Eat Well and Healthily? AI Nutritionists Understand You Better Than Human Nutritionists
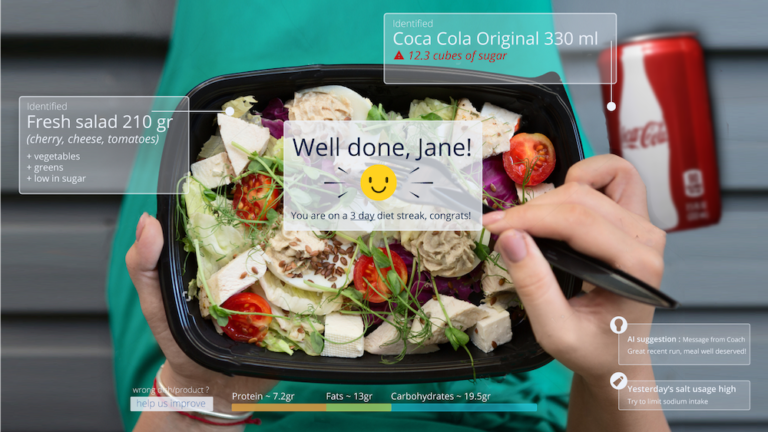
The importance of a reasonable diet and balanced nutrition is self-evident, but how to implement it is not simple. In order to get a more reasonable, healthier and more suitable recipe for people's taste, AI has also joined the team of nutritionists.
In terms of healthy eating, modern peopleHealth care, expert recommendations, the most complete recipesThese keywords have a passion beyond imagination.
Fitness people pursue muscle gain and stick to a low-carb, high-ketone diet; beauty-conscious people pursue quick weight loss, and intermittent fasting and the Copenhagen diet are the most popular; postoperative patients and pregnant women are brainwashed by the millet porridge and pig's trotter soup that are passed down by word of mouth; young people who yearn for a green life and Buddhists advocate vegetarianism, and try their best to maintain physical and mental health with limited choices.
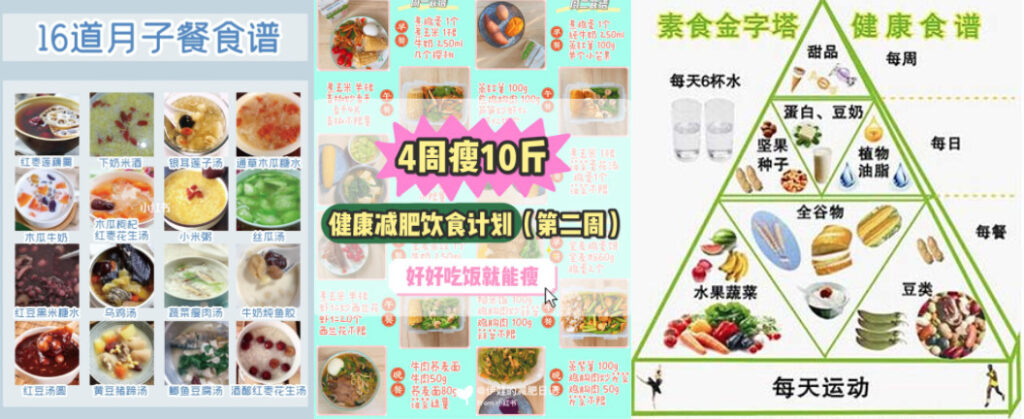
Faced with a wealth of choices, what is the right combination to choose? Some researchers are using algorithms and big data to recommend healthier and more nutritious recipes for everyone.
AI learns to “see people’s behavior and serve them the right dishes”
The biggest problem with the universal diet mentioned above is that everyone has different physiques, tastes, regional resources, and consumption levels.
Some bloggers' high-quality recipes often use salmon and avocado, ingredients that are expensive and difficult to buy in some areas, which makes many people stay away.
In order to make personalized dietary recommendations for each individual, many researchers have also introduced artificial intelligence technology.
Researchers from Rensselaer Polytechnic University and IBM Research recently developed a personalized food recommendation system, pFoodReQ, that can recommend tailored recipes based on individual user preferences and dietary needs.

Paper address: https://arxiv.org/pdf/2101.01775.pdf
The authors point out in the paper that existing dietary recommendation methods generally have three main shortcomings:
- Not understanding the user’s exact requirements;
- Failure to consider the critical factors of allergies and nutritional needs;
- There is no custom recipe based on abundant food options.
In this study, the research team proposed personalized food recommendations and regarded them as answers to restricted questions on the food knowledge graph (KG), thus trying to solve the above problems in a unified way.
The team proposed a personalized food recommendation framework based on knowledge base question answering KBQA (Knowledge Base Question Answering), which makes personalized food recommendations through question and answering.
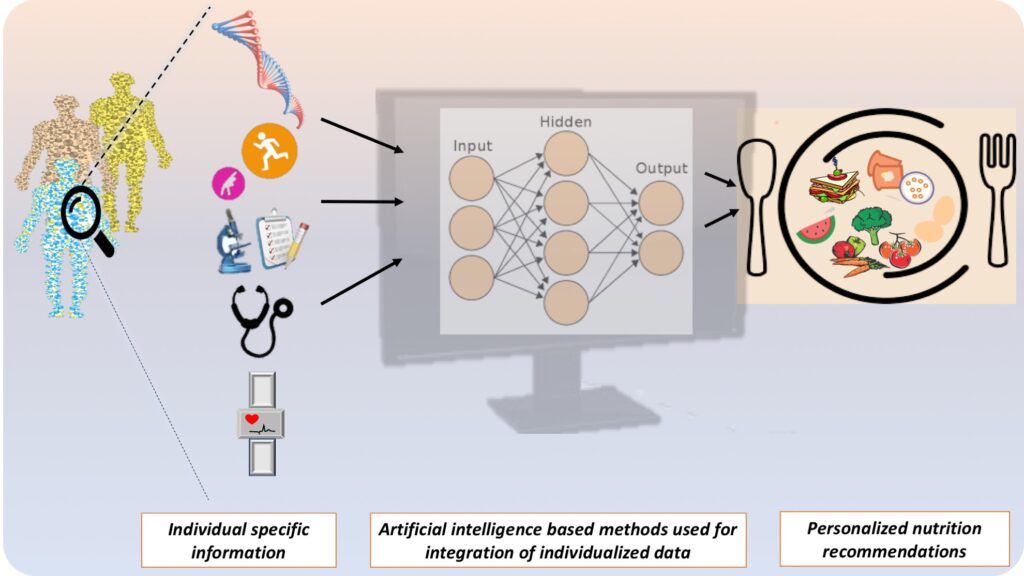
Specifically, the pFoodReQ system will respond to users’ questions, such as "What's a good breakfast that includes bread?", and then retrieve all recipes that meet this query condition from the KG. Then the ingredients in these recipes are scored for suitability.Finally, we recommend a few recipes with the highest ratings.
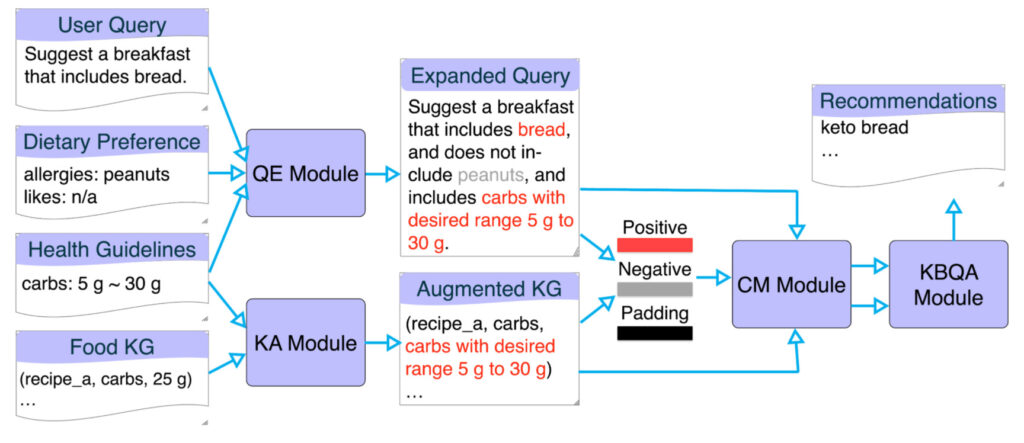
Finally, the verification experimental results showed that the method they proposed was significantly better than the non-personalized method and could recommend more relevant and healthier recipes.
There are thousands of recipes, AI only recommends dishes that are suitable for you
Overall, the team built the systemA total of four steps were involved: creating a data set, generating benchmark questions, compiling health guidelines, and training the system.
Step 1: Recipe Dataset Creation
The team created a benchmark QA dataset based on the extensive food knowledge graph FoodKG (FoodKG integrates recipes, food and nutrition data) (not yet public), which contains more than 1 million recipes, 7.7 million nutrition records, and 7.3 million foods, along with their ingredients and nutritional facts.And refer to the lifestyle guidelines recommended by the ADA American Diabetes Association.

Dataset download: https://orion.hyper.ai/datasets/5615
According to the authors, this is the first personalized food recommendation dataset related to ingredients, nutrition, and recipes that supports question-answering systems.
Each example in the dataset consists of a user query, dietary preferences, health guidelines relevant to the user, and a basic answer (i.e., recipe recommendation).
Step 2: Generate Benchmark Problem
In order to get questions that reflect people's true eating habits,The author collected more than 200 recipes and diabetes-related questions on the social media Reddit.A total of 156 posts were found asking for recipes, and they mainly focused on four types of questions:
- which ingredients are edible;
- Which ingredients are not edible?
- "Low carbohydrate" or "high protein", which ones are recommended?
- "Italian style" or "Mediterranean style", what are your recommendations?
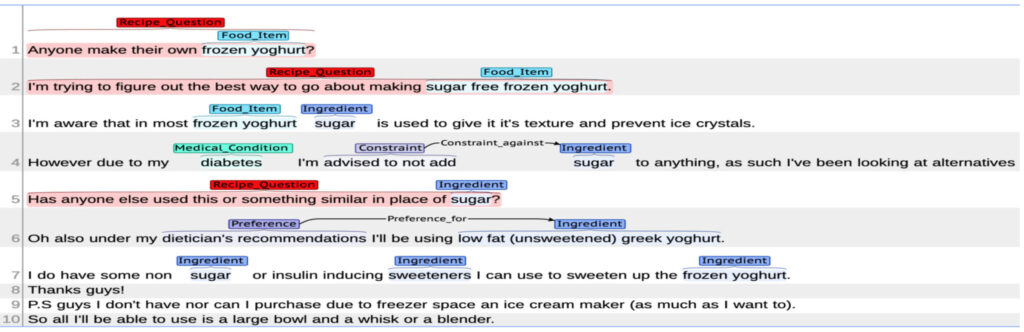
Based on the way the questions were asked in these posts, the team summarized 56 different templates and generated some benchmark questions based on them.
Step 3: Compile a health guide
Once the preliminary preparations are complete, you can make healthy diet recommendations.
The team selected some food-related guidelines from the ADA lifestyle guidelines, which involve nutrients and trace elements, and used them as additional food recommendations. Therefore, its systematic recommendations are all healthy recipes that meet the health guidelines.
Since these criteria are in natural language, they are converted into a structured representation (e.g., a hash table storing key-value pairs).

Step 4: Train the Personalized System
In order to achieve personalization, the team also solved the problems of query expansion and allergy query. The author believes thatAn effective food recommendation system should respect individual needs within dietary preferences and health guidelines.Therefore, for user queries, further expansion is performed.
For example, the user asks the system a question Please recommend a breakfast that includes bread.The system will understand the user's dietary preferences based on their previous queries, dietary preferences, and dietary history logs, and automatically expand the single query into a query with additional personalized needs.
The expanded query becomes:"A high-quality breakfast that includes bread, no peanuts, and contains 5g to 30g of carbohydrates is recommended."
Because of this, the system can give different recipe suggestions when faced with the same question asked by different users.
Experimental results: better than other models
The team conducted a human evaluation of the food recommendations by providing 8 raters with a random test of 50 questions, along with a user persona including ingredient preferences (likes and dislikes) and applicable nutritional guidelines.
For each question, the four models, BAMnet, P-BOW, P-MatchNN and pFoodReQ, were fed in random order and the answers were obtained. Each answer contained the top three recipes (if more than three recipes were retrieved), the ingredient list and the nutritional value.

However, this personalized diet recommendation system is only the first step in the team's research. The author said, "There are still many challenges in the future. We need more complex answer benchmarks to handle implicit user intentions and various special cases."
AI nutritionists are more needed by Chinese people
The importance of a healthy diet is self-evident, but our current eating habits are still very unhealthy.According to a research report released by The Lancet, China ranks 140th among 195 countries in the world.
To fundamentally change unhealthy eating habits, it is far more than just recommending recipes. Can the intervention of AI bring us more possibilities? Here are some of the advantages of AI nutritionists that we have summarized:
Let down your mental defenses when facing machine recommendations
When facing human nutritionists and fitness coaches, many people may not reveal their true eating habits and consumption levels due to face or privacy issues.
But it’s different if you use an AI nutritionist. You can let go of your psychological burden, tell the AI your real needs, and then let it search for the one that interests you and is nutritious from thousands of recipes.
Tolerate cheat day and dynamically adjust the menu
Nowadays, the Internet is flooded with popular science articles on nutritional combinations and balanced diets, but for readers, they are too difficult to implement and compliance is low.
We will always encounter situations where we cannot fully execute the recipe or go out for social events. AI can make timely adjustments to subsequent recipes to adapt to these changes.
Fill the gap in nutritionists and improve health awareness
Although people's health awareness is rising, the profession of nutritionist in our country faces a huge gap.
According to a survey, in Japan, there is one nutritionist for every 300 people; in the United States, there is one nutritionist for every 4,000 people;In China, there is only one nutritionist for every 400,000 people.
If AI has complete knowledge of nutrition and health, then everyone can have a personal nutritionist accompanying them 24 hours a day who can provide dietary guidance anytime and anywhere.
At that time, the difficult question of "what to eat today" can be left to AI!
