MV-MATH Mathematical Reasoning Annotation Dataset
Date
Size
Publish URL
License
MIT
Categories
MV-MATH is a multimodal mathematical reasoning benchmark dataset proposed by the Institute of Automation, Chinese Academy of Sciences in 2025. It aims to comprehensively evaluate the mathematical reasoning ability of multimodal large language models (MLLMs) in multi-visual scenes.MV-MATH: Evaluating Multimodal Math Reasoning in Multi-Visual Contexts", has been accepted by CVPR 2025.
The MV-MATH dataset contains 2,009 high-quality math problems, and the types of questions are divided into three categories: multiple-choice questions, fill-in-the-blank questions, and multi-step questions. The dataset contains multiple visual scenes, and each question is accompanied by 2 to 8 images. These images are intertwined with text to form complex multi-visual scenes, which are closer to mathematical problems in the real world and can effectively evaluate the model's reasoning ability to process multi-visual information. Secondly, the dataset is very rich in annotations. Each sample has been cross-validated by at least two annotators. The annotations cover questions, answers, detailed analysis, and image correlation, providing detailed information for model evaluation. In addition, the dataset covers 11 mathematical fields from basic arithmetic to advanced geometry, including analytic geometry, algebra, metric geometry, combinatorics, transformation geometry, logic, solid geometry, arithmetic, combinatorial geometry, descriptive geometry, and statistics. According to the length of the detailed answers, the dataset is also divided into 3 difficulty levels, which can comprehensively evaluate the model's reasoning ability in different mathematical fields. It is worth mentioning that this dataset introduces the feature label of image correlation for the first time, dividing the dataset into two subsets: Mutually Dependent Set (MD) and Independent Set (ID). In the MD subset, images are interrelated, and understanding an image requires reference to other images; while in the ID subset, images are independent and can be interpreted separately.
It not only originates from real K-12 education scenarios and can be used to develop intelligent tutoring systems to help students solve complex math problems by combining text and images, but also provides standardized evaluation tools for multimodal learning research, helping researchers identify and improve the performance gaps of models in mathematical reasoning. However, in tests of mainstream multimodal large language models, such as GPT-4o and QvQ, their scores on the MV-MATH dataset were 32.1 and 29.3, respectively, both of which failed to reach the passing line, indicating that current multimodal large models still face major challenges in multi-visual mathematical reasoning tasks.
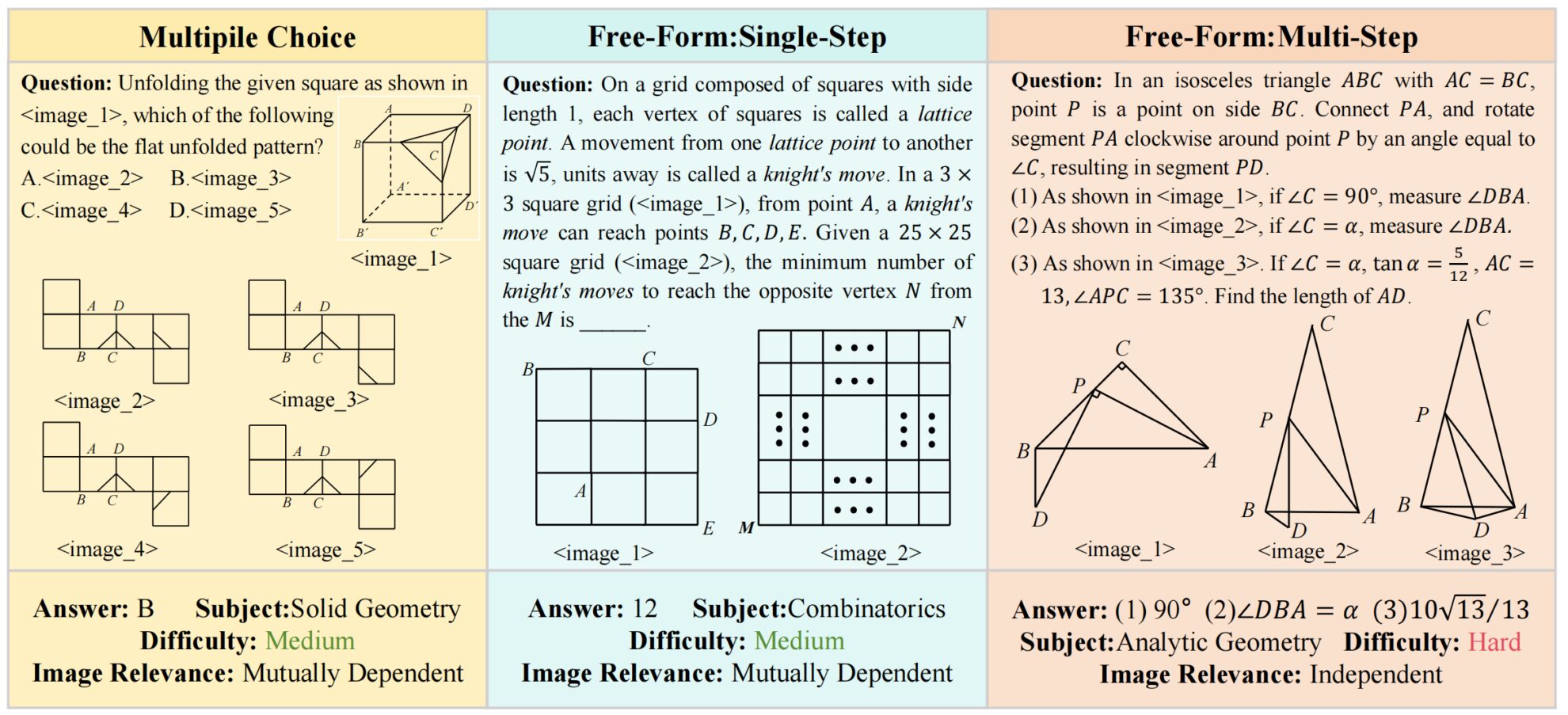