Although Human Beings Do Not Share the Same Feelings of Joy and Sorrow, Sentiment Analysis Models Can Understand Them.
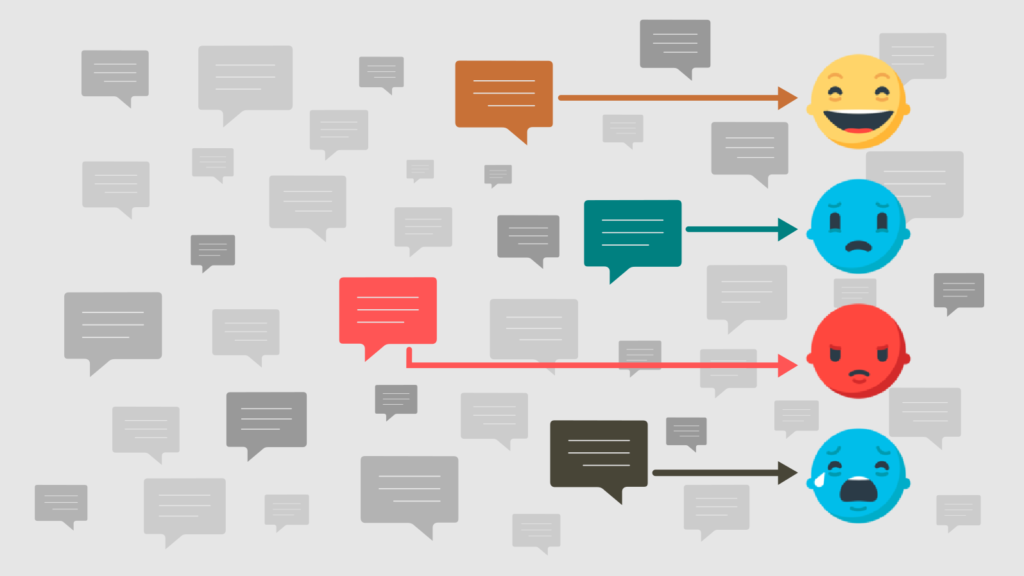
Social media has gradually become a part of people's lives today, and it has also become an important source of data for psychologists to conduct research. At the same time, researchers are also trying to use natural language processing and machine learning techniques to predict the emotional fluctuations of social media users.
The sudden outbreak of the COVID-19 pandemic last year has profoundly affected people's lives. During this special historical period, the psychology of the general public has become sensitive and fragile.
During the epidemic, people spent more time on social networks due to reduced outings and contact. Some people inevitably vented their dissatisfaction with work and life to others through the Internet. Negative emotions such as panic, anxiety, sadness, and helplessness have also increased.
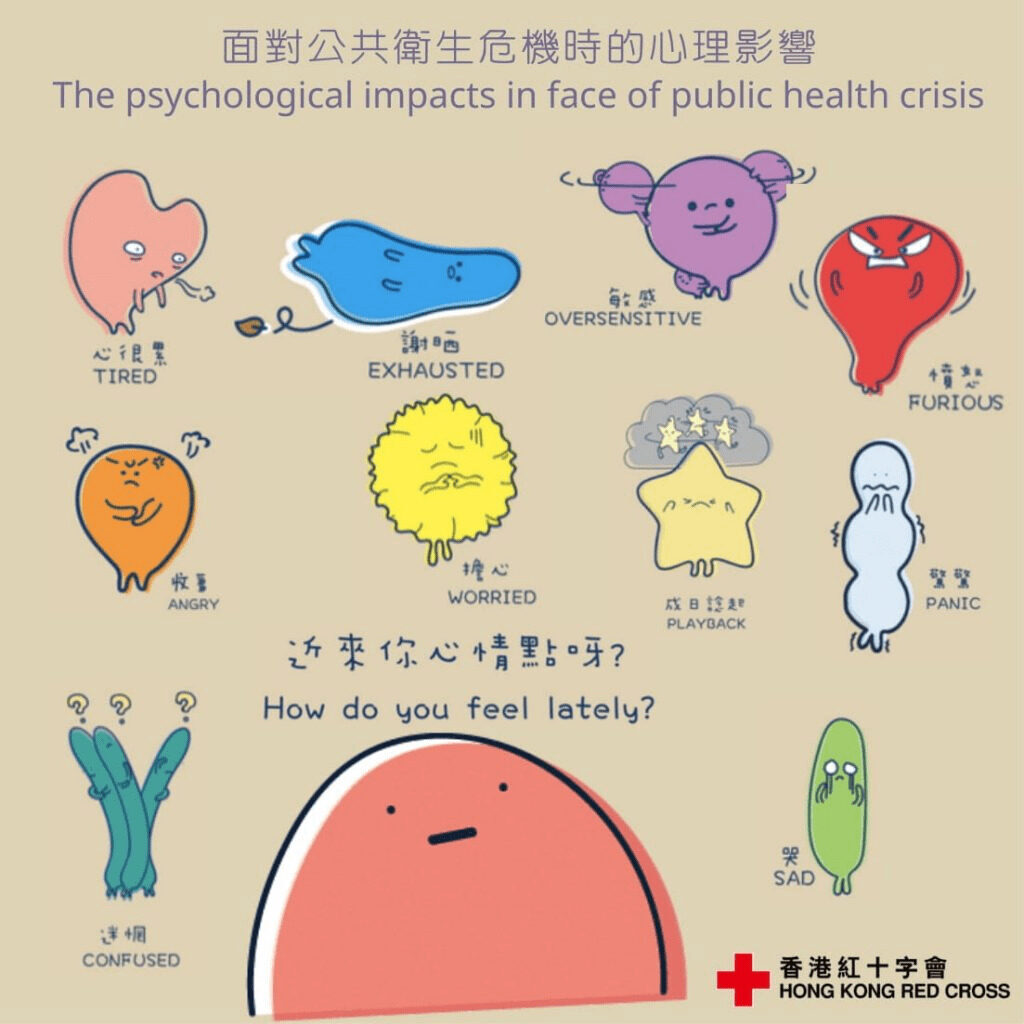
According to a survey, the average time that Internet users around the world spend on social media is 2 hours and 22 minutes per day.Social media are no longer limited to social functions, they have also become a place for many people to record their feelings and express their thoughts.
Whether it is domestic WeChat Moments, Weibo, QQ Space, etc., or foreign Twitter, Instagram, Facebook, they all carry the status of thousands of users.
For psychology researchers, these social media posts undoubtedly provide them with a considerable amount of research data.
In their latest study, researchers Johannes Eichstaedt from Stanford University and Aaron Weidman from the University of Michigan used natural language processing tools to analyze Facebook users' posts.
Research shows that machine learning models can provide insights into a person’s emotions and fluctuations through social media with an accuracy comparable to traditional psychology measurements.
Read your joys, sorrows, anger and happiness from the lines
In recent years, the large amount of information on the Internet has become an important source of data in personality science.A large body of research has shown that using social media profiles to categorize personality-related dimensions is effective.
Eichstaedt and Weidman's latest research provides a cutting-edge case for using social media big data analysis to track people's psychological states.

Sampling calibration
The authors used two basic emotional dimensions, “valence” and “arousal”, to evaluate the emotions of posts on Facebook.
Note: "Valence" and "arousal" are two dimensions of evaluating emotions in psychology. The former indicates the degree of positivity/negativity felt, distinguishing between positive and negative emotions; the latter indicates the degree of calmness/excitement.
They first had human research assistants with a background in psychology annotate 2,895 public Facebook posts from an earlier study.
The researchers rated each post on valence and arousal using a 9-point scale (for valence, 1 = "negative" and 9 = "positive", and similarly for arousal, 1 = "low" and 9 = "high").

Once these reviews were completed, the posts were used to train a machine learning model that would be able to predict which language conveyed which sentiment.
The authors then fit a series of models to these ratings data, each of which showed a clear possible link between valence and arousal.
For domestic NLP researchers, the Chinese sentiment analysis dataset is more applicable.Therefore, Super Neuro recommends a Chinese Weibo sentiment analysis dataset from the 2014 NLPCC.
The evaluation data comes from Sina Weibo. For the entire input Weibo, the task requires to determine whether the Weibo contains emotions. For Weibo containing emotions, the emotional classification output is required to be anger, disgust, fear, happiness, like, sadness, and surprise.
The dataset details are as follows:
Chinese Weibo Sentiment Analysis Dataset
Data provided:NLPCC2014
Release time: 2014
Quantity included:Hundreds of thousands of microblog texts
Data format:.xml
Data size:18 MB
Download address:https://orion.hyper.ai/datasets/14390
Model creation
The team used the Differential Language Analysis ToolKit (DLATK) to extract language features from the selected Facebook posts, and based on the relative frequency of words and phrases, retained words that were more than three times more frequent than phrases that appeared by chance. In the end, 1,439 sentence components were filtered out to predict "valence" and 675 sentence components to predict "arousal".
then,Train a ridge regression model based on the entire language feature set to predict "valence" and "arousal",And 10-fold cross validation was used (i.e., the model was built on 90% of data and then evaluated on the remaining 10%).
The cross-validation out-of-sample prediction accuracy of the model is: valence prediction accuracy is 0.63; arousal accuracy is 0.82. Compared with other standard emotion measurement methods, it is found that the model is more accurate than these alternative measurement methods.
Verification Sample
To test the model, the research team sampled 640 American users from more than 65,000 Facebook posts, with an equal number of men and women. The users were also required to meet the following conditions: they had to post more than 10 status updates for at least 14 consecutive weeks.
Ultimately, the research team collected 303,575 posts posted by these users as a validation sample.
Experimental Results
The author visualized the user's emotional evaluation, as shown in the figure below, which describes the weekly mood and arousal fluctuations of a woman (left) and a man (right), as well as the predictions of the five major personality traits.
Note: The Big Five personality traits are a structural model used to describe personality traits in modern psychology, including: extraversion, neuroticism, agreeableness, conscientiousness, and openness to experience.
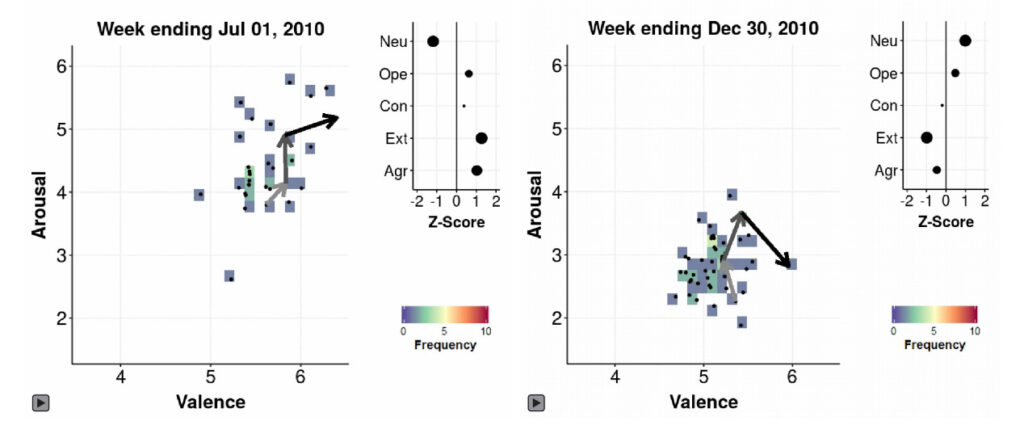
As can be seen from the figure, the female user on the left has greater emotional fluctuations, and has a higher frequency of high pleasure (Valence) and high excitement (Arousal).
In contrast, the male user on the right has less emotional fluctuations and rarely experiences high levels of pleasure or excitement.
This is also a new discovery in the team's experiment: women tend to be more optimistic and have a wider range of emotions than men.
In addition, the team's analysis also found correlations between "valence" and "arousal" values and the five major personality traits.
Model Evaluation
The Facebook users who provided the validation samples had previously voluntarily participated in a “My Personality” questionnaire, which assessed their five major personality traits.
The results showed that the machine learning model's predictions about their personalities were consistent with those made using psychological survey methods.
Defect Analysis
Of course, the author also points out the current problems with this model.
First, they used relatively active Facebook users as their sample, but they were chosen because they provided sufficiently frequent status updates, but they are unlikely to be representative of all Americans.
Secondly, different social platforms have different attributes and styles. It is still unknown whether the results obtained using Facebook posts can be replicated on different social media such as Twitter.
Therefore, these limitations and universal issues are also directions that researchers need to further explore in the future.
Social platforms have unlimited potential for psychology
Perhaps for many people, social platforms are nothing more than a place to share life, beautiful photos, and read gossip, but in fact they have great potential in psychological research.
Through data mining and machine learning, we can extract signals from huge amounts of data, identify people with depression, anxiety and other emotional disorders, and then take timely treatment measures. In this regard, there are already mature cases in China.
Huang Zhisheng, an artificial intelligence scholar at Vrije Universiteit Amsterdam, the Netherlands,In 2018, an AI program called "Shudong Rescue Team" was created to search for posts with suicidal tendencies on Weibo.Then, through "clues", the location of users with suicidal thoughts is locked, and rescue volunteers are dispatched in time to find and guide them.
Now this team of volunteers is still active in the front line of psychological counseling.

In addition, sentiment analysis technology based on social media,It can also track the psychological impact of traumatic events (such as major earthquakes, wars, the COVID-19 epidemic, etc.) on people, thereby helping government departments to effectively carry out public opinion guidance, scientific rescue and appeasement of public emotions.
As for individuals, maybe in the future we can use these tools to analyze the little emotions of our boyfriends/girlfriends, so we don’t have to guess anymore~

News Source:
https://hai.stanford.edu/blog/can-artificial-intelligence-map-our-moods