The Huake Team Released the OVIS Occluded Video Instance Segmentation Benchmark Dataset
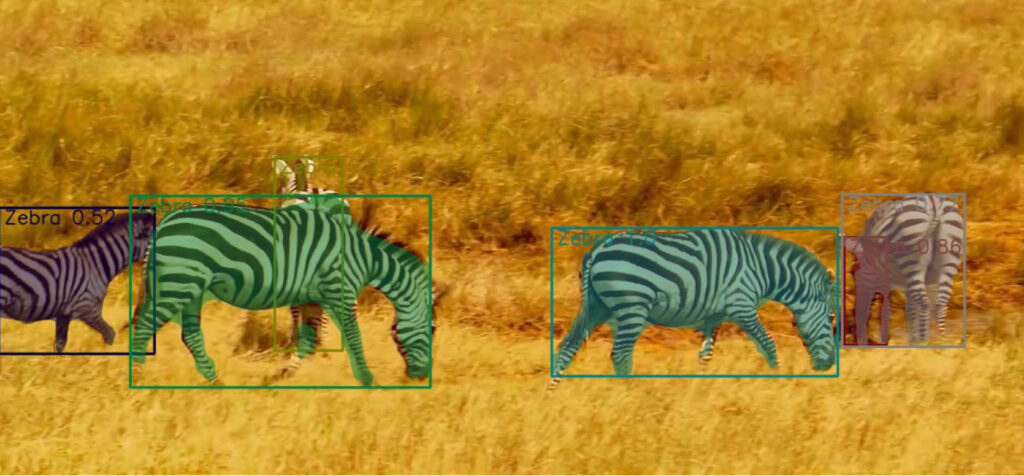
Instance segmentation can be widely used in various application scenarios. As an important research direction in the field of computer vision, it is also very difficult and challenging. In many scenarios, due to occlusion, instance segmentation becomes a difficult problem. Recently, researchers from Huazhong University, Alibaba and other institutions proposed a large-scale occluded video instance segmentation dataset OVIS to solve this problem.
In computer vision, object detection is the most core problem. In object detection, instance segmentation is considered to be the most challenging task. Instance segmentation is to segment the pixels of an object based on object detection.
Objects often block each other, which has become a headache for engineers. When we humans see an occluded object, we can identify it based on experience or association.
So, in computer vision, can we accurately identify occluded objects like humans? In the research topic of instance segmentation, solving the interference caused by occlusion has always been an important research direction.
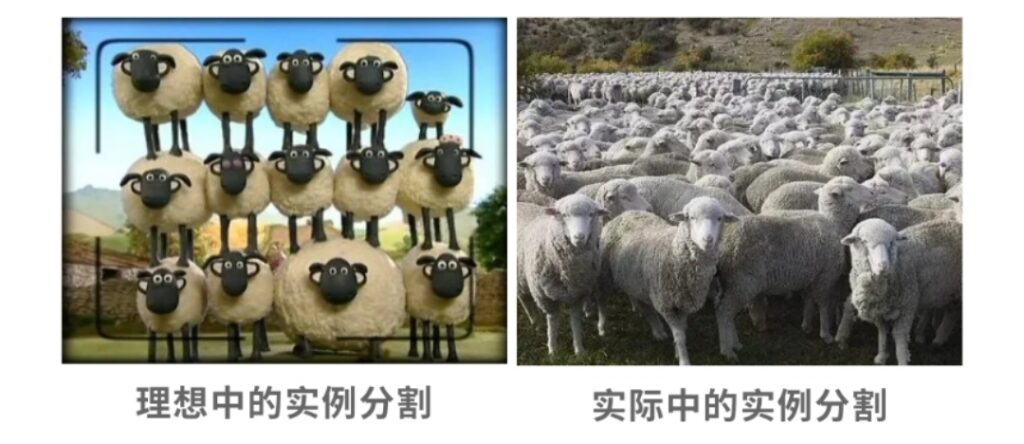
To solve this problem, teams from Huazhong University of Science and Technology, Alibaba, Cornell University, Johns Hopkins University, and Oxford University,A large-scale dataset OVIS (Occluded Video Instance Segmentation) for occluded video instance segmentation is collected, which can be used to simultaneously detect, segment and track instances in occluded scenes.
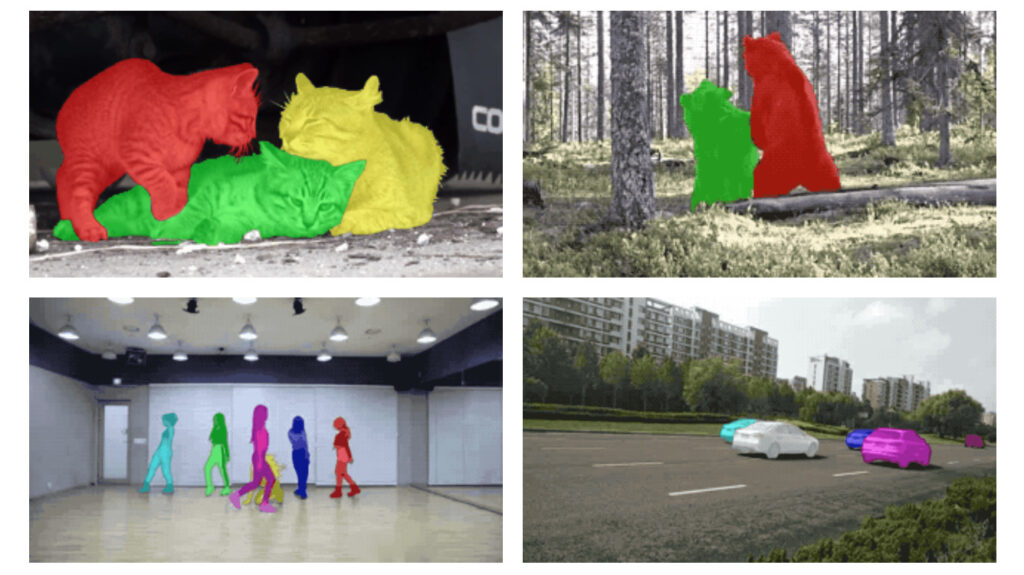
This is the second large-scale occluded video instance segmentation dataset after the Google YouTube-VIS dataset.
OVIS: Born from 901 severely obstructed videos
For everything we see, there are few objects that appear in isolation, and there are more or less occlusions. However, research shows that for the human visual system, it can still distinguish the actual boundaries of the target object under occlusion, but for the computer vision system, it becomes a big problem, that is, the occluded video instance segmentation problem.
In order to further explore and solve this problem, teams from Huazhong University of Science and Technology, Alibaba and other institutions tried to develop a better model based on the original open source instance segmentation algorithm.
To accomplish this work, the team first collected the OVIS dataset, which is specifically designed for video instance segmentation in occluded scenes.《Occluded Video Instance Segmentation》This dataset is introduced in detail.

Paper address: https://arxiv.org/pdf/2102.01558.pdf
To collect this dataset,The team collected nearly 10,000 videos in total, and finally selected 901 clips with severe occlusion, a lot of motion, and complex scenes. Each video had at least two target objects that occluded each other.
Most of the videos have a resolution of 1920×1080 and a duration between 5s and 60s. They annotated every 5 frames with high quality and finally obtained the OVIS dataset.
OVIS contains a total of 296k high-quality mask annotations of 5223 target objects.Compared to the previous Google Youtube-VIS dataset with 4883 target objects and 131k masks, OVIS obviously has more target objects and mask annotations.
However, OVIS actually uses fewer videos than YouTube-VIS, as the team’s philosophy favors longer videos for long-term tracking. The average video duration and average instance duration of OVIS are 12.77s and 10.55s, respectively, while those of YouTube-VIS are 4.63s and 4.47s, respectively.

In order to make the task of occluded video instance segmentation more challenging, the team sacrificed a certain number of video segments and annotated longer and more complex videos.
The OVIS dataset contains 25 common categories in life.As shown in the figure below, the specific categories include: people, fish, vehicles, horses, sheep, zebras, rabbits, birds, poultry, elephants, motorcycles, dogs, monkeys, boats, turtles, cats, cows, parrots, bicycles, giraffes, tigers, giant pandas, airplanes, bears, and lizards.

These categories were chosen based on the following three considerations:
- These targets are often in motion and are more likely to be severely occluded;
- They are very common in life;
- These categories have a high overlap with currently popular large-scale image instance segmentation datasets (such as MS COCO, LVIS, Pascal VOC, etc.), making it convenient for researchers to migrate models and reuse data.
Compared with other previous VIS datasets,The most notable feature of the OVIS dataset is that a large portion of objects are severely occluded due to various factors.Therefore, OVIS is an effective testbed for evaluating video instance segmentation models dealing with severe occlusions.
In order to quantify the severity of occlusion, the team proposed an indicator, mean Bounding-box Overlap Rate (mBOR), to roughly reflect the degree of occlusion. mBOR refers to the ratio of the area of the overlapping part of the bounding box in the image to the area of all bounding boxes. From the parameter comparison list, it can be seen that compared with YouTube-VIS, OVIS has more serious occlusion.
The details of the OVIS dataset are as follows:
Occluded Video Instance Segmentation
Occlusion Video Instance Segmentation Dataset
Data source:《Occluded Video Instance Segmentation》
Quantity included:5223 target objects, 296k masks
Number of types:25 types
Data format:Frame: jpg; Comment: Json
Video resolution:1920×1080 Data size:12.7 GB
Download address:https://orion.hyper.ai/datasets/14585
OVIS proposes a higher benchmark for video instance segmentation
The OVIS dataset is randomly divided into 607 training videos, 140 validation videos, and 154 test videos. The team conducted a comprehensive evaluation of five existing open source video instance segmentation algorithms on OVIS, which also served as a benchmark for the baseline performance of the OVIS dataset.
The evaluation results are shown in the following table:


Compared with YouTube0-VIS, the performance of the five algorithms, FEELVOS, IoUTracker+, MaskTrack R-CNN, SipMask and STEm-Seg, on OVIS has dropped by at least 50%.For example, the AP of SipMask dropped from 32.5 to 12.1, while that of STEm-Seg dropped from 30.6 to 14.4. These results remind researchers to pay more attention to the problem of video instance segmentation.
In addition, the team significantly improved the performance of the original algorithm by using a calibration module.The CMaskTrack R-CNN developed by it has improved the AP of the original algorithm MaskTrack R-CNN by 2.6, from 12.6 to 15.2, and CSipMask has improved the AP of SipMask by 2.9, from 12.1 to 15.0.
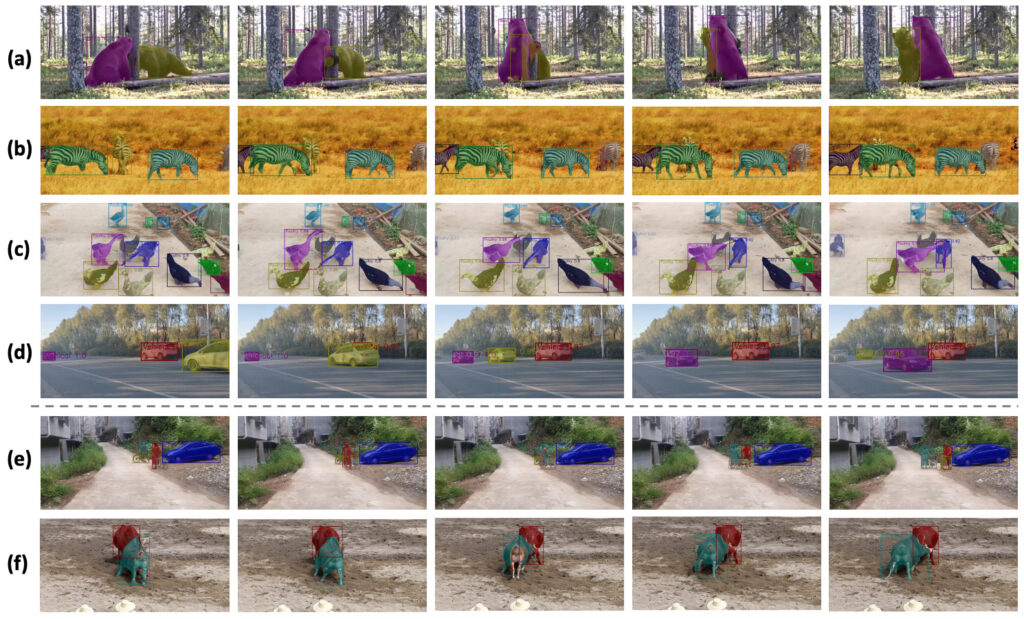
In the above figure (c), in a crowded scene with ducks, the team's method almost correctly detects and tracks all ducks, but the leftmost duck in the second frame fails to be detected. However, in the subsequent frames, the duck is re-tracked, proving that the team's model captures temporal cues well.
The team further evaluated their proposed CMaskTrack R-CNN and CSipMask algorithms on the YouTube-VIS dataset, and the results showed that they surpassed the original methods in terms of AP.
Future applications: video panorama segmentation, synthetic occlusion data
The team said that the baseline performance of commonly used video segmentation algorithms on OVIS is much lower than that on YouTube-VIS, which indicates that in the future, researchers should invest more energy in processing occluded video objects.
In addition, the team explored ways to address occlusion issues using temporal context clues, and in the future, the team will formalize the experimental track of OVIS in video object segmentation scenarios in unsupervised, semi-supervised, or interactive settings. In addition, it is also crucial to extend OVIS to video panoramic segmentation (Note: Video panoramic segmentation is to achieve semantic segmentation of the background and instance segmentation of the foreground at the same time, which is a recent new trend in the field of instance segmentation).
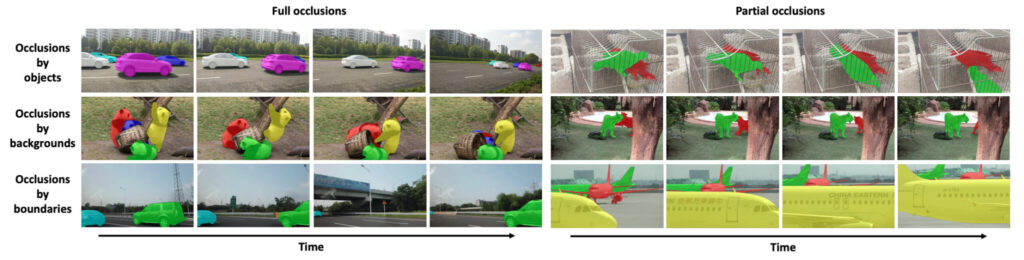
In addition, synthetic occlusion data is also a direction that the team needs to explore further. The team said that they believe that the OVIS dataset will trigger more research on understanding videos in complex and diverse scenarios.
This technology will play an important role in the separation of characters and backgrounds in film and television special effects, short videos, and live broadcasts in the future.
References:
Paper address: https://arxiv.org/pdf/2102.01558.pdf
Project official website: http://songbai.site/ovis/
Google YouTube-VIS Dataset: