MINT-1T Text-Image Pair Multimodal Dataset
Date
Publish URL
The MINT-1T dataset is a multimodal dataset jointly open-sourced by Salesforce AI and multiple institutions in 2024. It has achieved significant expansion in scale, reaching one trillion text tags and 3.4 billion images, which is 10 times the size of the previous largest open-source dataset. The related paper results are "MINT-1T: Scaling Open-Source Multimodal Data by 10x: A Multimodal Dataset with One Trillion Tokens". The construction of this dataset follows the core principles of scale and diversity. It includes not only HTML documents, but also PDF documents and ArXiv papers. Such diversity significantly improves the coverage of scientific documents. MINT-1T's data sources are diverse, including but not limited to web pages, academic papers, and documents, which have not been fully utilized in multimodal datasets before.
In terms of model experiments, the XGen-MM multimodal model pre-trained on MINT-1T performed well in image description and visual question answering benchmarks, surpassing the previous leading dataset OBELICS. Through analysis, MINT-1T has significantly improved in scale, data source diversity and quality, especially in PDF and ArXiv documents, which have significantly longer average length and higher image density. In addition, the results of topic modeling of documents through the LDA model show that the HTML subset of MINT-1T shows a wider range of field coverage, while the PDF subset is mainly concentrated in the fields of science and technology.
MINT-1T has demonstrated excellent performance on multiple tasks, especially in science and technology, thanks to the popularity of these fields in ArXiv and PDF documents. The models trained on MINT-1T outperformed the baseline model OBELICS in all numbers of examples when evaluating the contextual learning performance of the models using different numbers of examples. The release of MINT-1T not only provides researchers and developers with a large multimodal dataset, but also provides new challenges and opportunities for the training and evaluation of multimodal models.
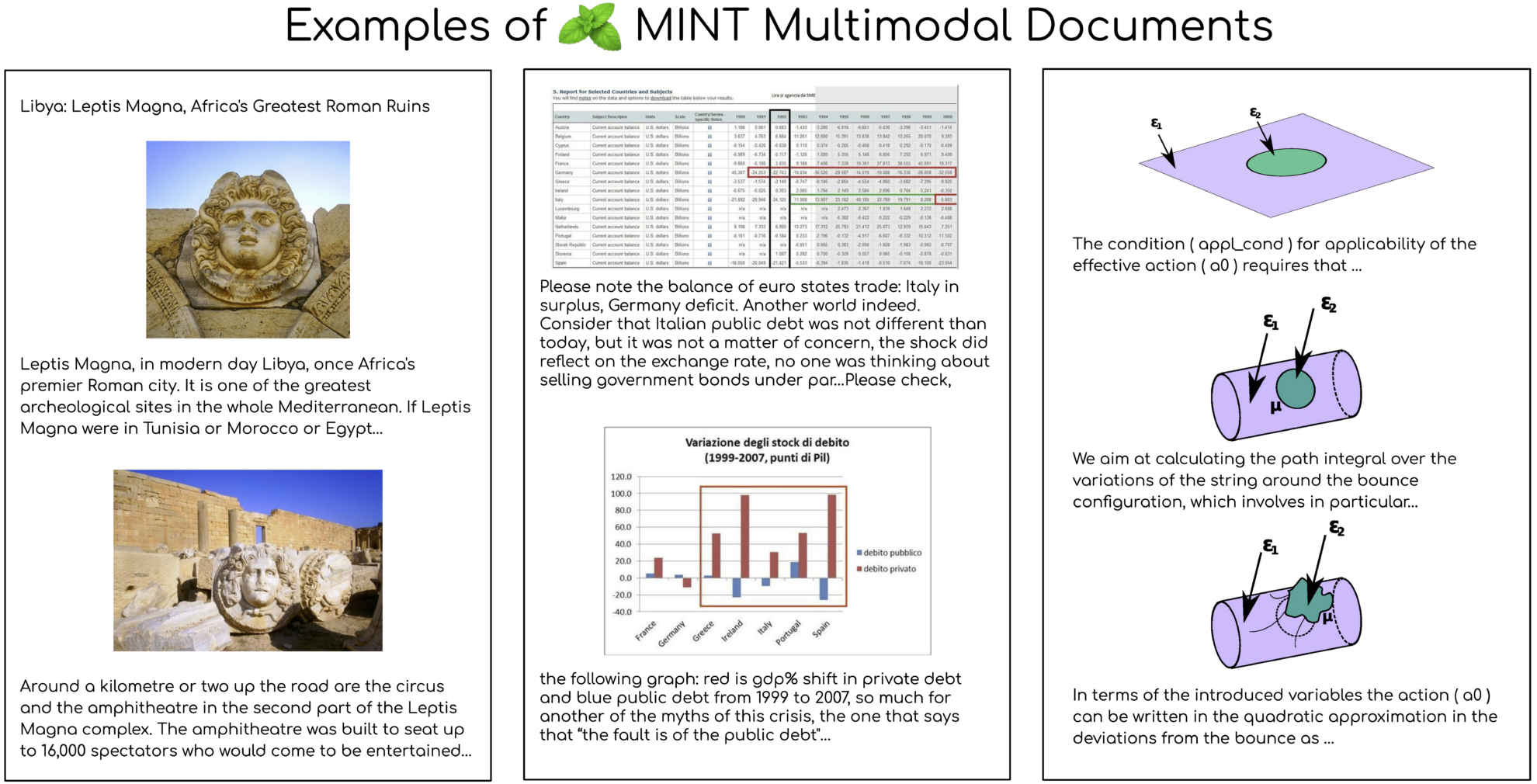