The Hottest ECCV in History Has Opened, and These Papers Are so Interesting
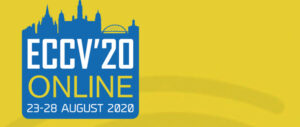
ECCV 2020, one of the three top international conferences in the field of computer vision, was held online from August 23 to 27. This year, ECCV accepted a total of 1,361 papers. We have selected 15 of the most popular papers to share with readers.
Due to the impact of the epidemic, this year's ECCV 2020, like other top conferences, moved from offline to online and kicked off on August 23.

ECCV, the full name is European Conference on Computer Vision (European International Conference on Computer Vision),It is one of the three top international conferences in computer vision (the other two are CVPR and ICCV), held every two years.
Although this year's epidemic has disrupted many people's plans, everyone's enthusiasm for scientific research and paper submission remains unabated. According to statistics,ECCV 2020 received a total of 5,025 valid submissions, more than twice the number of submissions in the previous session (2018), and is therefore considered the "hottest ECCV in history."
Finally, 1,361 papers were accepted for publication, with an acceptance rate of 27%.Among the accepted papers, oral papers accounted for 104, accounting for 2% of the total number of valid submissions, and spotlight papers accounted for 161, accounting for about 3%. The rest of the papers were posters.
Pose estimation, 3D point cloud, excellent papers list
What exciting research results has this grand event in the field of computer vision brought us this year?
We have selected 15 papers from the selected papers, covering multiple directions such as 3D object detection, pose estimation, image classification, and face recognition.
Pedestrian Re-identification "Please Don't Disturb Me: Pedestrian Re-identification Under Interference of Other Pedestrians"

unit:Huazhong University of Science and Technology, Sun Yat-sen University, Tencent Youtu Lab
summary:
Traditional person re-identification assumes that the cropped image contains only a single person. However, in crowded scenes, off-the-shelf detectors may generate bounding boxes for multiple people, where background pedestrians account for a large proportion or there are human occlusions.
The features extracted from these images with pedestrian interference may contain interference information, which will lead to wrong retrieval results.
To solve this problem, this paper proposes a new deep network (PISNet). PISNet first uses the Query image-guided attention module to enhance the features of the target in the image.
In addition, we propose a reverse attention module and a multi-person separation loss function to promote the attention module to suppress the interference of other pedestrians.Our method is evaluated on two new pedestrian interference datasets and the results show that it outperforms the state-of-the-art Re-ID methods.
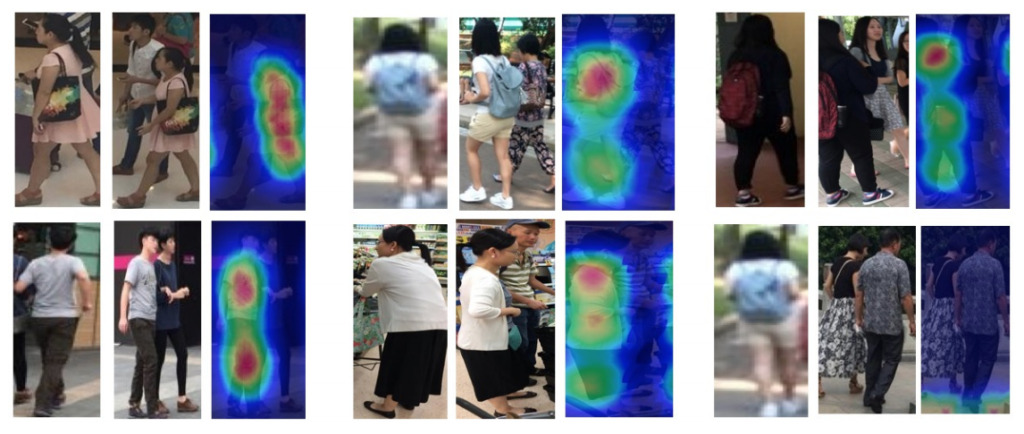
Pose Estimation "3D Pose Estimation of Multiple People in Crowded Scenes via Multi-Viewpoint Geometry"

unit:Johns Hopkins University, National University of Singapore
summary:
Extremal constraints are the core problem of feature matching and depth estimation in current multi-machine 3D human pose estimation methods. Although the formulation performs satisfactorily in sparse crowd scenes, its effectiveness is often challenged in dense crowd scenes, mainly due to ambiguity from two sources.
The first is the mismatch of human joints due to the simple clues provided by the Euclidean distance between joints and epipolar lines. The second is the lack of robustness due to naively minimizing the problem as least squares.
In this article,We depart from the multi-person 3D pose estimation formulation and reformulate it as crowd pose estimation.Our approach consists of two key components: a graphical model for fast cross-view matching and a maximum a posteriori (MAP) estimator for 3D human pose reconstruction. We demonstrate the effectiveness and superiority of our approach on four benchmark datasets.
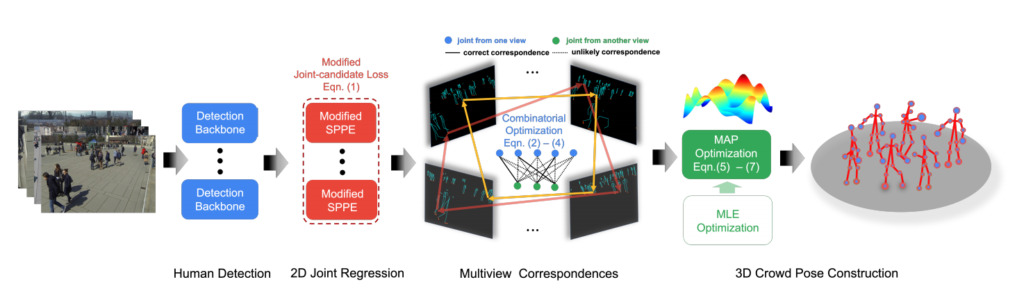
Describing images 《Natural language description generation via scene graph decomposition》
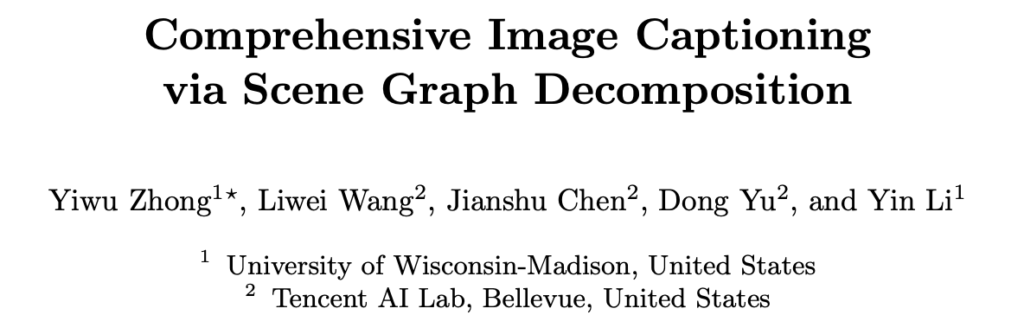
unit:Tencent AI Lab, University of Wisconsin-Madison
summary:
This paper proposes a natural language description generation method based on scene graph decomposition.
Using natural language to describe images is a challenging task. This paper reviews the image scene graph expression and proposes a method for generating natural language descriptions of images based on scene graph decomposition. The core of this method is to decompose the scene graph corresponding to an image into multiple subgraphs, where each subgraph corresponds to describing a part of the content or a part of the area of the image.By selecting important subgraphs through a neural network to generate a complete sentence describing the image, this method can generate accurate, diverse, and controllable natural language descriptions.The researchers also conducted extensive experiments, and the results demonstrated the advantages of this new model.
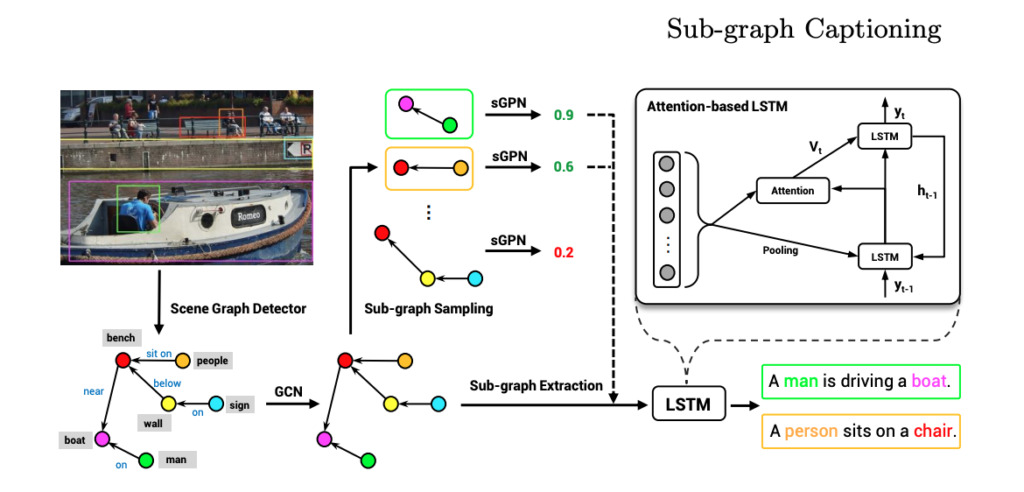
3D Point Clouds 《Quaternion Equivariant Capsule Networks for 3D Point Clouds》
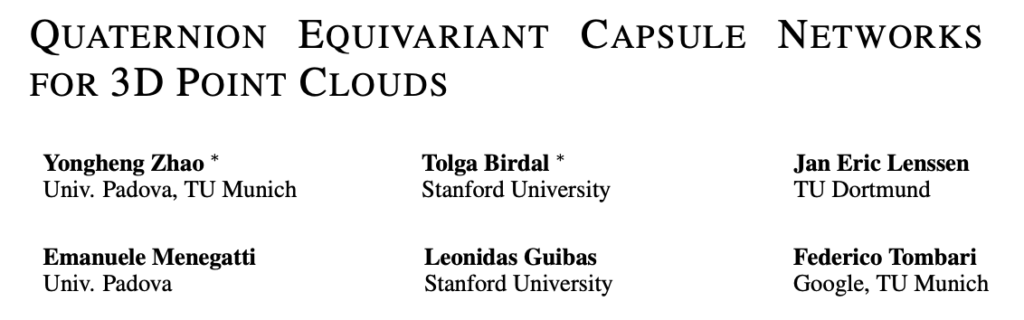
unit:Stanford University, Technical University of Dortmund, University of Padova
summary:
We propose a 3D capsule architecture for processing point clouds that is equivalent to SO(3) groups of rotations, translations, and permutations of an unordered input set.
The network operates on a sparse set of local reference frames computed from the input point cloud. The network is end-to-end robust via a novel 3D quaternion group capsule layer that includes an equivariance dynamic routing procedure.
Capsule layers enable us to disentangle geometry from pose, paving the way for more informative and structured latent spaces.In doing so, we theoretically link the dynamic routing process between capsules to the well-known Weiszfeld algorithm for solving the iteratively reweighted least squares (IRLS) problem with provable convergence properties, thus achieving robust pose estimation between capsule layers.
Thanks to sparse equivariant quaternion capsules, our architecture allows for joint object classification and orientation estimation, which we empirically validate on common benchmark datasets.
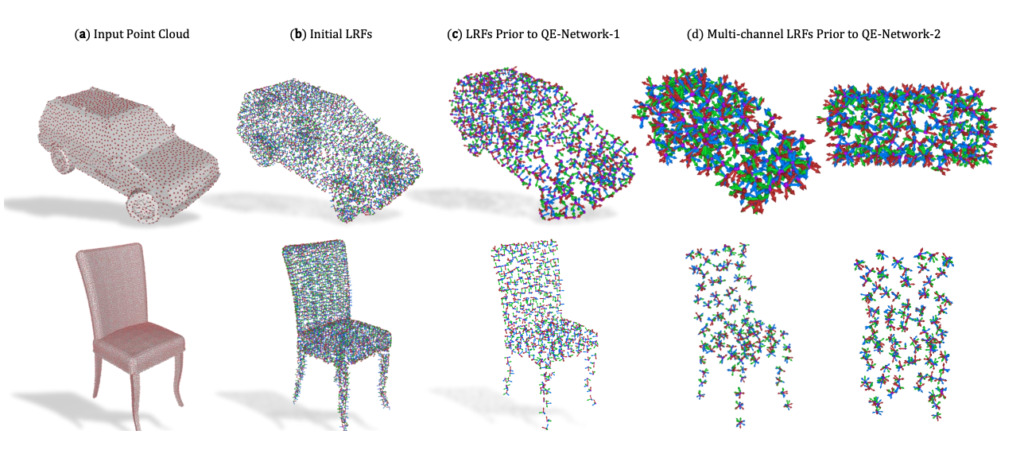
Face Recognition 《Explainable Face Recognition》

unit:Systems & Technology Research, Visym Labs
summary:
Explainable face recognition (XFR for short) is the problem of explaining the matching results returned by a face matcher.This provides insight into why a detector matches one identity and not another.Understanding this principle can help people trust and explain facial recognition.
In this paper, we provide the first comprehensive benchmark and baseline evaluation of XFR. We define a new evaluation scheme, called the "inpainting game", which is a curated set of 3648 triplets (probe, mate, nonmate) from 95 subjects, by synthetically inpainting selected facial features (such as nose, eyebrows or mouth) to create a patched nonmate.
The task of the XFR algorithm is to generate a network attention map that best indicates which regions in the probe image are matched to the paired image, rather than the unmatched regions that are inpainted for each triplet. This provides a basis for quantifying which image regions contribute to face matching.
Finally, we provide a comprehensive benchmark on this dataset, comparing five state-of-the-art algorithms on three face matchers. This benchmark includes two new algorithms, called Subtree EBP and Density-based Input Sampling Explanation (DISE), which significantly outperform the existing state-of-the-art techniques.
We also show qualitative visualizations of these network attention techniques on novel images and explore how these explainable face recognition models can improve transparency and trust in face matchers.
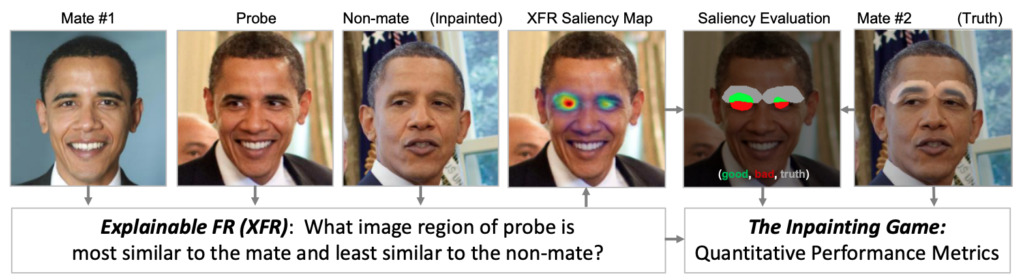
Age estimation 《Life span to age conversion synthesis》
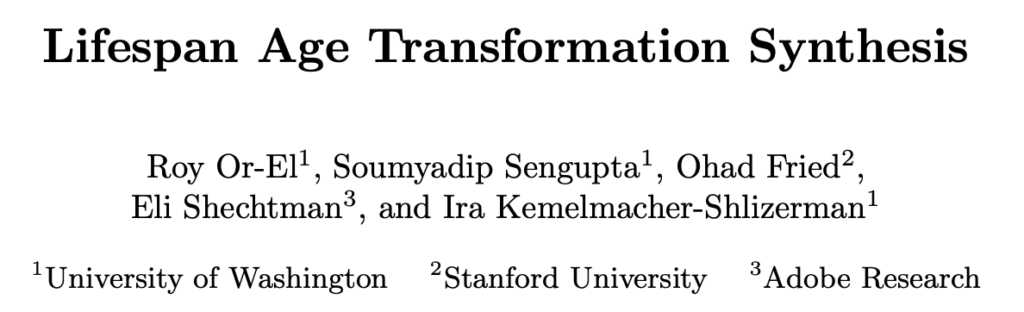
unit:University of Washington, Stanford University, Adobe Research
summary:
We solve the problem of age progression and regression for a single photo - predicting what a person will look like in the future or in the past.
Most existing aging methods are limited to changing textures, ignoring the changes in head shape during human aging and growth. This limits the applicability of previous methods to slightly older adults, and the application of these methods to photos of children does not produce high-quality results.
We propose a novel multi-domain image-to-image generative adversarial network architecture whose learned latent space models a continuous bidirectional aging process.The network is trained on the FFHQ dataset, which we label according to age, gender, and semantic segmentation. Fixed age classes are used as anchors to approximate continuous age transformations.Our framework can predict complete head portraits ranging from 0 to 70 years old based on only one photo, and modify the texture and head shape.We present results on a wide variety of photos and datasets, and show significant improvements over the state of the art.
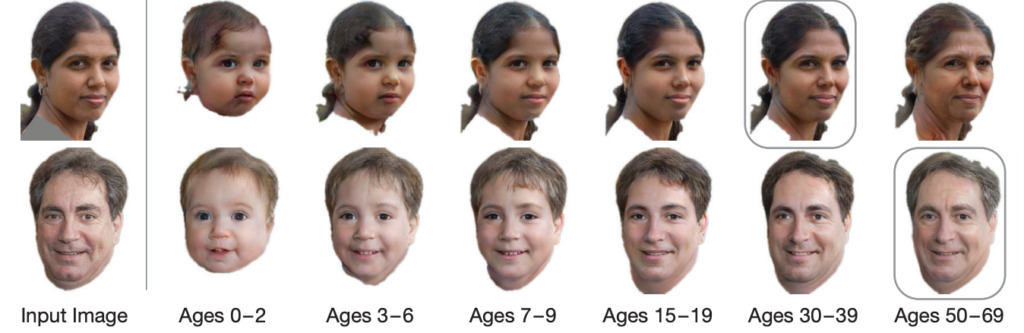
Portal: Papers, codes, all within one click
The above is just the tip of the iceberg of the thousands of selected papers for ECCV 2020. However, faced with a massive amount of 1,361 papers, it is really not an easy task to find the papers you are interested in, as well as the original links, codes, etc.
However, a Paper Digest Team The team has paved the way for readers, and finding papers and codes is no longer a problem.
The team recently published a summary of the ECCV 2020 paper highlights in one sentence.Each paper was summarized in one sentence, which was concise and to the point, and the paper address was attached.Allow readers to quickly find the papers they want to read most.
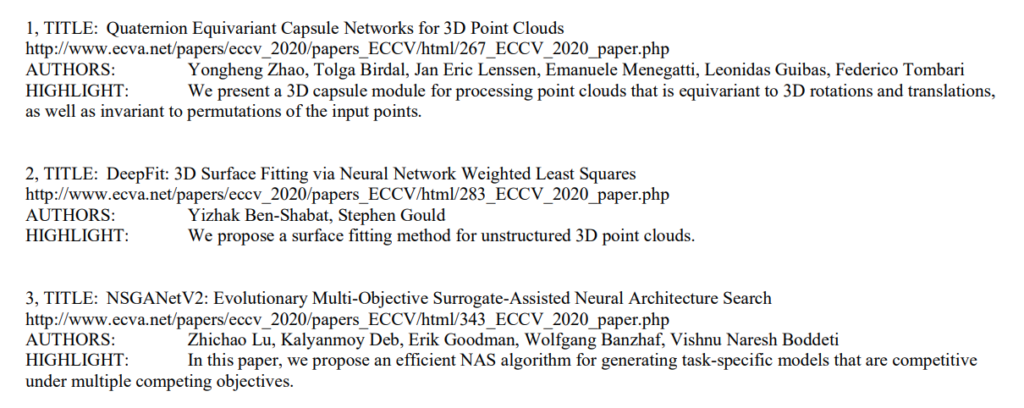
The address is available for you to take:
In addition, they have also thoughtfully compiled 170 papers that have published codes. Readers can directly click on the corresponding link to view the code:
In addition, crossminds.ai also compiled the presentation of the oral paper, and readers can understand the technology in the paper more clearly and intuitively through its demo demonstration, which is very interesting:
https://crossminds.ai/category/eccv%202020/
-- over--