Zhejiang University Team Publishes a New Method for 3D View Synthesis, Which Is Far Better Than NeRF and NV
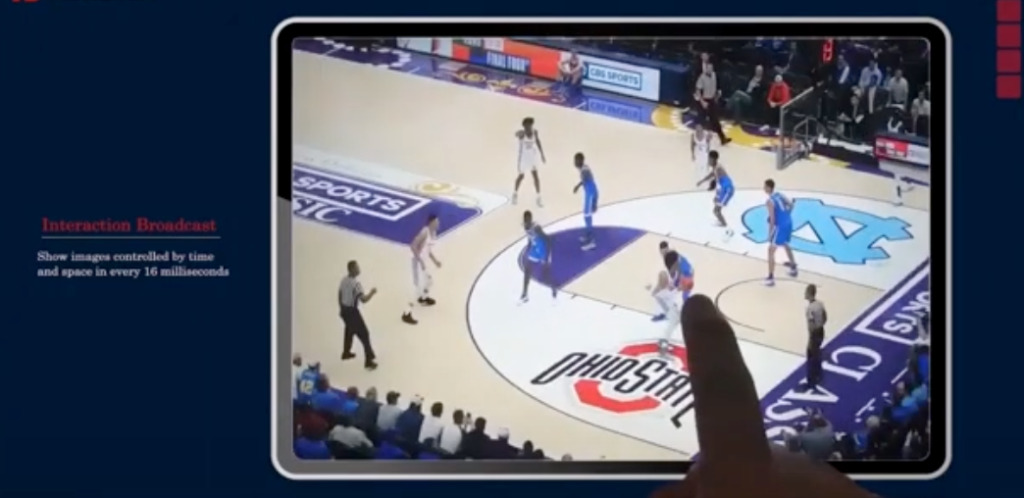
With just a few videos from different angles, the entire 360° image of the human body can be constructed without blind spots. It has to be said that AI's ability to make up for the past is getting stronger and stronger. Such tools may bring new breakthroughs to the film and television industry, sports program presentation, etc. in the future.
In the future, the way we watch movies, football games, concerts, etc. may be completely changed by "free-viewpoint video".
You may not know what "free viewpoint video" is, but you should have experienced VR, AR videos, or played 3D games. These all fall into the category of free viewpoint video, and their characteristics are:It can be viewed from any angle, providing a fully immersive experience.

How can such a video be shot? Generally speaking, the traditional method requires multiple cameras to shoot from different angles, and then combine the videos from all angles together.
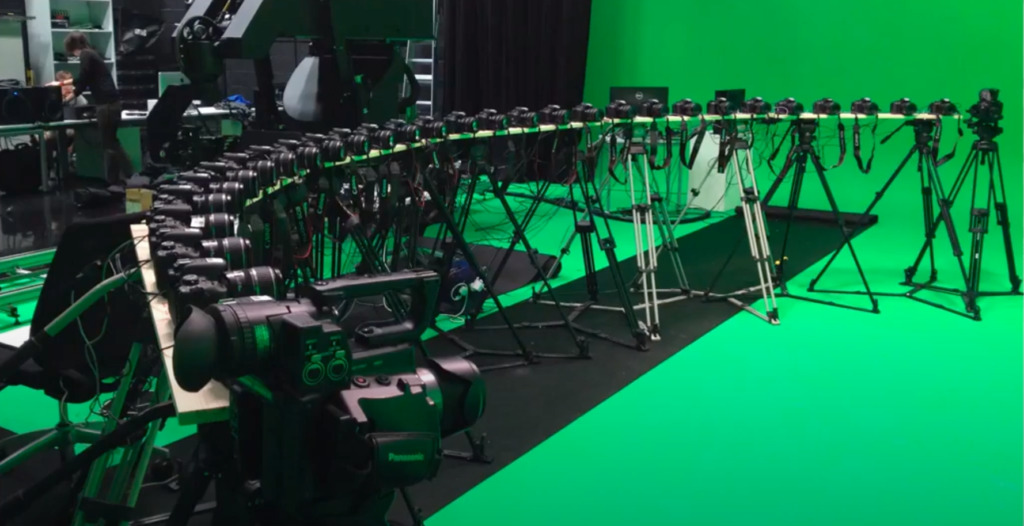
However, this method relies on multiple cameras, which is not only expensive but also limited by the environment of the shooting site.
There is another way to get rid of these limitations.By simply inputting a few shots of the human body taken from different angles, a new 360° 3D view of the human body can be synthesized.This is the latest result recently published by researchers from Zhejiang University.
At the end of December, the team published a new paper on arxiv"Neural Body: Implicit Neural Representations with Structured Latent Codes for Novel View Synthesis of Dynamic Humans", proposed a new human body representation Neural Body, using sparse multi-view videos to synthesize new dynamic 3D human body views. Experimental verification shows that this method is superior to other previous methods.
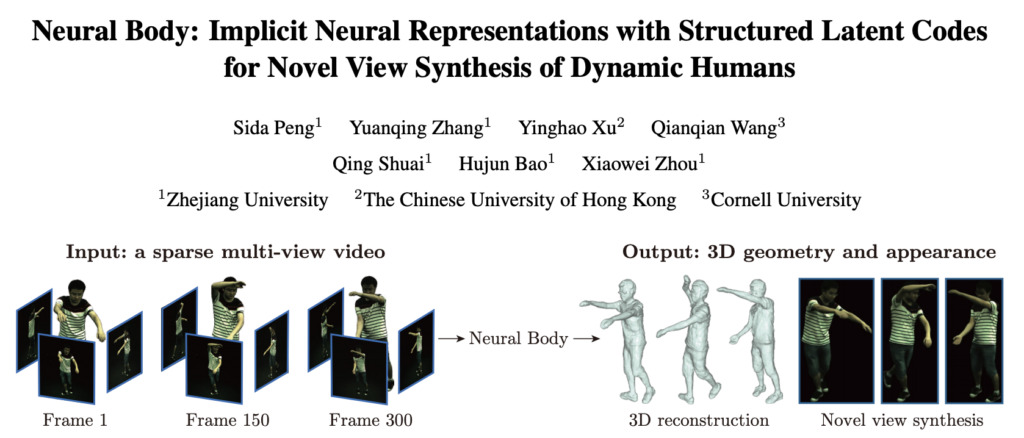
Paper address: https://arxiv.org/pdf/2012.15838.pdf
It is worth mentioning that the seven authors behind the paper all studied or graduated from Zhejiang University, and are from the State Key Laboratory of Computer-Aided Design and Graphics of Zhejiang University.Among them, Hujun Bao and Xiaowei Zhou are both professors of the laboratory. After graduating from undergraduate studies, Yinghao Xu and Qianqian Wang pursued their doctoral degrees at the Chinese University of Hong Kong and Cornell University respectively.
Even with a small amount of material, you can still generate high-quality 3D views
At present, whether it is a film or television program or a sports event, what we see is the picture taken by a single camera. If we can get "free perspective video" and see whatever we want, it will definitely be an experience like God's perspective.
In fact, AI has also been studying this problem in recent years, and has produced view synthesis solutions such as NeRF and Neural Volumes (NV for short).
However, existing studies have shown that learning implicit neural representations of 3D scenes can achieve good view synthesis quality under dense input view conditions. However, if the views are highly sparse, representation learning will be ill-posed.
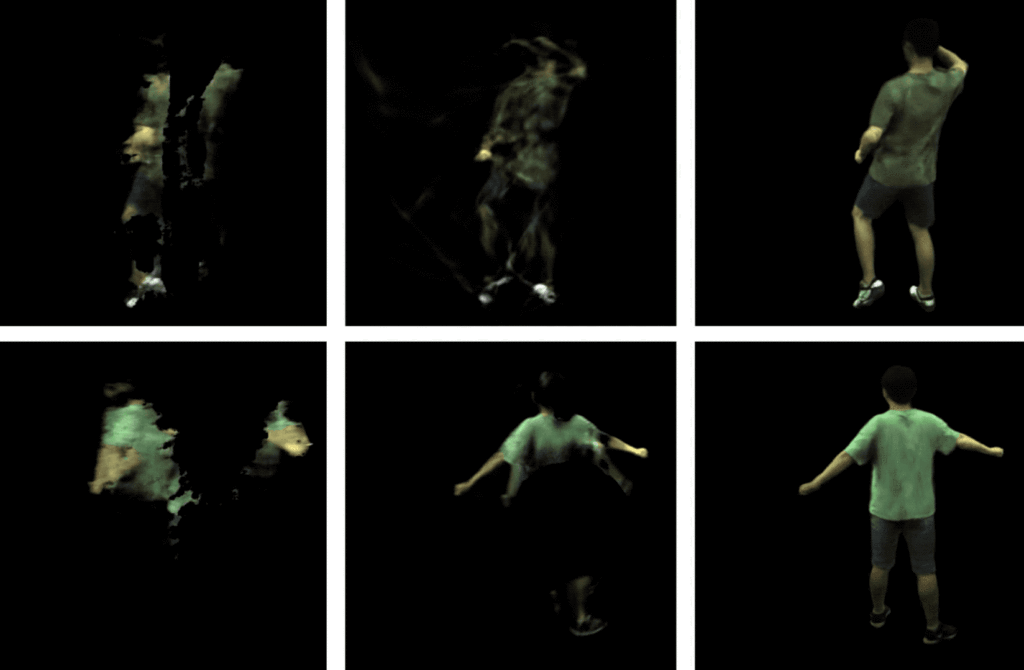
Therefore, in order to solve this ill-posed problem, a research team from Zhejiang University, the Chinese University of Hong Kong and Cornell University proposed the key idea of integrating observation results on video frames.
The team’s latest research result proposed the Neural Body.This is a new representation of the human body that assumes that neural representations learned over different frames share the same set of latent codes anchored on a deformable grid so that observations across frames can be naturally integrated.The deformable mesh also provides geometric guidance to the network to learn 3D representations more effectively.

The researchers conducted experiments on a newly collected multi-view dataset and showed that their method has a large advantage over previous methods in terms of view synthesis quality.
In a demo, the team demonstrated the ability of their method to reconstruct moving figures from monocular videos of people performing various actions.
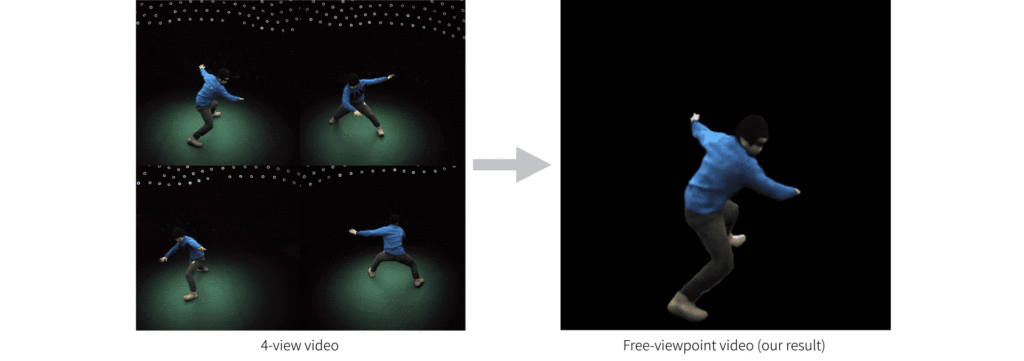
This method greatly reduces the cost of free-viewpoint video synthesis, at least, saving the cost of the camera, and therefore has a wider applicability.
Get Neural Body in 5 Steps
1. Structured latent code
In order to control the spatial position and human posture of the latent codes, the team anchored these latent codes to a deformable human model (SMPL). SMPL is a skin vertex-based model, which is defined as shape parameters, pose parameters, and rigid body transformation functions relative to the SMPL coordinate system.
Latent codes are used together with neural networks to represent the local geometry and appearance of a person. Anchoring these codes on a deformable model enables the representation of a dynamic person. With the representation of dynamic people, the team built a latent variable model that maps the same set of latent codes into implicit domains of density and color across different frames, naturally integrating observations.
2. Code Proliferation
Since the structured latent code is sparse in 3D space, directly interpolating the latent code will result in zero vectors for most 3D points. To solve this problem, the team diffused the latent code defined on the surface into the nearby 3D space.
Since the diffusion of the code should not be affected by the position and orientation of the person in the world coordinate system, they transform the position of the code into the SMPL coordinate system.
Code diffusion also aggregates the global and local information of structured latent codes, which helps to learn the implicit domain.
3. Density and Color Regression
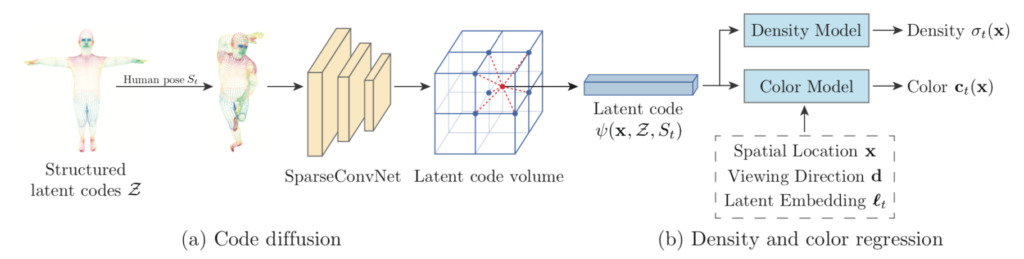
The research team found that time-varying factors affect the appearance of the human body, such as secondary lighting and self-shadowing. Inspired by the automatic decoder, the team assigned a latent embedding frame t to each video frame to encode the time-varying factors.
4. Volume Rendering
Under a given viewpoint, the team used classic volume rendering (also known as stereo rendering) technology to render the Neural Body into a two-dimensional image.
Then, the scene boundaries are estimated based on the SMPL model, and Neural Body predicts the volume density and color of these points.
Based on volume rendering, the model is optimized by comparing the rendered image with the observed image.
5. Training
Compared with frame-based reconstruction methods, this method utilizes all images in the video to optimize the model and has more information to recover the 3D structure.
In addition, the team used the Adam optimizer to train Neural Body. The training was performed on four 2080 Ti GPUs. For a four-view video with a total of 300 frames, the training usually takes about 14 hours.
After the above five steps, Neural Body is able to achieve free-viewpoint video synthesis based on a small number of views, and compared with other methods, the effect is significantly better than the former.
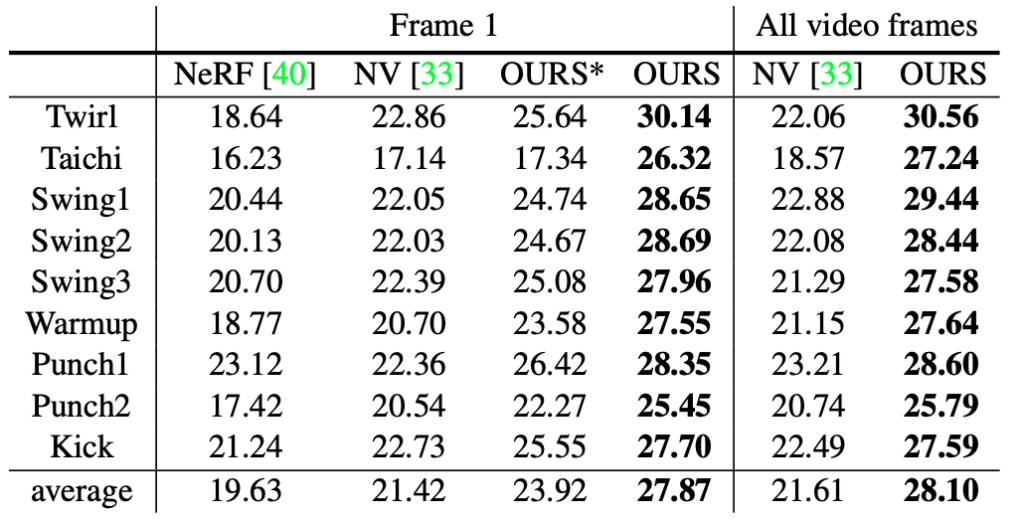
Note: "OURS*" and "OURS" represent the results of training on only one frame of video and four frames of video respectively)
AI's brain-filling technology makes it easier to achieve 3D effects, and its applications are not limited to the film and television industry and live sports events. For game developers, fitness instructors, 3D advertising providers, etc., it is a tool that can greatly improve work efficiency and effectiveness.

Project homepage: