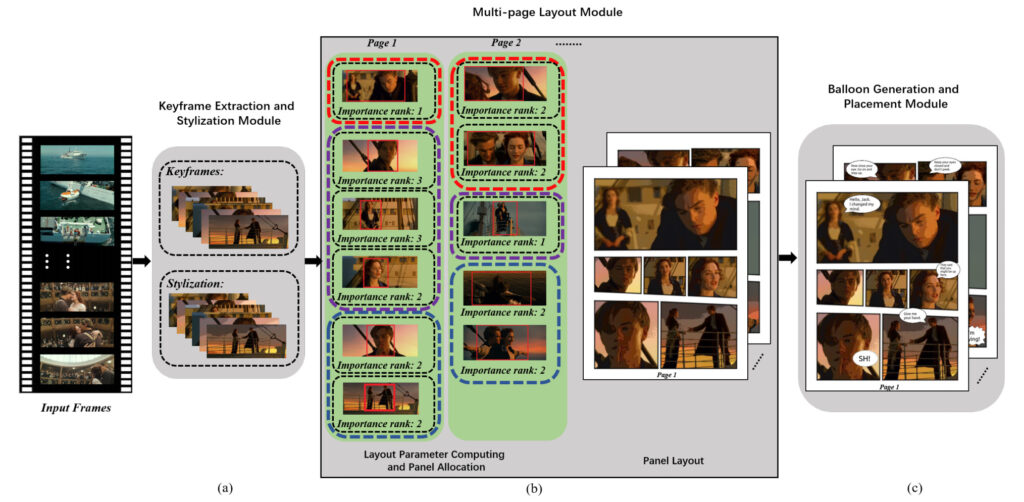
Module 1: Keyframe extraction and stylization
The input of the system is a video and its subtitles, which contain dialogues and corresponding start and end timestamp information.
They first selected a frame from the original video every 0.5 seconds, then used the temporal information in the subtitles and the similarity between two consecutive frames to select informative key frames. Finally, they stylized the key frames, which means converting ordinary images into comic-style images.
Keyframe extraction
The selection of key frames is a particularly important and difficult task. The team mainly uses time information to make the selection.

As shown in the figure above, the team first used the start and end time of each subtitle to split the video into multiple shots. These shots are divided into two types: dialogue shots (shots with subtitles) and non-dialogue shots (shots without subtitles).
For dialogue shots:The system calculates the GIST similarity between two consecutive frames obtained previously (if the GIST similarity is small, the difference between the two frames is large).
During the execution, if the similarity is less than the preset threshold value ?1, the next frame will be selected as the key frame.If none of the frames corresponding to a group of subtitles are selected, the middle frame is selected as the key frame.
Considering that a continuous dialogue and the same scene may correspond to multiple subtitles, the team will calculate the GIST similarity between the previous continuous key frames. If the similarity is greater than the set threshold of ?2, they are considered to belong to the same scene. Then, only one of the key frames is retained and the subtitles are merged.
In addition, in the same set of subtitles, the system may select multiple key frames because after calculation, it may be found that these key frames have semantic relationships and these key frames will be used for multi-page layout.
For non-dialogue shots:The system will first select the frame that is most different from the frame in the current shot. In order to reduce the redundancy of selected frames, the system will calculate the GIST similarity between this shot and the previously selected key frame. Only when it is less than the previously set threshold will it be selected as a key frame.
Finally, the subtitle sets are grouped by comparing the start timestamp with the timestamp of the keyframe. Any subtitles that fall within the range of the start and end timestamps of a keyframe will be collected together.
Stylized picture
The team used the extended Gaussian difference method to convert the source image into a black and white image. Afterwards, 128 levels of color quantization were performed to obtain the quantized image and achieve color stylization. In this way, a series of real-life movie shots were transformed into a comic style.
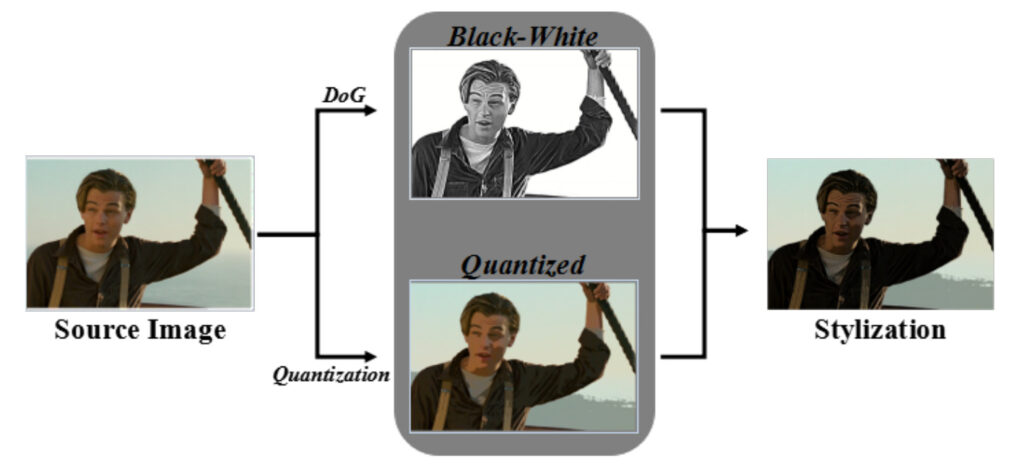
Module 2: Multi-page layout
The team proposed a multi-page layout framework to automatically allocate and organize page layouts while presenting richer visual effects.
In this module, we first need to calculate four key factors to guide the generation of multi-page layouts, including: the region of interest (ROI) of keyframes, the importance level of keyframes, the semantic relationship between keyframes, and the number of panels on a page.
The team then proposed an optimization-based panel assignment method to assign key frames to a page sequence and used a data-driven comic layout synthesis method to generate the layout of each page.
Friends who follow comics know that the number of frames on each page of a comic book is not fixed. In order to give readers a better reading experience, cartoonists will arrange the number of frames according to the plot.
In this study, the team treated this problem as a global optimization problem to complete the allocation of each shot in the comic page.
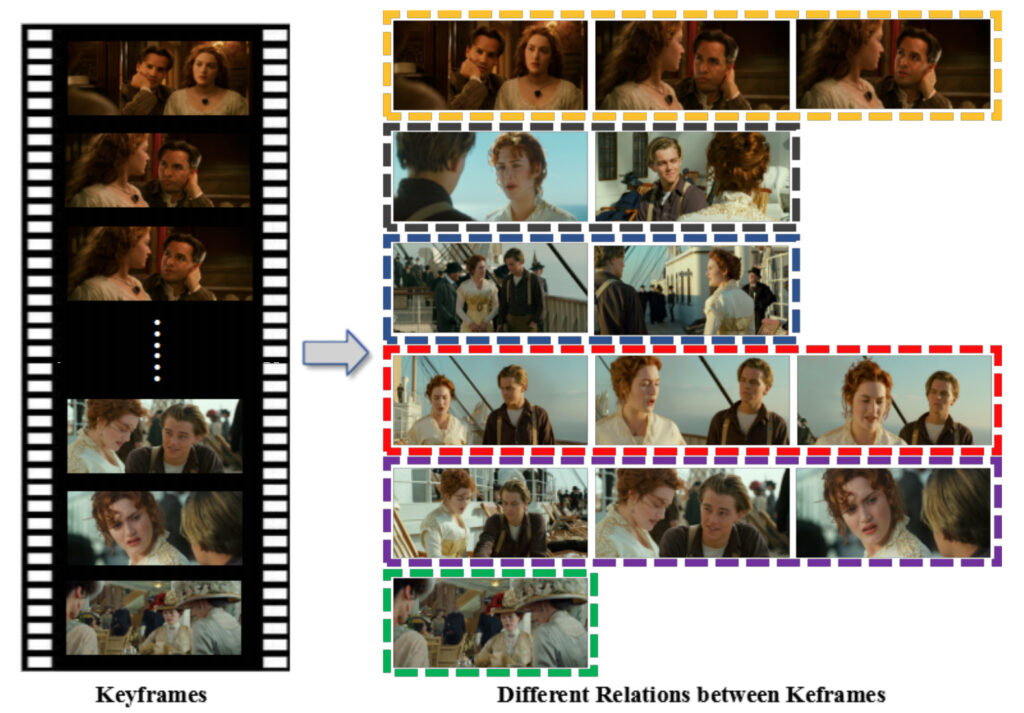
Module 3: Generation and placement of text bubbles
Generating text bubbles
Usually in comics, authors will choose different bubble shapes for dialogues in different situations and emotions, which is very important for the expression of comic content. However, existing related studies generally only use basic elliptical bubble shapes, which are not rich enough for emotional expression.
An important result proposed in this paper is a bubble generation method based on emotion perception, which can use video audio and subtitle information containing emotions to generate text bubble shapes that are suitable for them.
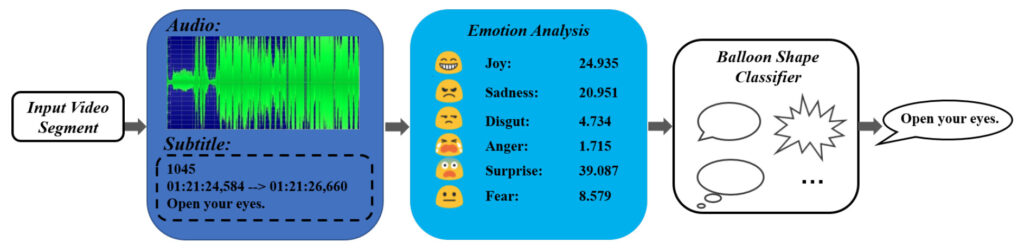
In this system, the author uses three common bubble shapes: oval bubbles, thought bubbles and jagged bubbles. These three bubbles are suitable for emotions: calm emotions, thoughts (unspoken), and strong emotions.
For the training of the bubble classifier, the team mainly used some anime videos and corresponding comic books to collect data on audio emotions, subtitle emotions, and bubble types.
Bubble Positioning and Placement
Similar to the previous method, this paper also uses speaker detection and lip movement detection to obtain the position of the person speaking in a frame, and then places the balloon near the person to whom it belongs.
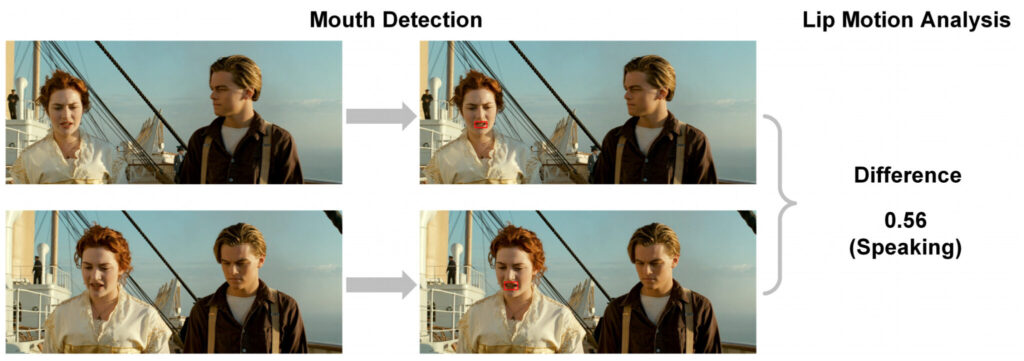
The specific execution process is as follows:
- First, use the "Dlib" face detection Python library to detect the mouth of each character in a frame;
- Then, lip movement analysis is used to calculate the mean square difference of the pixel values of the mouth area between two consecutive frames. The difference is calculated on the search area around the mouth area in the current frame to determine whether the lips are moving;
- Finally, set a threshold to determine whether a character is speaking.
Once you have the speaker's location, place the text bubble near it, with the tail of the bubble pointing toward the speaker's mouth.
Use four classic films to evaluate the system effect
To test the model, the team input 16 clips from four different films: Titanic, The Message, Friends, and Up in the Air.
The duration of the input videos ranged from 2 to 6 minutes, and each clip contained a portion with dialogue.
For each clip, the team recorded how long it took to generate a comic book using the system and calculated the average time taken to evaluate the system's performance.

The authors concluded that the method in this study is superior to other methods in the following three aspects:
- First, the system can generate richer bubble shapes for conversations, while existing methods only use simple elliptical word balloons;
- Secondly, using the text summarization method, some related subtitles are merged, which can ensure that the sentences in the text bubbles are not too long and enhance readability;
- Third, by automatically obtaining four important parameters, fully automatic multi-page layout is achieved (previous methods were mostly semi-automatic and required manual intervention), and the layout results are reasonable and rich.
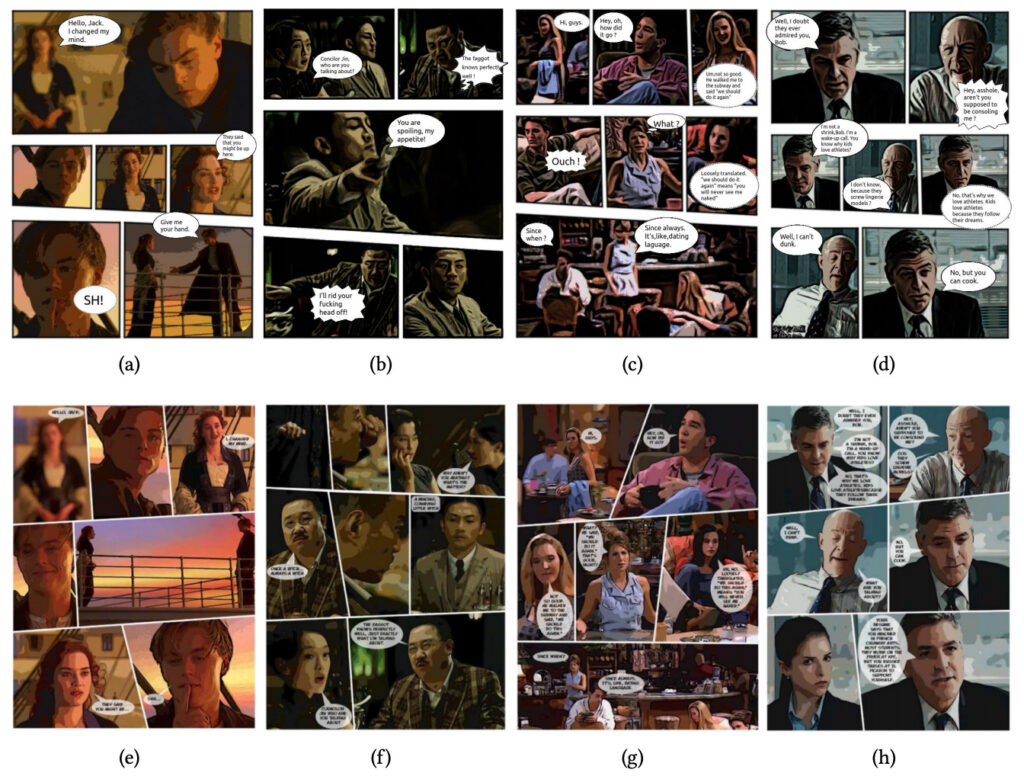
To avoid interference from subjective factors, the team also recruited 40 volunteers through Amazon Mechanical Turk to compare the team's generated results with those generated by other similar systems.
Volunteers first watched the original video, then read the cartoons generated by various methods and gave their ratings. To avoid subjective bias, the videos and corresponding cartoons were randomly arranged.
The end result was that the system received better ratings than other methods, regardless of whether the volunteers had seen the videos before.
Generate comics with one click, what else can you do?
Although it has received positive reviews from users, the system is certainly not perfect and there are still some issues to be resolved.
For example, when selecting key frames, there may still be a situation where the similarity is too high, which will cause redundancy in the picture.
In addition, if the input video does not have subtitles, the system must first extract the lines through voice recognition before generating comics, but the results of voice recognition are often prone to errors, so this is also a challenge faced by the system. However, the team said that they believe that with the continuous advancement of voice recognition technology, this problem will be solved in the future.
In the future, when this technology is mature enough, many video works will have an additional way to open them. "Watching" a film in the form of comics may bring more imagination space for readers.
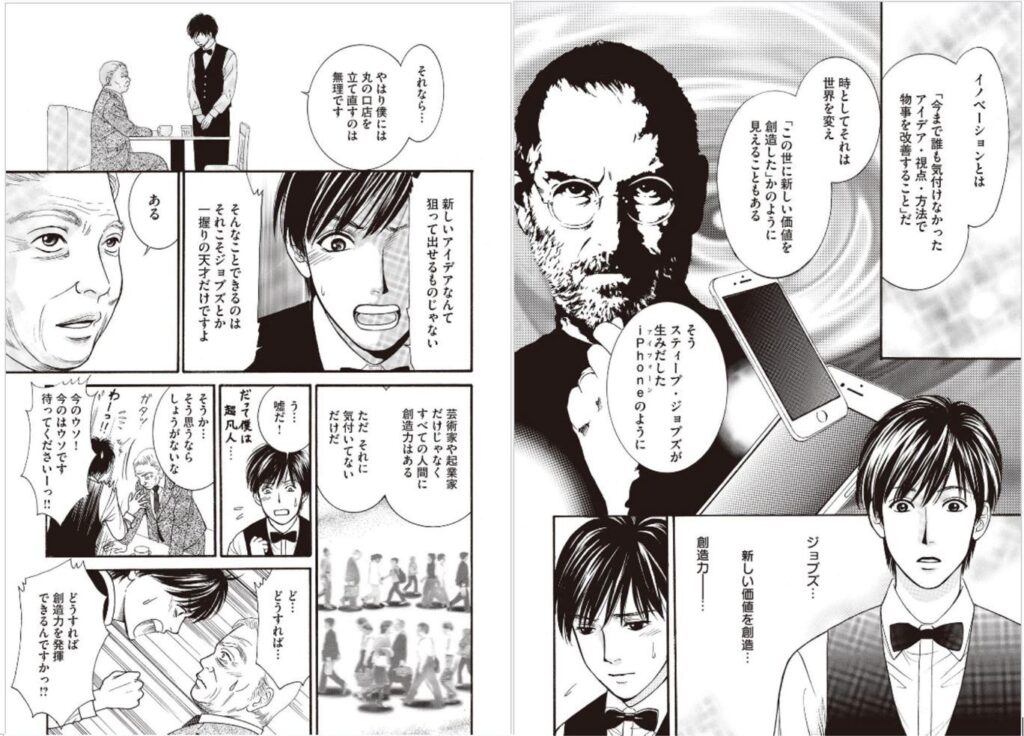
In addition, ordinary people can easily convert videos into comics without having any painting skills. This may become a new mass entertainment tool just like the Prisma App that can convert photos into painting-style images.
The team also plans to expand this method to generate comics using text information. In other words, as long as the comic script is given, the system can automatically generate comics, saving a lot of time for comic artists.
