World's First, Industry First: Wuhan University Opens Source Mask Face Recognition Dataset
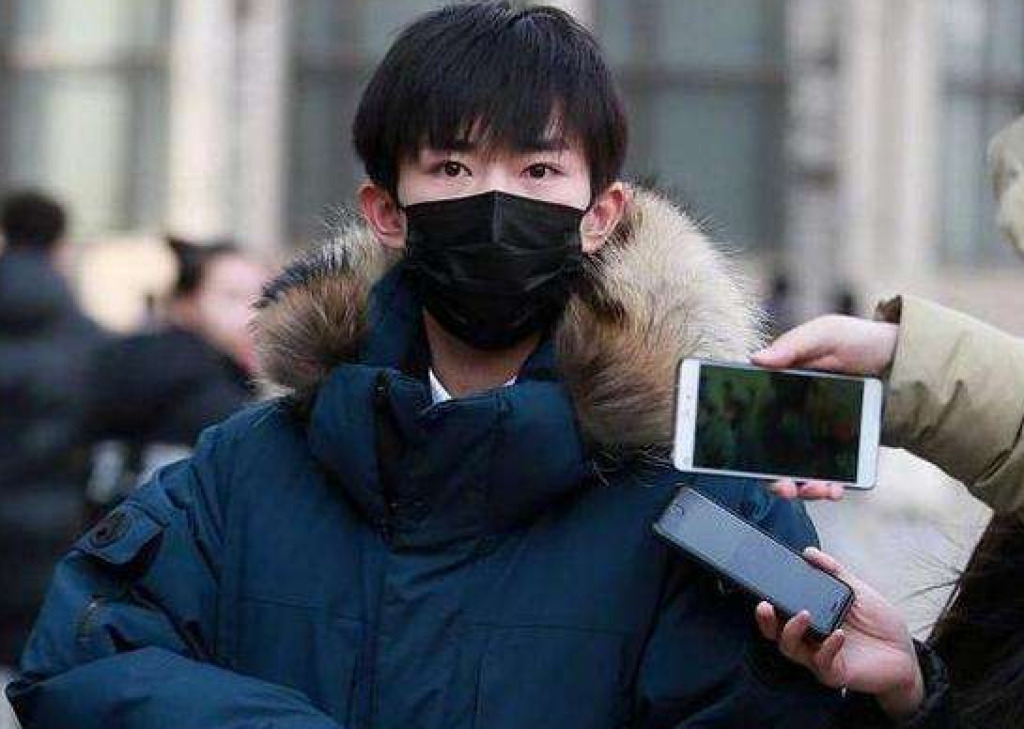
Wuhan University has made available for free the world's first mask-obstructed face dataset, including nearly 100,000 images of real people wearing masks and normal faces, as well as 500,000 simulated images of people wearing masks.
During this special period of fighting against the new coronavirus pneumonia, teachers and students of Wuhan University have not slowed down the pace of scientific research.
In early March, the National Multimedia Software Technology Research Center of Wuhan University opened a special face recognition dataset:Mask occluded face dataset:Real-World Masked Face Dataset, abbreviated as RMFD.
The world's first real mask face dataset
During the COVID-19 epidemic, almost everyone wore a mask, which made previous facial recognition technology basically ineffective.Facial recognition technology that can detect facial expressions hidden by masks has become an urgent need during the epidemic.
On March 8, Professor Wang Zhongyuan of the National Multimedia Software Engineering Technology Research Center of Wuhan University led a team to promptly launch emergency research on mask face recognition.
It is reported that Professor Wang Zhongyuan led a team of more than ten graduate students including Huang Baojin, Hong Qi, and Wu Hao to initially collect 360,000 facial data and developed semi-automatic auxiliary production tools such as data cleaning and labeling.

Dataset ①: 5000 real mask face dataset
In addition to simulating mask face datasets,The team also built the world's first public real mask face recognition sample set RMFD, which includes 5,000 mask faces of 525 people and 90,000 normal faces.

Datasets ② and ③: 500,000 simulated mask face datasets (including WebFace simulation and LFW simulation)
At the same time, in order to expand data diversity, the team developed a precise mask wearing software program.By putting masks on the faces of people in the public dataset, we constructed a simulated mask face dataset of 10,000 people and 500,000 faces.
The mask face recognition sample set must contain multiple face images of the same person with and without masks, which is difficult to construct.
Therefore, in response to the long production cycle of mask face sample sets, the team developed a four-step iterative R&D technical route and formulated four sets of R&D plans, so that timely adjustments and selection can be made based on the sample set situation and model performance.

At present, the real mask face recognition datasets and the simulated mask face recognition datasets have been opened to the public free of charge. The simulated mask face recognition datasets include WebFace and LFW simulated mask face dataset.
Based on the dataset they established, the team developed a face-eyebrow multi-granularity mask occlusion face recognition model.Achieved 95% accuracy on the dataset.
Datasets: Contributions are welcome
In addition, in order to further expand the data set, the team welcomes everyone to send their personal collection of mask-wearing pictures to x_zhangyang@whu.edu.cn, and will process the received pictures in a unified manner.
Now that we have the dataset, how do we download and use it?
How to download?
Mask face recognition dataset_Open source download address:
https://github.com/X-zhangyang/Real-World-Masked-Face-Dataset
How to use?
During the epidemic, how can we continue machine learning tasks when we cannot obtain computing power from schools and companies?
The partner we are going to introduce this time is OpenBayes, a cloud service that provides cloud computing power for machine learning. They have a large-scale supercomputing cluster, and the GPU cluster architecture is designed specifically for matrix computing. They provide computing power containers for AI applications, and they are very easy to use and can be used out of the box. Currently, OpenBayes' computing power container products already support TensorFlow, PyTorch, MXNet and other CPU and GPU environments, different versions and types of standard machine learning frameworks and various common dependencies.
Currently, the OpenBayes computing container supports standard librariesand provide CPU, NVIDIA T4, NVIDIA Tesla V100 and other computing resourcesWhether it is centralized training of massive data or low-power model resident operation, it can easily meet user needs.
From CPU to T4 to V100, a wide range of computing container configurations OpenBayes supportScript upload and JupyterLab editorOnline programming and then model training.
Clear and concise execution processFull tutorial: https://openbayes.com/docs/quickstart/
Register as a new user to enjoy GPU computing power!
Visit openbayes.com, click on the official website to register immediately, and there will be free gifts every week during the internal test period, so you don’t have to compete with classmates and colleagues for computing power~
The dataset can be used/downloaded directly from public resources Activity Description Visit openbayes.com Register as a new user with the invitation code [HyperAI]You can enjoy600 minutes of CPU + 300 minutes of NVIDIA T4 per week Free computing power~
-- over--