MIT Updates the Largest Natural Disaster Image Dataset, Covering 19 Disaster Events
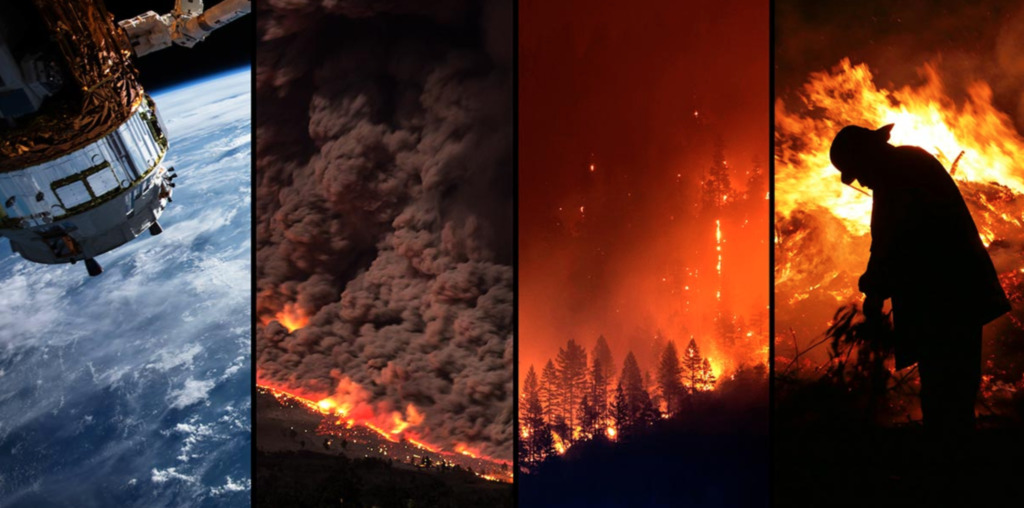
MIT recently released a natural disaster image dataset in a paper presented at ECCV 2020. This is the largest and highest quality natural disaster satellite image dataset to date.
2020 has been a year of disasters. The outbreak at the beginning of the year, the floods in the south in the summer, the recent wildfires in California, USA...
Natural disasters such as floods, wildfires, and earthquakes always threaten people's lives and property.If we can detect some subtle changes in a timely and rapid manner, we can better formulate corresponding rescue plans and allocate resources more reasonably, which will also help report related news.
So, MIT engineering master's student Ethan Weber and collaborator Hassan Kan, in a new paper, Building Disaster Damage Assessment in Satellite Imagery with Multi-Temporal FusionA deep learning model is proposed inSatellite imagery of damaged areas can be assessed faster and more accurately, buying more time for first responders and minimizing damage.
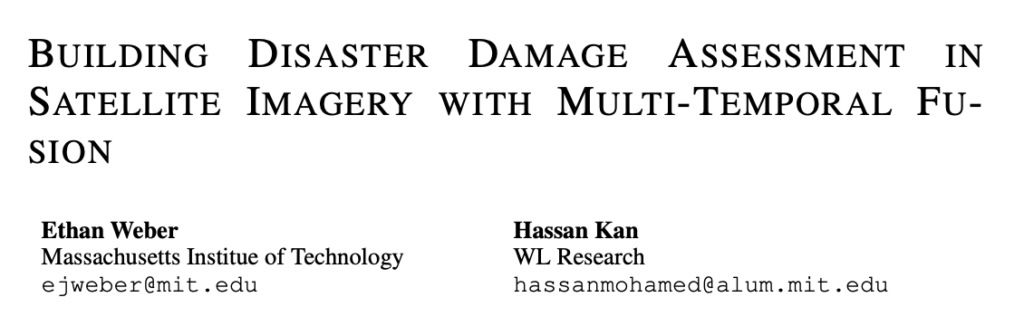
At the same time, they also released a new satellite imagery dataset for damage assessment, which takes research on image event detection a step further and enables researchers to more accurately locate and quantify losses.
Racing against time with AI: Accelerating disaster assessment
For natural disasters, it is crucial for on-site emergency teams to reduce reaction time, respond quickly and take action quickly to reduce losses and save lives.It is also important for emergency responders to understand the exact location and severity of the damage in order to better deploy resources in the affected areas.
Currently, emergency personnel usually assess the extent of disaster damage by manually observing satellite images, but the assessment process may take several hours, which is extremely unfavorable for rescue work.

Ethan Weber's contribution to this study is thatCreate tools that automatically analyze images, reduce image analysis time, and win the race against time.
In addition, their research can achieve better performance by independently providing pre-disaster and post-disaster images through CNN (convolutional neural network) with shared weights.
They also proposed a new computer vision model that can detect events in images posted on social media platforms such as Twitter and Flickr.
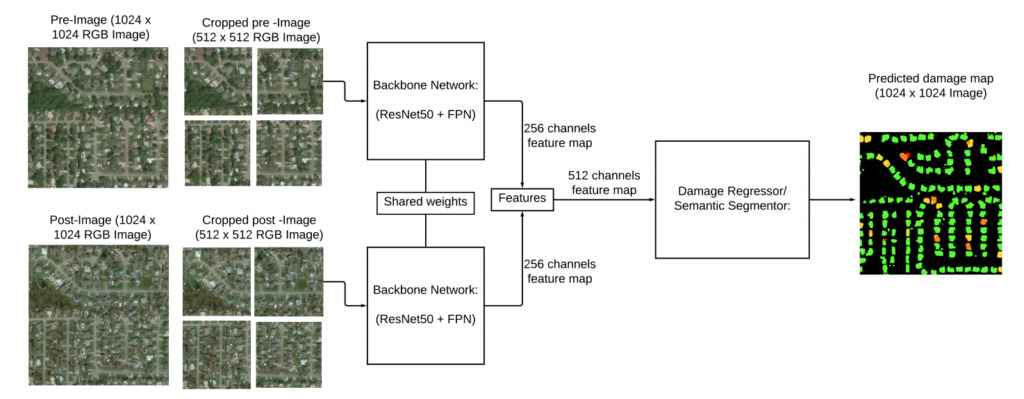
22068 images labeled with 19 natural disasters
In addition to proposing a new model,The research team also released a new event dataset: the xBD dataset.
The dataset contains 22,068 images labeled with 19 different events.Including earthquakes, floods, wildfires, volcanic eruptions and car accidents. These images include pre-disaster and post-disaster images, and the images can be used to construct two tasks: positioning and damage assessment.
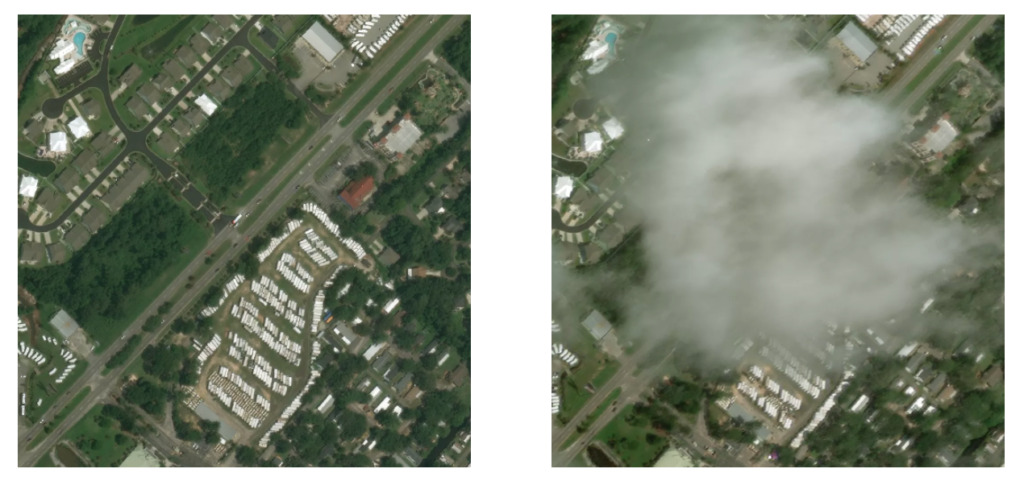
According to the introduction,The xBD dataset is the first building damage assessment dataset to date and is one of the largest and highest quality public datasets of annotated high-resolution satellite imagery.Its basic information is as follows:
xBD Dataset
Publishing Agency: MIT
Quantity included:22068 images
Data format:png
Data size:31.2GB
Update time:August 2020
Download address:https://orion.hyper.ai/datasets/13272
The images have a resolution of 1024×1024, with identifiers for each building, which remain consistent across pre- and post-disaster images.
However, the researchers found that the resolution of buildings was often too small for the model to accurately draw building boundaries. To this end, they trained and ran the model on four 512×512 images to form upper left, upper right, lower left, and lower right quadrants.
Based on these pre- and post-disaster data, damage assessment can be defined as single-temporal and multi-temporal tasks.In the single-temporal setting, only post-disaster images are fed into the model, which must predict the level of damage at each pixel. In the multi-temporal setting, both pre- and post-disaster images are fed into the model, which must predict the extent of damage on the post-disaster images.

Where does the dataset come from?
The team says the new dataset aims to fill a gap in this area.Existing datasets are limited in both the number of images and the diversity of event categories.
The authors also explain how to create a dataset, how to create a model to detect events in images, and how to filter events in noisy social media data.
As part of their work, they filtered 40 million Flickr images to look for disaster events.Other work is filtering images posted to Twitter during earthquakes, floods and other natural disasters.
For example, the team filtered tweets related to natural disasters to specific events and validated the process by correlating tweet frequencies with a database provided by the National Oceanic and Atmospheric Administration (NOAA).
“I’m excited about the potential for further research into detecting events in images with this dataset, and it’s been very effective in sparking interest in the computer vision community,” said Ethan Weber.
He also said social media and satellite imagery are forms of data that can help with emergency response.Social media provides on-the-ground observations, while satellite imagery provides expansive insights, such as identifying which areas are most affected by wildfires.
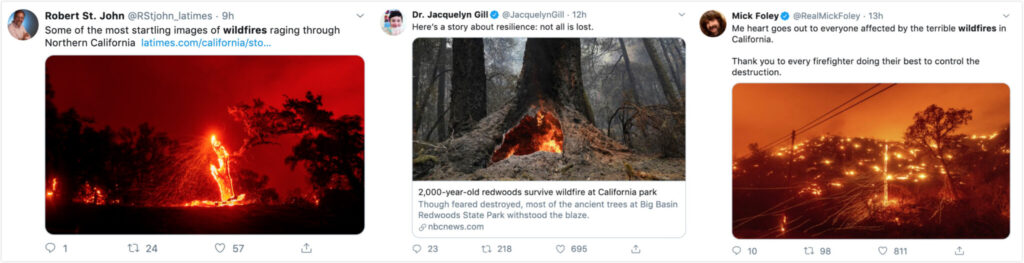
It is in recognition of this interconnectedness that Ethan Weber and his fellow alumni have collaborated to produce outstanding work in damage assessment.
“Now that we have the data, we are interested in locating and quantifying the damage,” said Ethan Weber. “We are working with emergency response organizations to stay focused and conduct research that has real-world benefits.”
accesshttps://orion.hyper.ai/datasets/13127Or click to read the original text to download the dataset at high speed.
-- over--